Decoding Bone Health: Can AI-Powered Image Analysis Revolutionize Osteoporosis Detection?
"Explore how cutting-edge image analysis techniques, fueled by artificial intelligence, are enhancing the precision and speed of osteoporosis diagnosis."
Osteoporosis, a condition characterized by decreased bone mass and increased fracture risk, affects millions worldwide, particularly postmenopausal women. Early and accurate diagnosis is crucial for effective management and prevention of severe consequences such as hip fractures. Traditional diagnostic methods, such as bone densitometry, have limitations, highlighting the need for innovative approaches.
The convergence of artificial intelligence (AI) and medical imaging is opening new frontiers in disease detection and management. AI-powered image analysis can enhance the precision and efficiency of diagnosing various conditions, including osteoporosis. By leveraging machine learning algorithms, subtle patterns and indicators within medical images can be detected, often missed by the human eye.
This article delves into how fractional Brownian motion (fBm), a mathematical concept used to describe natural phenomena, is being harnessed alongside AI to improve osteoporosis diagnosis. We'll explore how AI algorithms classify images generated by fBm, the effectiveness of these methods, and their potential to transform bone health assessments.
AI and Fractional Brownian Motion: A New Diagnostic Era
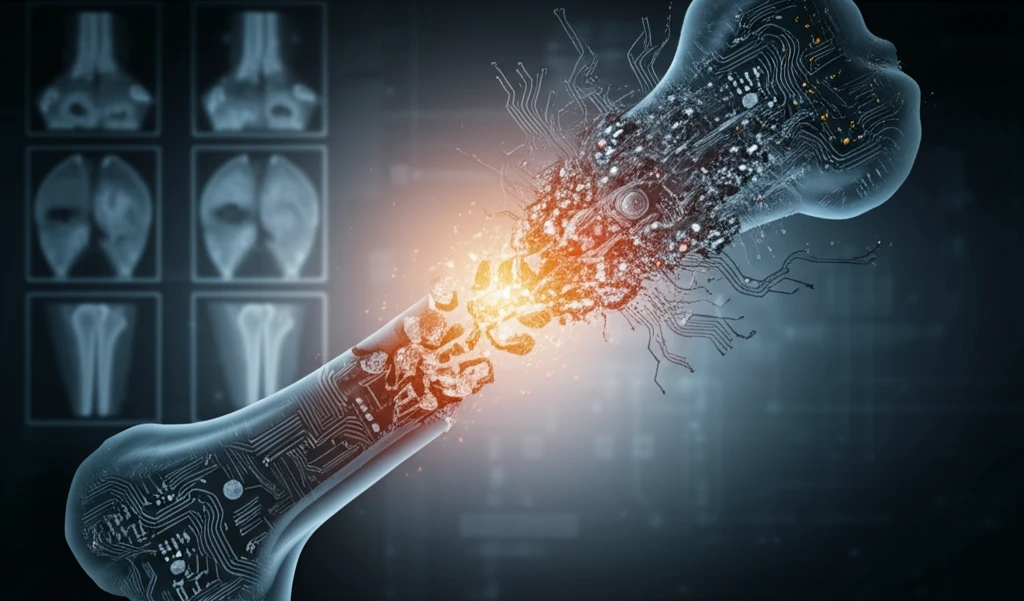
Fractional Brownian motion (fBm) is a mathematical concept used to characterize various natural phenomena, from landscapes to stock market fluctuations. In medical imaging, fBm helps model and analyze complex textures, such as those found in bone structures. By synthesizing images using fBm, researchers can create models that mimic the intricate patterns of bone tissue, allowing for better assessment of bone health.
- Dual-tree MBand Decomposition Transform (DMBDT): Extracts crucial texture features from the synthesized images, providing a detailed multi-scale analysis.
- Support Vector Machine (SVM) Classifier: Classifies the images based on the extracted features, evaluating the quality and accuracy of the fBm synthesis methods.
- Statistical Feature Analysis: Includes measures such as mean, variance, mode, and Renyi entropy to quantify the characteristics of bone texture.
The Future of Osteoporosis Diagnostics
The integration of AI and fractional Brownian motion analysis represents a significant leap forward in osteoporosis diagnostics. This innovative approach not only promises earlier and more accurate detection but also opens doors for personalized treatment strategies. As AI technology continues to evolve, we can anticipate even more sophisticated tools that will further transform bone health management and improve the quality of life for millions affected by osteoporosis.