Unlock Hidden Patterns: How AI is Revolutionizing Image Analysis
"Discover the power of unsupervised learning in hyperspectral imaging and how it's changing everything we see."
Imagine being able to see the world in more dimensions than ever before. Hyperspectral imaging (HSI) does just that, capturing images across a wide spectrum of light far beyond what the human eye can perceive. This technology has become increasingly important in fields ranging from environmental monitoring to medical diagnostics.
The challenge? These images contain so much data that traditional analysis methods struggle to keep up. That's where artificial intelligence (AI), and specifically a technique called feature extraction (FE), comes in. Feature extraction helps to simplify this complex data into something manageable and insightful.
However, a significant hurdle exists: most AI-driven FE methods require labeled data for training, and in the real world, labeled hyperspectral images are rare. This is where innovative AI solutions are stepping in to bridge the gap.
The AI Breakthrough: Learning Without Labels
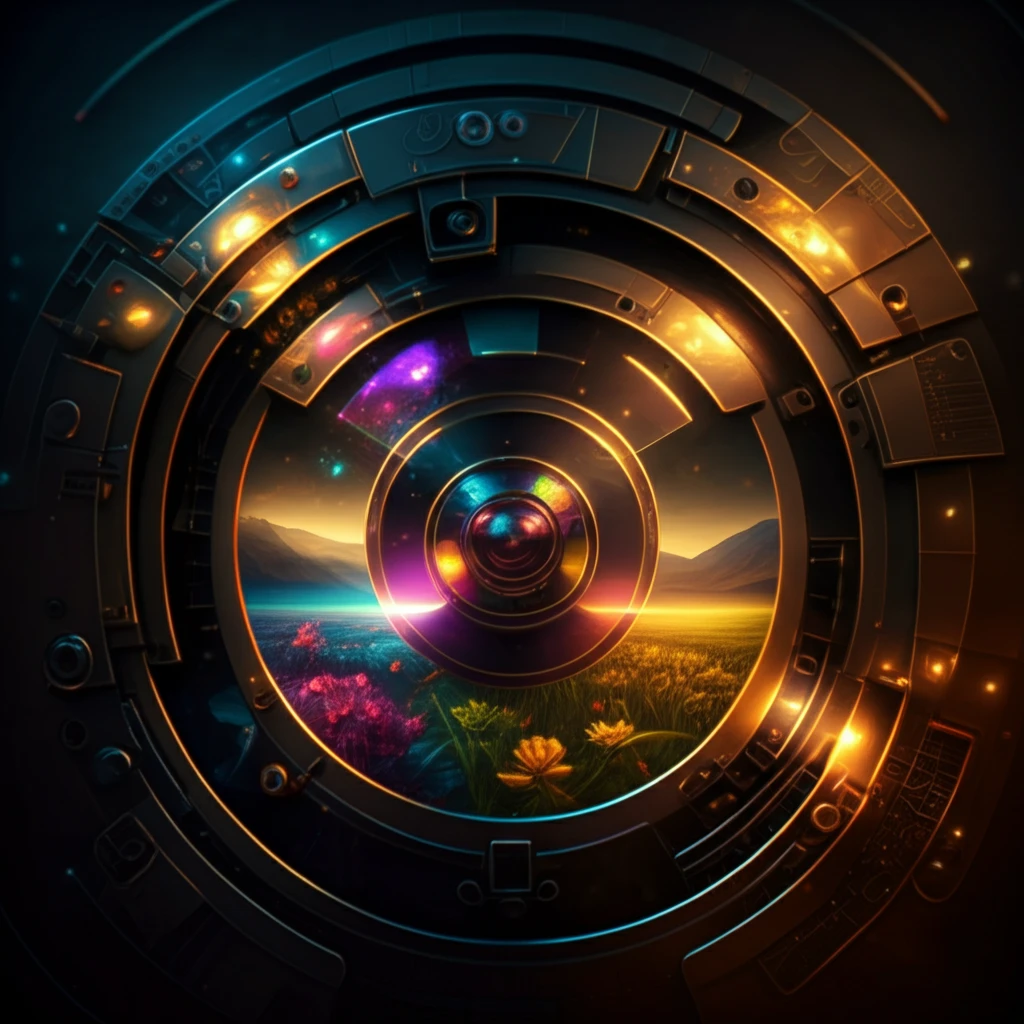
A groundbreaking study has introduced a new approach to feature extraction that doesn't rely on labeled data. This method uses a modified Generative Adversarial Network (GAN) – a type of AI that pits two neural networks against each other. One network, the generator, creates synthetic hyperspectral images, while the other, the discriminator, tries to distinguish between the synthetic and real images.
- Unsupervised Learning: Eliminates the need for labeled data, opening up new possibilities for analyzing previously inaccessible datasets.
- Adaptive Learning: The GAN framework adapts its learning strategy based on the characteristics of the data, leading to more effective feature extraction.
- Wasserstein Distance: Replaces traditional divergence metrics, ensuring more stable and reliable training of the GAN.
- All Convolutional Nets: Generator and Discriminator are designed based on a fully deconvolutional subnetwork and a fully convolutional subnetwork, respectively.
The Future of Seeing the Unseen
This new AI-driven approach represents a major step forward in hyperspectral image analysis. By eliminating the need for labeled data, it unlocks the potential to analyze vast amounts of previously inaccessible information. As AI continues to evolve, we can expect even more sophisticated tools to emerge, transforming how we understand our world through advanced imaging technologies. This has huge implications for environment monitoring, agriculture, urban development, and medical imaging.