Straighten Out Your Drive: How AI is Remapping Road Safety
"Discover how cutting-edge lane detection and road surface reconstruction technology uses multiple vanishing points to make self-driving cars safer and more reliable."
Navigating the world, whether on foot or behind the wheel, relies heavily on our ability to perceive our surroundings accurately. For self-driving cars, this perception is even more critical. They need to 'see' and understand the road ahead, identifying lanes, obstacles, and potential hazards in real-time. Current lane detection systems often rely on the assumption of a flat road, which, as anyone who's driven up a steep hill knows, isn't always the case.
Imagine a self-driving car approaching a slope. If the system assumes a flat road, it might misinterpret the lane markings, leading to navigation errors or, worse, accidents. This is where advanced research in lane detection and road surface reconstruction comes in. By incorporating multiple 'vanishing points'—points in the distance where parallel lines appear to converge—these systems can create a more accurate 3D model of the road, even on slopes and uneven surfaces.
This article will explore how a pioneering approach to lane detection is tackling this challenge, promising safer and more reliable autonomous navigation. It dives into the technical details while making the concepts accessible to everyone.
The Science of Seeing: Multiple Vanishing Points
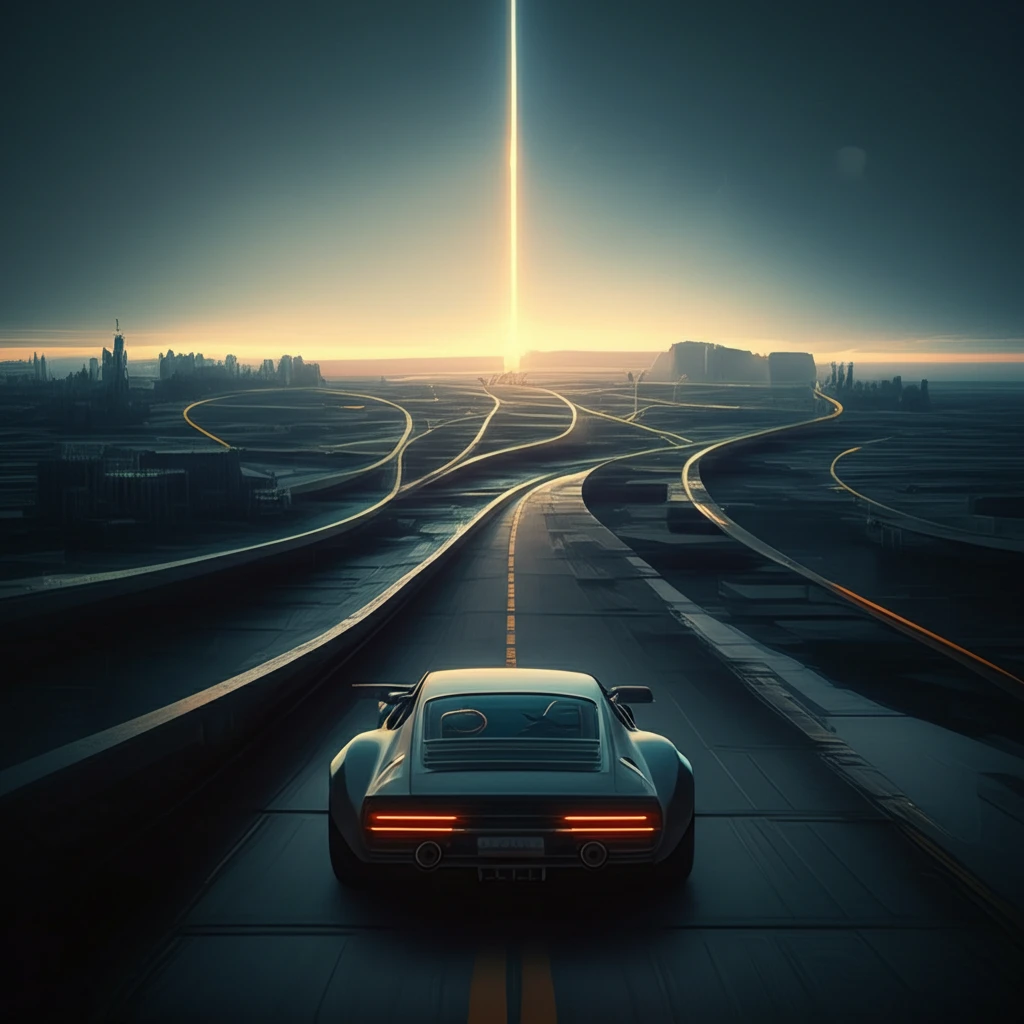
The core of this innovative system lies in its ability to identify and utilize multiple vanishing points in an image. Think of standing on a long, straight road: the edges of the road appear to meet at a single point in the distance. That's a vanishing point. On a flat road, a single vanishing point might suffice. However, on a sloped or curved road, multiple vanishing points are needed to accurately represent the 3D structure.
- Accounts for changes in road slope and curvature
- Enhances accuracy in challenging road conditions
- Divides images into 'near' and 'far' regions for better analysis
- Optimizes lane marker selection for accurate interpretation
The Road Ahead: Safer, Smarter Driving
The research marks a significant step forward in making self-driving cars a safer and more dependable reality. By using multiple vanishing points to build a more accurate picture of the road, these systems can better handle the complexities of real-world driving conditions. As AI and machine learning continue to evolve, we can anticipate even more sophisticated lane detection and road reconstruction technologies. These advancements promise to enhance the safety and efficiency of autonomous vehicles, making our roads safer for everyone.