Smarter Forecasting: How AI and Data Combine to Predict Energy Prices
"Explore how the innovative LASSO-PCA model enhances energy price forecasting, reduces reliance on guesswork, and promises more stable energy markets."
Predicting electricity prices is a tricky business, but it's also super important. Energy markets need accurate forecasts to make smart decisions about everything from daily operations to long-term investments. With renewable energy sources becoming more common, and energy markets facing increasing instability, the need for reliable forecasting methods has never been greater.
Traditionally, experts have used various models and historical data to predict future energy prices. However, these methods often involve a lot of manual adjustments and educated guesses, leading to uncertainty and potential errors. What if we could use AI to automate and improve this process, making energy price predictions more accurate and less dependent on human intervention?
That's where the LASSO-PCA model comes in. This innovative approach combines two powerful techniques—LASSO and Principal Component Analysis (PCA)—to create a fully automated forecasting system. By harnessing the power of AI and data, LASSO-PCA promises to reduce errors, increase efficiency, and ultimately bring more stability to energy markets.
Why Traditional Energy Forecasting Is So Problematic
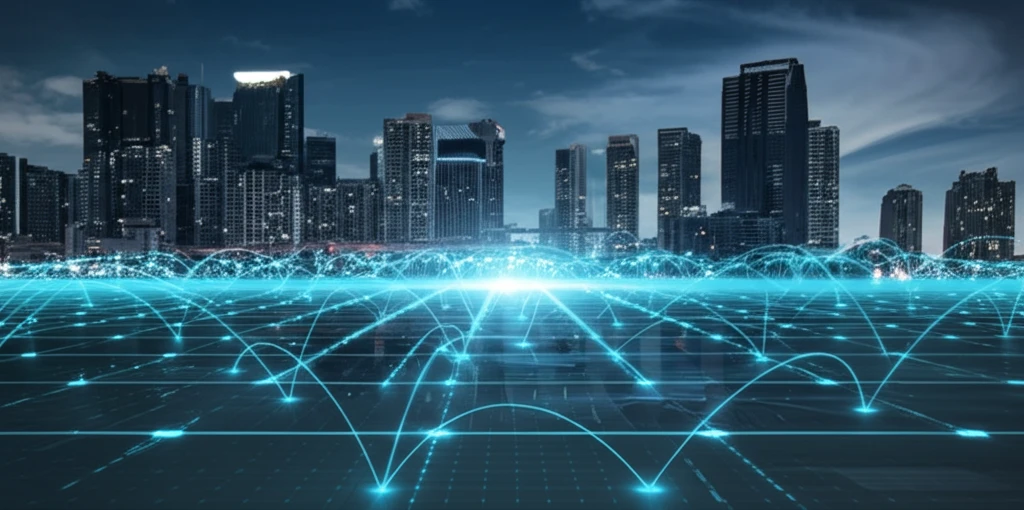
Traditional energy forecasting methods often rely on a mix of statistical models and expert judgment. While these approaches can be effective to some extent, they also have several limitations. One major issue is the reliance on historical data, which may not accurately reflect current market conditions. For example, the increasing prevalence of renewable energy sources like solar and wind power has introduced new levels of uncertainty and variability into energy markets.
- Reliance on Historical Data: Fails to capture new market dynamics.
- Manual Adjustments: Introduces bias and increases error potential.
- Inability to Adapt Quickly: Struggles with sudden market shifts.
The Future of Energy Forecasting: AI-Driven and Automated
The LASSO-PCA model represents a significant step forward in the field of energy price forecasting. By combining the strengths of LASSO and PCA techniques, this approach offers a fully automated, data-driven solution that reduces errors, increases efficiency, and adapts to changing market conditions. As AI continues to evolve, we can expect even more sophisticated forecasting models to emerge, further transforming the energy industry and paving the way for a more stable and sustainable future.