Early Detection of Liver Cancer: A Guide to Advanced Imaging and AI
"Discover how cutting-edge AI-enhanced CT scans are revolutionizing liver cancer detection, offering hope and improving outcomes."
The liver is a vital organ responsible for numerous functions, including protein synthesis and biochemical production essential for digestion. As the only liver you have, its strategic location in the abdominal cavity makes it prone to various diseases. Early detection of liver cancer significantly improves life expectancy and informs treatment strategies.
Traditional methods of liver tumor detection, such as manual segmentation, are time-consuming and can be subjective. To address these limitations, researchers are developing advanced, automated techniques that promise more efficient and accurate diagnoses.
This article explores an innovative method employing Fuzzy C-Means (FCM), Neutrosophic sets (NS), and Bayesian Neural Networks (BNN) to detect cancerous cells in the liver. This approach leverages abdominal CT scans to extract and analyze liver images, providing a more reliable and timely diagnosis.
How AI is Revolutionizing Liver Cancer Detection
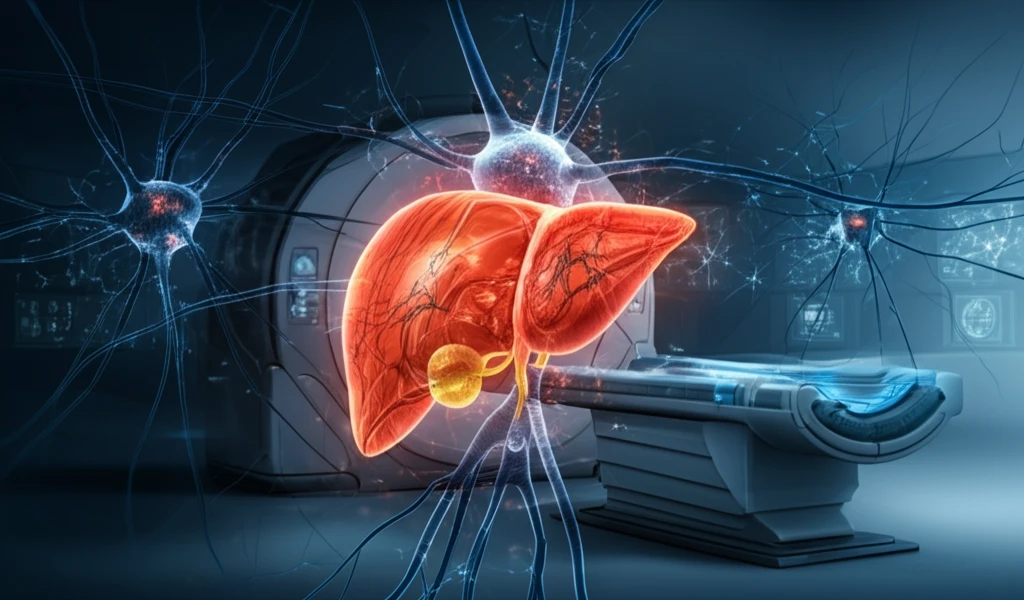
The core of this innovative approach lies in its methodology, which integrates several advanced techniques to segment and classify tumors in CT images of the liver. The process involves:
- Image Pre-processing: Enhances the quality of CT scan images to reduce noise and improve contrast, ensuring clearer and more defined images for subsequent analysis.
- Image Segmentation: Employs the Fuzzy C-Means (FCM) algorithm combined with Neutrosophic Sets (NS) to accurately delineate the liver and potential tumors from the surrounding tissues. FCM is particularly useful as it allows data points to belong to multiple clusters, reflecting the inherent ambiguity in medical images.
- Feature Extraction: Identifies and extracts key characteristics from the segmented liver images. This includes textural features (assessing the arrangement of pixels) and statistical measures such as entropy, mean, and standard deviation, which help differentiate between healthy and cancerous tissue.
- Classification: Uses a Bayesian Neural Network (BNN) to classify the extracted features and predict whether the cells are cancerous. BNNs are adept at handling uncertainty and can provide probabilistic assessments, offering a more nuanced diagnosis.
The Future of Liver Cancer Diagnostics
The AI-enhanced method demonstrates promising results, achieving an accuracy of 97.33%, sensitivity of 98.03%, and specificity of 97.26%. These metrics indicate the method's high reliability in correctly identifying both cancerous and healthy tissues. With a short time delay of just 3.04 seconds, it also provides a fast diagnostic option. This is a valuable second opinion for doctors and improves patients peace of mind for the accurate diagnosis of their condition at the earliest stages.