Decoding Seizures: How AI and Brainwave Analysis Are Changing Epilepsy Detection
"AI-powered EEG analysis offers a transformative approach to detecting epileptic seizures, providing new hope for faster and more accurate diagnoses."
Epilepsy, a chronic neurological disorder characterized by recurrent seizures, affects millions worldwide. These seizures, resulting from abnormal electrical activity in the brain, can significantly impair a person's quality of life, impacting everything from their physical safety to their cognitive functions. The unpredictable nature of seizures makes diagnosis and management particularly challenging.
Traditional methods of diagnosing epilepsy rely heavily on long-term electroencephalogram (EEG) recordings, which capture brainwave patterns over extended periods. However, visually analyzing these recordings is a time-consuming and labor-intensive process, often subject to human error. Neurologists must meticulously review hours of EEG data to identify subtle abnormalities indicative of seizure activity.
In recent years, the application of artificial intelligence (AI) to EEG analysis has emerged as a promising solution to overcome these limitations. AI algorithms, particularly those based on neural networks, can be trained to recognize complex patterns in EEG data with remarkable accuracy and speed. This opens the door to automated seizure detection systems that can assist neurologists in making faster and more reliable diagnoses.
The AI Revolution in EEG Analysis
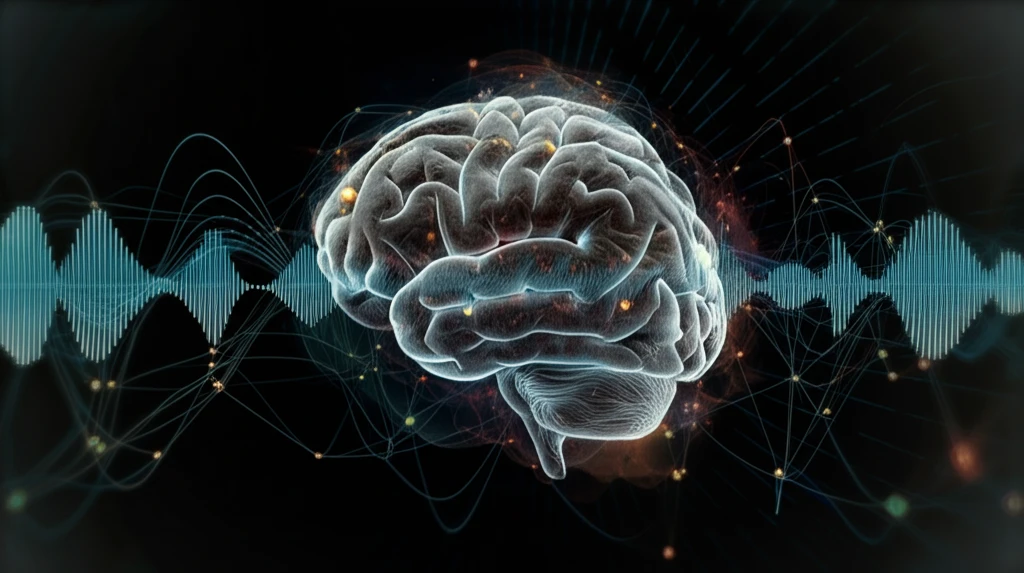
One particularly promising approach involves the use of Teager energy, a nonlinear measure that is sensitive to both the amplitude and frequency changes in EEG signals. Seizures are often characterized by rapid fluctuations in brainwave activity, making Teager energy an ideal feature for detecting these events. When combined with backpropagation neural networks, Teager energy analysis can achieve high levels of accuracy in distinguishing between normal brain activity and seizure patterns.
- EEG data pre-processing to remove noise and artifacts.
- Extraction of Teager energy features from the EEG signals.
- Training a backpropagation neural network to classify EEG segments as either normal or epileptic.
- Evaluating the performance of the classifier using metrics such as sensitivity, specificity, and false detection rate.
The Future of Epilepsy Diagnosis
The application of AI to EEG analysis represents a significant step forward in the diagnosis and management of epilepsy. By automating the detection of seizure events and inter-ictal abnormalities, AI-powered systems can reduce the burden on neurologists, improve diagnostic accuracy, and enable earlier intervention for patients with epilepsy. As AI technology continues to evolve, we can expect even more sophisticated and effective tools to emerge, further transforming the landscape of epilepsy care. This could lead to more personalized treatment plans, better seizure control, and ultimately, improved quality of life for individuals living with epilepsy.