Decoding Lung Cancer: How Digital Tools and Minor Solid Patterns Impact Prognosis
"New research reveals how digital pathology can enhance cancer diagnosis and why even small amounts of solid patterns in lung tumors matter for predicting patient outcomes."
Lung cancer remains a leading cause of cancer-related deaths worldwide, prompting continuous research efforts to improve diagnostic accuracy, treatment strategies, and prognostic predictions. Traditional methods of assessing tissue samples often rely on subjective evaluations, which can be enhanced with modern technologies.
Two recent studies highlight significant advancements in lung cancer research. The first explores the utility of digital pathology in complementing subjective assessments, particularly in measuring PD-L1 expression. The second investigates the impact of minor components of solid patterns in pathological stage I lung adenocarcinoma, revealing their significant prognostic value.
These studies emphasize the importance of integrating innovative technologies and refining pathological assessments to provide more precise and personalized approaches to lung cancer management. By understanding these advancements, individuals can better navigate the complexities of lung cancer diagnosis and treatment.
Digital Pathology: Enhancing Traditional Assessments
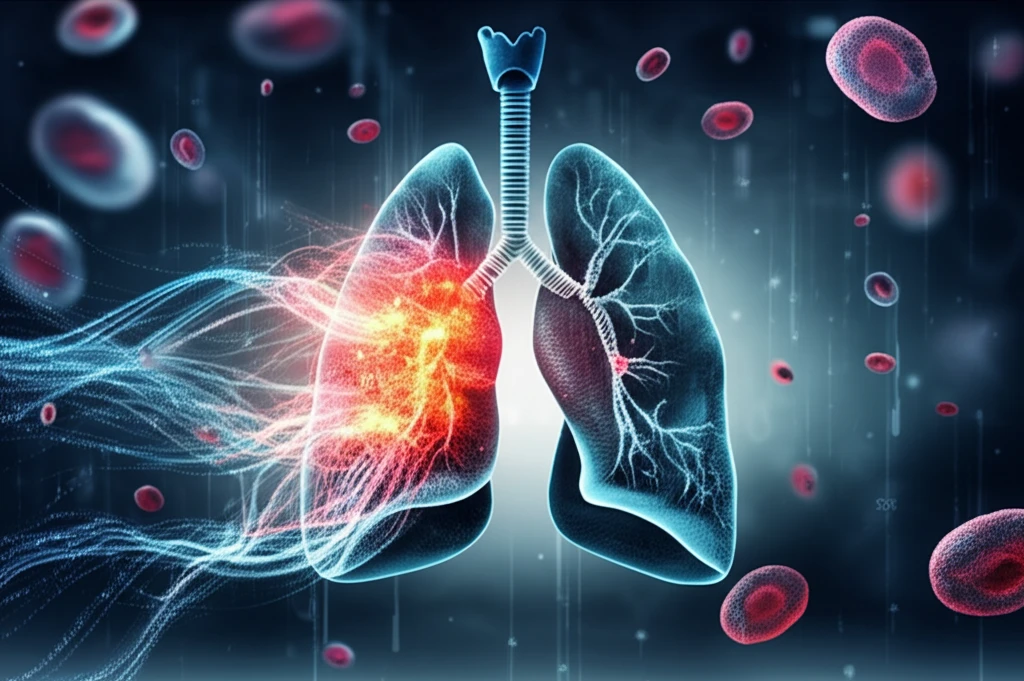
Traditional pathological assessment of tissue sections involves subjective analysis, which can be challenging, especially when 'measuring' PD-L1 expression in non-small cell lung cancer (NSCLC). PD-L1 expression guides the use of immune checkpoint inhibitors, making accurate measurement crucial. Heterogeneity in tumors, once seen as a problem, might reveal essential information about tumor behavior and the microenvironment.
- Scanning slides to create digital images using Aperio Scanscope.
- Dividing images into 1mm² squares using QuPath opensource software.
- Subjective assessment of squares by pathologists for morphological features and PD-L1 expression.
- Automatic image analysis, including cell counting and membrane detection.
The Prognostic Impact of Minor Solid Patterns
These advancements offer new hope for improving lung cancer outcomes through more accurate diagnosis and personalized treatment strategies. By staying informed and proactive, individuals can work with healthcare providers to leverage these cutting-edge approaches for better health management.