Decoding Bias: Can AI Really Make Fairer Lending Decisions?
"Explore how AI models inherit and amplify biases in mortgage lending, and discover innovative de-biasing methods that could revolutionize financial fairness."
In an era increasingly shaped by algorithms, the promise of artificial intelligence (AI) to automate and streamline decision-making processes is both exciting and fraught with challenges. One area where AI is making significant inroads is in the financial sector, particularly in mortgage lending. The appeal is clear: AI can process vast amounts of data quickly, potentially leading to faster and more efficient loan approvals. However, this automation raises a critical question: Can AI truly make unbiased decisions, or does it simply perpetuate existing societal inequalities?
The challenge lies in the data used to train these AI models. If the historical data reflects biased lending practices, the AI will inevitably learn and replicate these biases, even if protected characteristics like race or ethnicity are explicitly excluded from the model. This can lead to a situation where AI, intended to be a neutral arbiter, ends up reinforcing discriminatory patterns in lending.
A recent study delves into this issue, exploring various methods to de-bias AI models used in mortgage application approvals. The research investigates how AI models inherit biases, even when seemingly objective criteria are used, and compares different techniques to mitigate these biases, offering insights into the potential and limitations of AI in promoting fairer lending practices.
The Ghost in the Machine: How AI Learns to Discriminate
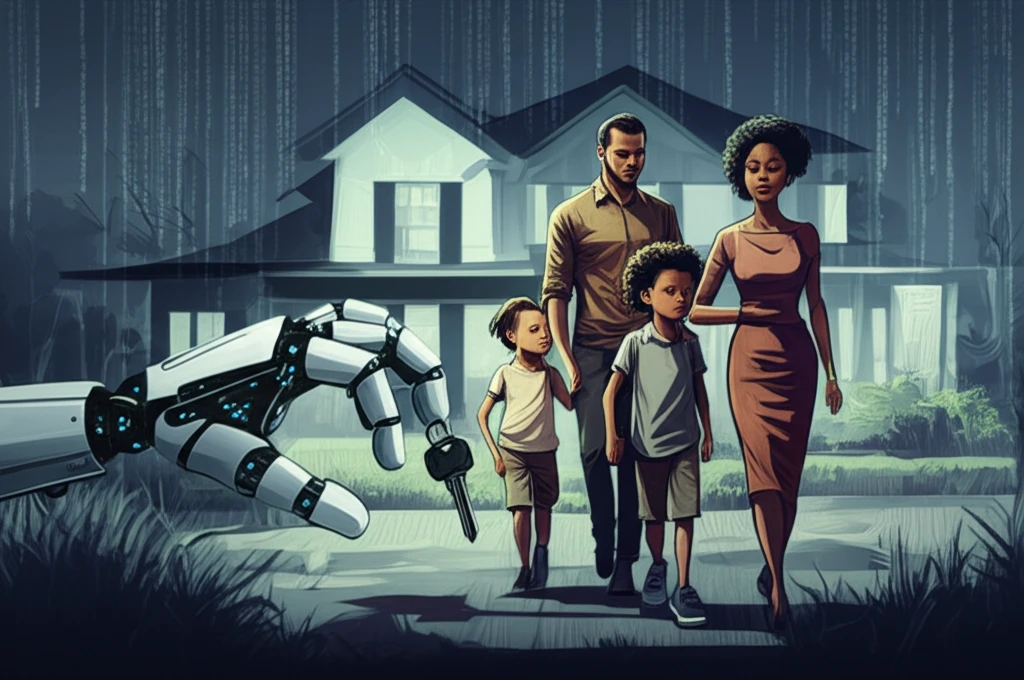
The study begins by demonstrating how easily an AI model can replicate bias, even without explicitly using protected characteristics. Researchers simulated bias against Hispanic and Latino applicants by artificially altering approval decisions in a real-world mortgage dataset. This manipulation ensured that the AI model would encounter biased data during its training phase.
- Correlation Exploitation: AI can identify correlations between seemingly neutral variables and protected characteristics, using these as proxies for discrimination.
- Data Reflection: If historical data is biased, AI models trained on that data will inevitably learn and replicate those biases.
- Subtle Patterns: AI can uncover and amplify subtle discriminatory patterns that humans might miss.
The Path to Fairer Algorithms
The journey toward truly fair AI in mortgage lending is ongoing. The findings underscore the importance of contextual awareness and careful consideration of the different forms that bias can take. By implementing appropriate de-biasing techniques and continuously monitoring AI models for discriminatory outcomes, the industry can move closer to a future where technology promotes, rather than hinders, equal access to financial opportunities. The key is to understand the nuances of how AI learns and to proactively address the potential for bias at every stage of the development and deployment process.