Data Annotation Revolution: How Smart Rules and Fair Pay Boost AI Training
"Unlock the secrets to high-quality AI: Discover how optimized task instructions and ethical compensation are transforming data annotation for better models."
Artificial Intelligence is reshaping industries at a rapid pace. Underlying the advancements is data, specifically the labeled data that AI models learn from. This critical process, known as data annotation, involves humans labeling images, text, and other forms of data, and it's more complex than one might think. But what truly impacts the quality of data annotation, and how can we optimize it for better AI?
The global data annotation market is a booming sector, valued at billions of dollars, and is expected to continue growing exponentially. However, along with its growth, questions arise about the ethical considerations and working conditions of data annotators. The core issue revolves around ensuring high-quality data annotation while maintaining fair labor practices. What drives annotators to perform their best, and what strategies are most cost-effective in achieving top-tier results?
Recent research has begun to dissect the variables affecting data annotation quality, with a particular focus on task instruction design and monetary incentives. A groundbreaking study sheds light on how clear rules and fair pay not only improve performance but also enhance the well-being of annotators. By bridging economics, ethics, and technology, this research highlights the path toward more reliable and ethical AI development.
The Power of Clarity: How Rules Outperform Standards in Data Annotation
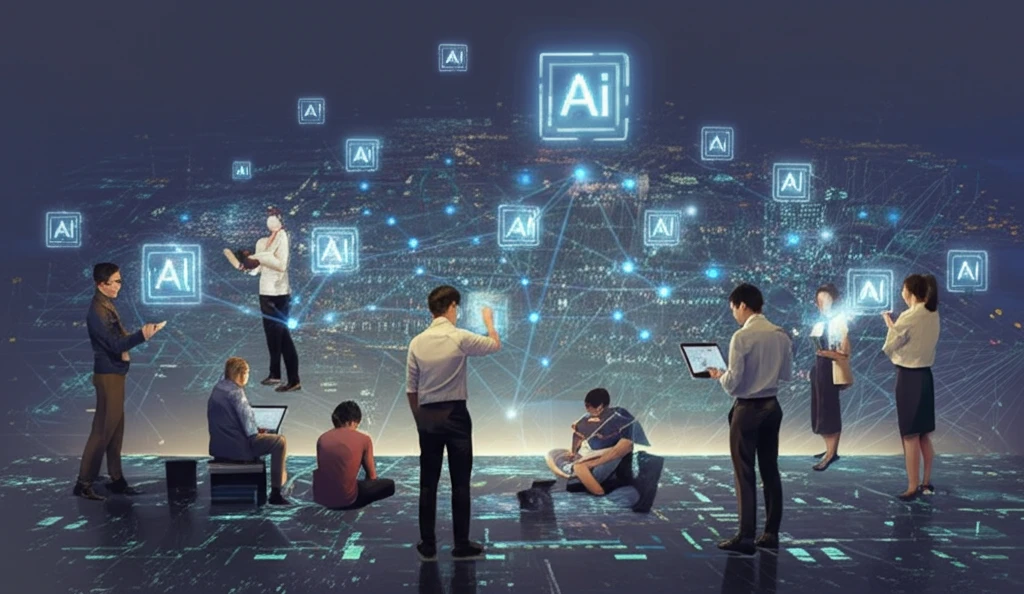
Traditional approaches to data annotation often involve either vague standards or detailed rules. The study examined these approaches, pitting clear, explicit rules against more open-ended standards. The findings? Annotators provided with clear rules demonstrated a 14% higher accuracy rate than those guided by standards. When instructions are specific, annotators make fewer errors, leading to cleaner, more reliable datasets.
- Clarity Matters: Explicit rules reduce ambiguity and improve consistency.
- Comprehensive Instructions: Complete rules set the standard for high-quality annotation.
- Reduced Errors: Clear guidelines minimize mistakes, benefiting overall AI model performance.
The Future of AI Training: Ethical Considerations and Practical Steps
This research offers vital insights for improving data annotation by integrating fair compensation and clear instruction design. By embracing these principles, AI developers can ensure higher data quality, improved ethical practices, and a more inclusive and effective future for AI.