Credit Lending: Can AI Really Be Fair? How to Fix Hidden Biases
"Discover how a new AI technique called Subgroup Threshold Optimization (STO) can reduce discrimination in credit lending models by up to 90%."
In today's financial landscape, Artificial Intelligence (AI) is increasingly used to automate credit lending decisions. While AI promises efficiency and accuracy, recent studies reveal a troubling side: these systems can perpetuate biases, unfairly disadvantaging certain groups. Imagine a world where your loan application is unfairly denied not because of your credit history, but because of your gender or other protected characteristic. This is the reality that many face due to hidden biases in AI lending models.
The problem stems from the data used to train these AI systems. If the data reflects historical biases, the AI will learn and amplify these biases, leading to discriminatory outcomes. For example, if past lending practices favored men, an AI trained on this data might unfairly reject creditworthy women. This isn't just unethical; it can also lead to significant financial losses and legal repercussions for lending institutions.
Fortunately, researchers are developing innovative solutions to combat AI bias. One promising technique is Subgroup Threshold Optimization (STO). This method doesn't require altering the original training data or the AI algorithm itself. Instead, it fine-tunes the decision-making thresholds for different subgroups to minimize discrimination and ensure fairer outcomes for all.
Unveiling the Hidden Biases in AI Lending Models
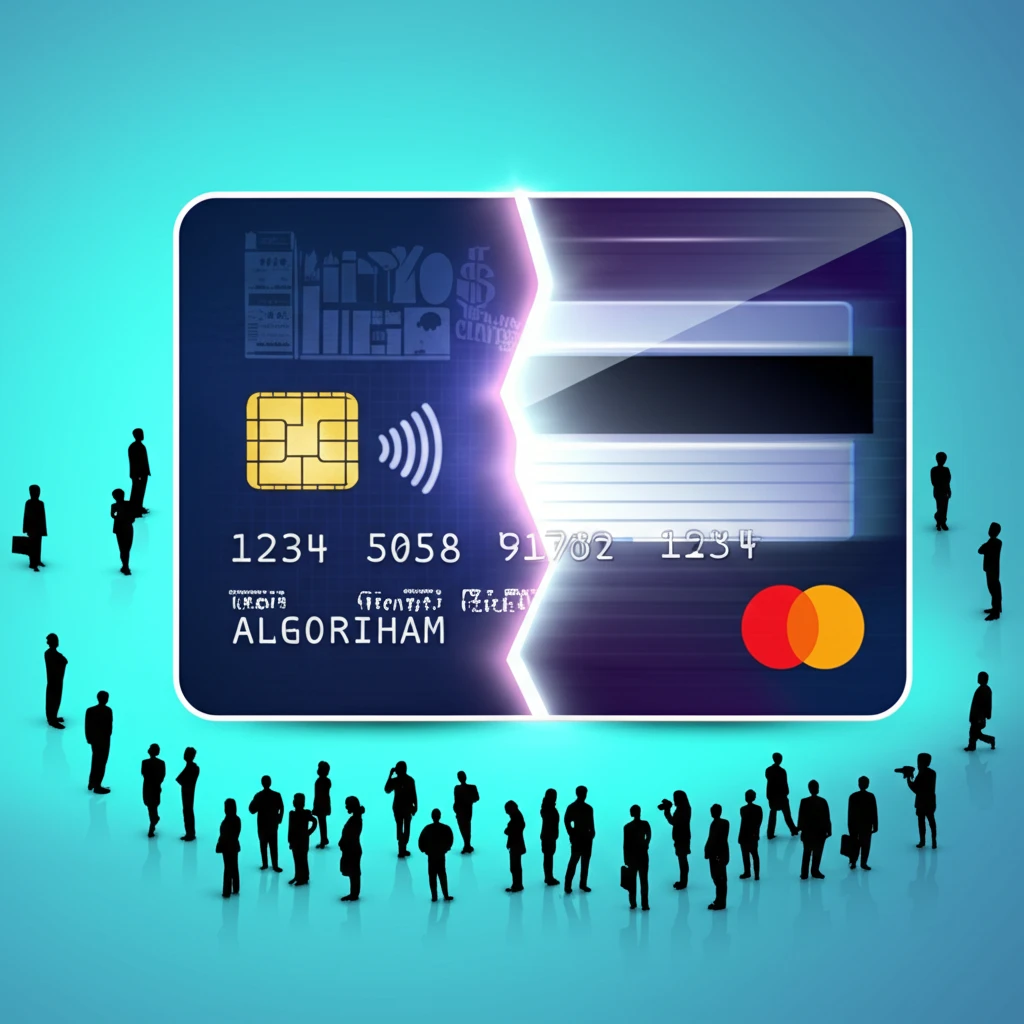
AI bias in credit lending can manifest in various ways. Historical bias occurs when past societal prejudices seep into the data, influencing the AI's decisions. Measurement bias arises when using unsuitable data as proxies for real-world factors. For example, using zip code as a proxy for race can lead to discriminatory lending practices.
- Historical Bias: Past prejudices reflected in training data.
- Measurement Bias: Using inaccurate proxy data.
- Representation Bias: Training data not representative of the population.
- Aggregation Bias: Losing unique features by combining data improperly.
- Evaluation Bias: Ineffective metrics that reward biased outcomes.
The Future of Fair Lending with AI
Subgroup Threshold Optimization (STO) offers a promising path toward fairer AI lending. By fine-tuning decision thresholds for different subgroups, STO minimizes discrimination without requiring extensive changes to existing AI systems. This approach is easy to understand, flexible, and can be implemented by non-experts, making it a practical solution for the credit lending industry. As AI continues to transform the financial landscape, techniques like STO are essential for ensuring that these technologies promote fairness and opportunity for all.