Can AI Spot Your Next Move? Deep Learning and Human Activity Recognition
"Explore how convolutional neural networks are revolutionizing human activity classification in radar technology."
In an era increasingly defined by the need for enhanced security and comprehensive surveillance, human activity classification has emerged as a pivotal area of focus. Its applications span a multitude of sectors, from ensuring patient safety in healthcare to bolstering law enforcement capabilities, aiding search and rescue missions, and enhancing operational effectiveness in urban military contexts. The ability to accurately identify and categorize human actions is proving invaluable in managing and safeguarding our environments.
Traditionally, optical sensors and natural images have been the cornerstone of human detection and classification systems. These technologies, however, are often challenged by fluctuating weather conditions that can significantly impair their performance. Radar systems offer a robust alternative, capable of maintaining operational integrity regardless of environmental factors. They not only overcome weather-related obstacles but also excel in measuring distances and detecting subtle changes in Doppler frequency, which reflect the velocity of a target.
The uniqueness of human movement lies in the distinct motions of different body parts during various activities. Arms, legs, and the torso each contribute unique echoes that, due to differing Doppler shifts, create identifiable micro-Doppler signatures. These signatures, varying over time and typically periodic depending on the target's speed, offer a basis for classifying human activities through characteristic feature analysis. This capability marks a significant advancement in how we interpret and utilize radar data for enhanced security and surveillance applications.
Deep Learning with CNNs: Revolutionizing Activity Recognition
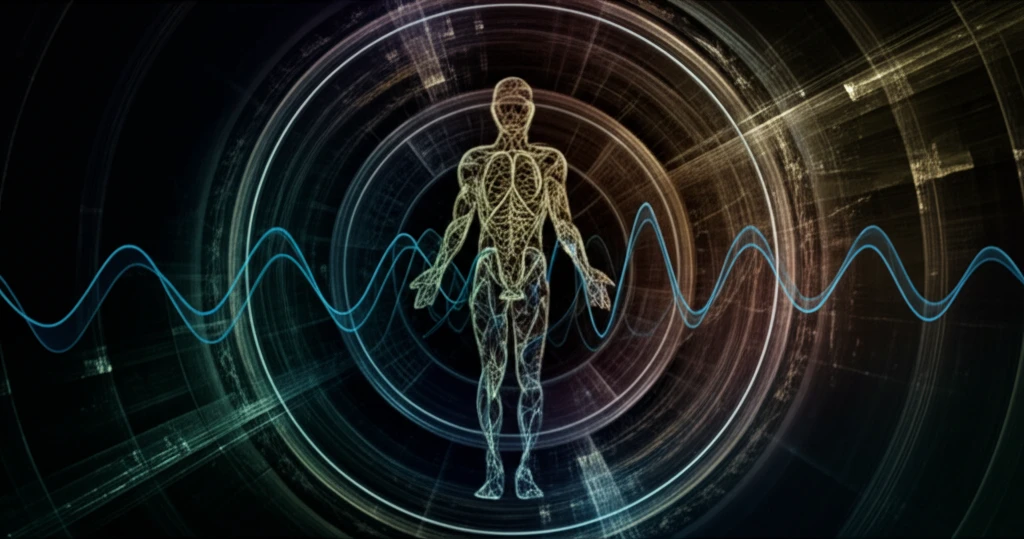
For decades, classifying activities hinged on manually extracting features, a method also applied to radar spectrogram-based target classification. This involved using conventional handcrafted features from spectrograms, such as torso Doppler frequency, signal bandwidth, and Doppler signal strength, to train classifiers. These classifiers then distinguished different human activities, marking a significant step in automated recognition.
- Hidden Markov Models (HMMs): Employed to model instantaneous and correlated micro-Doppler variations.
- Support Vector Machines (SVMs): Trained and optimized with cross-validation to classify human activity effectively.
- Convolutional Neural Networks (CNNs): Used to classify human micro-Doppler spectrograms, offering a deep dive into network performance and training strategies.
The Future of AI in Activity Recognition
The study successfully developed a CNN architecture for classifying human activities in radar micro-Doppler images, achieving a high classification rate across seven activities. By comparing the model with existing networks, the research validated the approach's superiority in accuracy and convergence time. Future work will concentrate on assessing network parameters and training algorithms, enhancing the performance and robustness of AI-driven activity recognition systems. The team aims to refine our understanding and application of CNNs in radar-based human activity classification, pushing the boundaries of what's possible in automated surveillance and security systems.