Unlocking sharper images: How fractional order differentiation is revolutionizing image segmentation
"A deep dive into active contour models and how fractional order differentiation, coupled with selective segmentation, boosts image clarity and detail, even in noisy conditions."
Image segmentation stands as a cornerstone in the realm of image processing, bridging the gap between low-level and high-level operations. It’s the crucial step that allows computers to understand and interpret visual data, enabling everything from medical diagnoses to autonomous vehicle navigation. Traditional methods like thresholding, edge detection, and clustering have long been the go-to techniques. However, these approaches often struggle with adaptability and flexibility, particularly when dealing with complex or noisy images.
Enter active contour models (ACMs), also known as snakes. These models, rooted in the theory of geometric flows and surface evolution, offer a more dynamic and adaptable approach to image segmentation. They can be broadly categorized into edge-based models, which rely on image gradients to guide curve evolution, and region-based models, which use regional image information. Edge-based models excel with clear boundaries but falter with weak edges, while region-based models handle weak boundaries well but struggle with intensity inhomogeneity—when the brightness or color varies significantly across the image.
The challenge then becomes: how do we create an image segmentation technique that combines the strengths of both approaches, offering adaptability, resilience to noise, and the ability to handle varying image intensities? The answer may lie in a groundbreaking approach: integrating fractional order differentiation with selective segmentation.
The Power of Fractional Order Differentiation
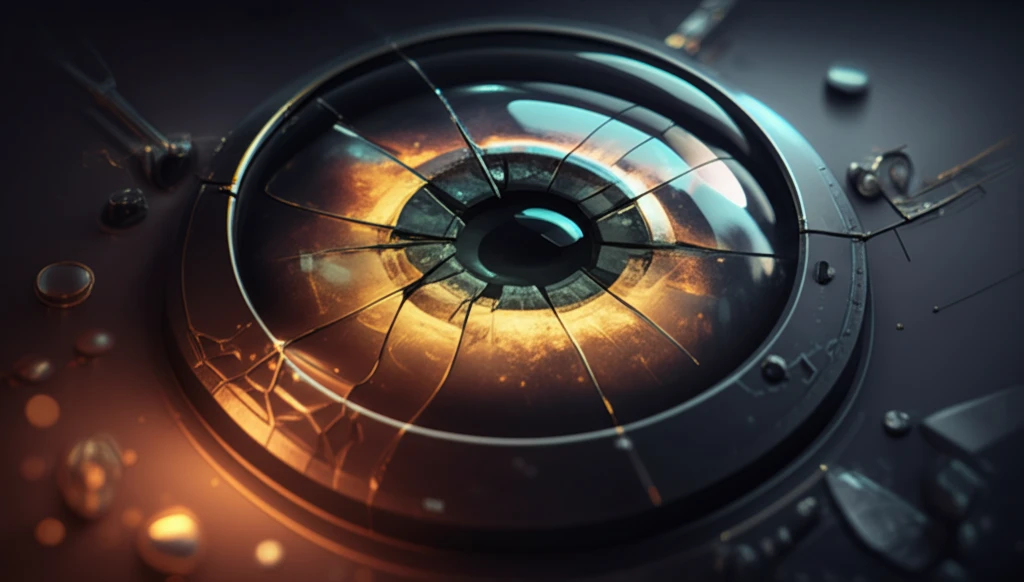
Fractional order differentiation, a concept that extends the traditional integer-order differentiation, has emerged as a powerful tool in various fields, including signal processing and automatic control. Unlike traditional methods, fractional order differentiation provides more precise derivatives, offering a better description of many natural phenomena. Its application in image processing, though relatively new, is proving to be transformative.
- Offers precise derivatives.
- Enhances image details.
- Ideal for highlighting edges.
The Future of Image Segmentation
The active contour model, blending fractional order differentiation and selective segmentation, represents a significant leap forward in image segmentation. By protecting texture, enhancing details, and offering resilience to noise, this model paves the way for more accurate and reliable image analysis in various applications. As technology advances, we can expect further refinements and integrations of these techniques, promising even greater breakthroughs in how machines perceive and interpret the visual world.