Unlocking Hidden Patterns: How Higher Order Eigenvalues are Revolutionizing Data Analysis
"Dive into the groundbreaking research that uses eigenvalues to uncover deeper insights in complex datasets, offering new possibilities across science, technology, and beyond."
In an era overwhelmed by data, the ability to extract meaningful insights from complex datasets is more critical than ever. Traditional analytical methods often fall short when dealing with the intricate relationships within these datasets. However, groundbreaking research is now leveraging advanced mathematical concepts to unlock hidden patterns and revolutionize how we interpret information.
One such area of innovation involves the use of higher-order eigenvalues. Eigenvalues, traditionally used in linear algebra to understand the properties of matrices, are now being applied in more sophisticated ways to analyze complex systems. This approach not only enhances our understanding of the underlying structure of data but also opens up new avenues for practical applications across various fields.
This article delves into the exciting research surrounding multiple sets exponential concentration and higher-order eigenvalues, exploring how these mathematical tools are providing new perspectives on data analysis. We'll break down the core concepts, discuss their implications, and highlight how they're being used to solve real-world problems.
What are Higher Order Eigenvalues and Why Should You Care?
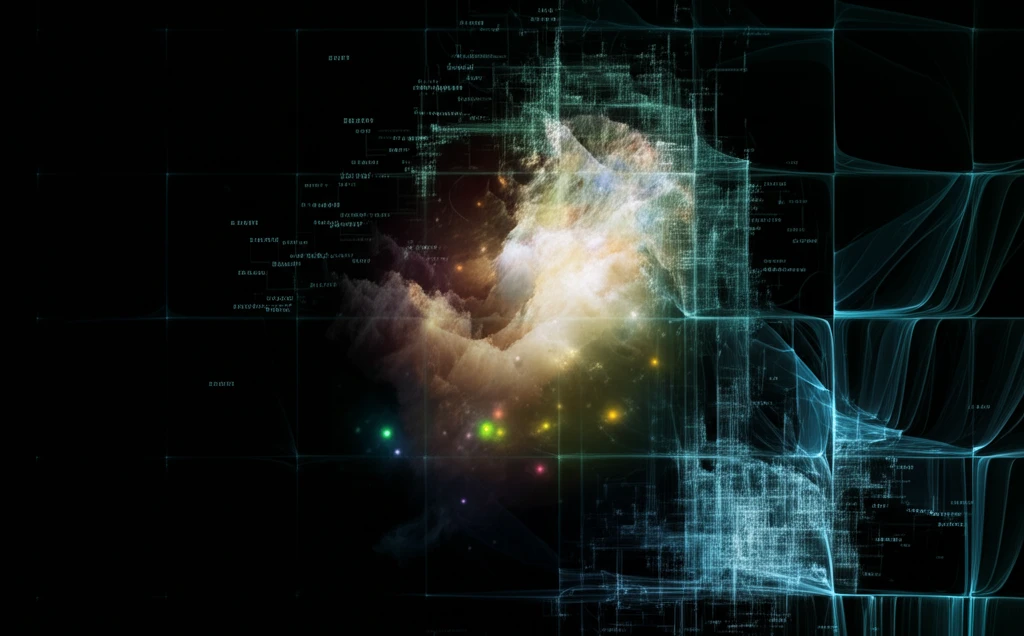
At its core, the research introduces a novel approach to measuring concentration in complex datasets. Imagine you have a vast network, like a social network or a biological system, where elements are interconnected in various ways. Traditional methods might only look at individual connections or simple clusters. Higher-order eigenvalues, however, allow us to analyze the relationships between multiple distinct sets within this network.
- Enhanced Data Interpretation: Uncover hidden patterns and complex interactions within large datasets.
- Improved Accuracy: Provides more accurate and detailed insights compared to traditional methods.
- Broad Applicability: Useful across various fields, including network analysis, machine learning, and more.
The Future of Data Analysis is Here
The research into multiple sets exponential concentration and higher-order eigenvalues represents a significant step forward in our ability to analyze and understand complex data. By providing a more nuanced and sophisticated approach to measuring concentration, this work opens up new possibilities for insights and applications across a wide range of fields. As data continues to grow in volume and complexity, these advanced analytical tools will become increasingly essential for unlocking its hidden potential.