Unlocking Emotions: How AI Facial Recognition Reads Your Feelings
"Dive into the groundbreaking world of AI-driven facial expression analysis and discover how this tech is changing human-computer interactions."
In an increasingly digital world, the quest to bridge the gap between human emotion and artificial intelligence has led to remarkable innovations. One of the most intriguing is facial expression recognition (FER) technology, a field within computer vision and machine learning that seeks to decode the complex tapestry of human emotions through facial cues. From automated access control to enhancing human-computer interactions, the potential applications of FER are vast and transformative.
Facial Expression Recognition is not just about identifying a smile or a frown; it involves a deep dive into the nuances of facial muscle movements, texture variations, and edge patterns. These subtle changes, often imperceptible to the human eye, hold the key to unlocking a person's emotional state. Extracting and interpreting these features accurately is a significant challenge, requiring sophisticated algorithms and computational power.
The journey to create an effective FER system involves several critical stages: acquiring facial images, preprocessing them to enhance clarity, extracting relevant features, and classifying those features into distinct emotional categories. Among these stages, feature extraction stands out as particularly crucial. The accuracy of emotion recognition hinges on the ability to identify and optimize the features that best represent different emotional states.
Decoding the Science: Gabor Filters and Feature Optimization
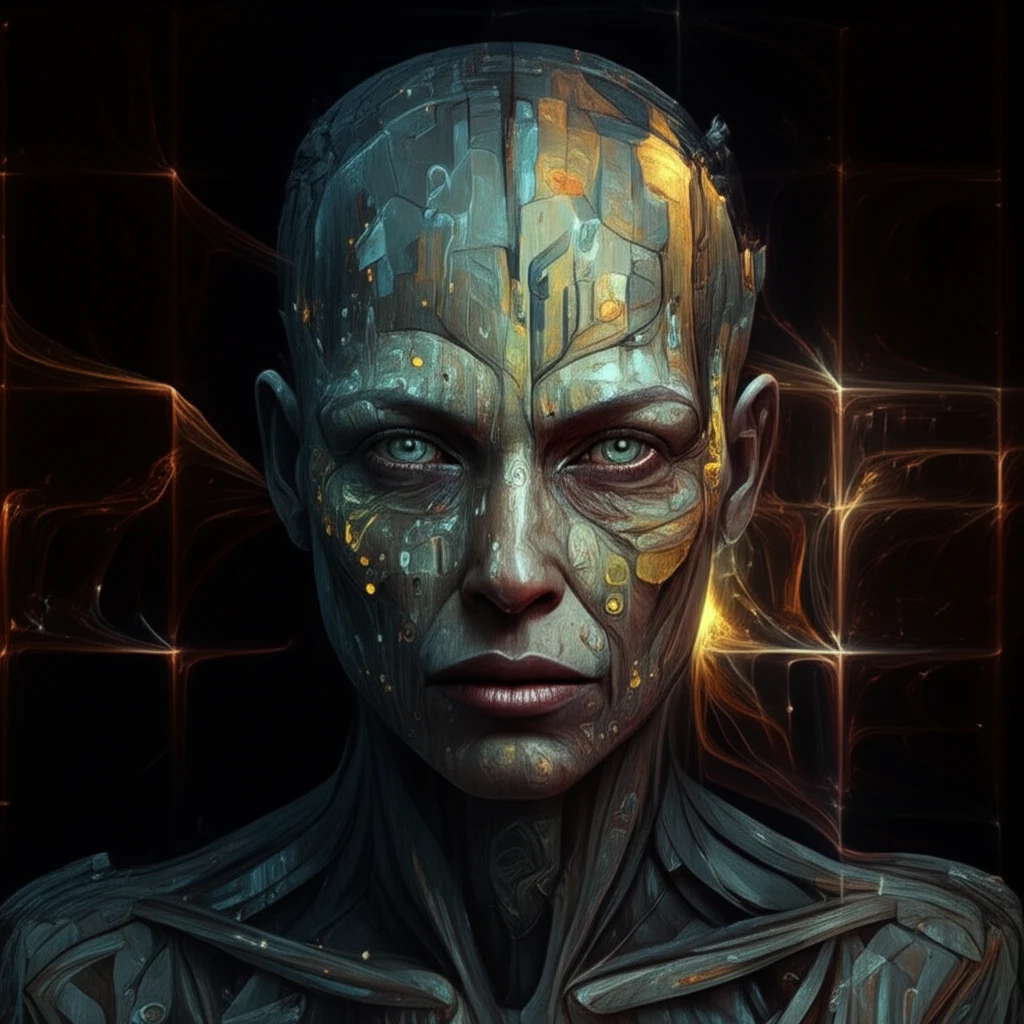
Traditionally, Gabor filters have been a cornerstone in facial feature extraction. These filters excel at capturing edge patterns and texture variations, making them ideal for analyzing facial expressions. However, Gabor filters come with their own set of challenges, primarily the generation of high-dimensional and redundant feature sets, which can bog down processing and reduce accuracy.
- Gabor Filtering: Initial feature extraction using Gabor filters to capture edge and texture information.
- Averaging: Optimizing Gabor features by averaging to reduce redundancy.
- DWT (Discrete Wavelet Transform): Applying DWT to decompose the averaged features into different frequency bands.
- DCT (Discrete Cosine Transform): Further refining the low-frequency components to extract the most salient features.
The Future of Emotion AI: Applications and Ethical Considerations
The advancements in facial expression recognition technology are paving the way for a new era of human-computer interaction. As AI becomes more adept at understanding and responding to human emotions, the potential applications are limitless. From personalized education and healthcare to enhanced security systems and customer service, emotion AI promises to transform the way we interact with technology.