Unlock Your Digital Identity: A Fresh Approach to Face Detection Technology
"Discover the innovative binary texture analysis method revolutionizing face detection in diverse environments."
In today's increasingly digital world, face detection technology is ubiquitous, enhancing security systems, improving user experiences in apps, and even personalizing advertising. The technology has grown far beyond its original scope and is essential for content-based retrieval and video processing. However, current systems often struggle with variations in lighting, skin color, and complex backgrounds, which can reduce accuracy and reliability.
Traditional face detection methods typically involve a series of steps, including initial rough face inspection, window scaling and moving, sub-window preprocessing, and final face judgment using algorithms like neural networks or Adaboost. Each sub-window needs individual preprocessing to highlight or standardize partial objects, and it increases overall complexity, often leading to inefficiencies.
Recent research introduces a new approach that uses binary texture extraction for image preprocessing, potentially improving the robustness and efficiency of face detection. This method aims to simplify and enhance the initial stages of face detection by preprocessing the entire image in a way that standardizes texture, making it easier to identify faces under diverse conditions. The ultimate goal is to create a more reliable and adaptable system for a wide range of applications.
Binary Texture Extraction: A Game Changer
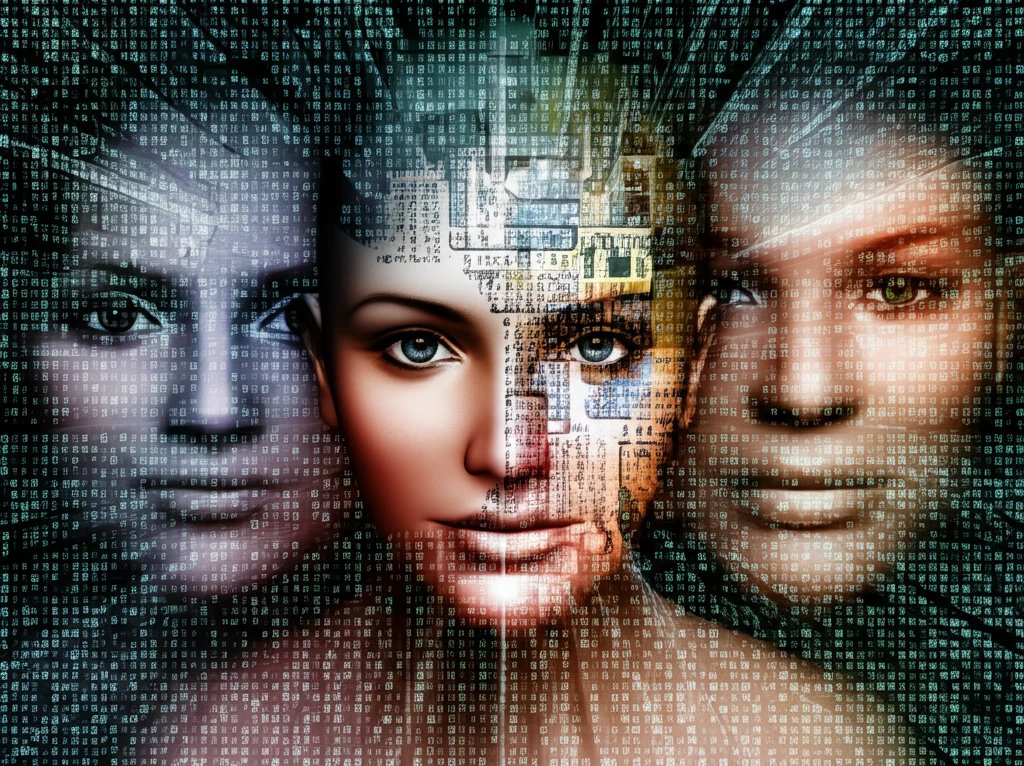
At the heart of this innovative approach is the binary texture extraction algorithm. This technique enhances image preprocessing by focusing on essential features that remain consistent despite varying conditions. This method aims to replicate the detailed effects of independent sub-window processing on a global scale by highlighting key objects and ensuring consistent feature extraction across the entire image.
- Outlines of objects are clearly highlighted.
- Features of concave and convex portions (such as the nose and cheeks) are extracted.
- Reflective effects from different materials are well reflected.
- Fewer image gray levels for an emphasis on object characteristics and a reduction in fine texture interference.
Future Directions and Conclusion
The research successfully combined binary texture extraction with mainstream face detection methods like BP neural networks, Gabor, and Adaboost, demonstrating improved efficiency and accuracy. As the technology evolves, future research should explore the integration of side and rotating faces to ensure a more complete face detection solution. While this method represents a significant step forward, continuous refinement and testing will pave the way for increasingly robust and reliable face detection systems.