Unlock the Future: How Wavelet Neural Networks are Revolutionizing Time Series Prediction
"Discover a groundbreaking approach to time series forecasting with Wavelet Neural Networks, offering new possibilities in data analysis and predictive modeling."
In an era defined by unprecedented data streams, the ability to accurately predict future trends has become invaluable. From financial markets to climate patterns, time series prediction plays a crucial role in decision-making across various sectors. Traditional methods, however, often fall short in capturing the complexities and nuances of real-world data. This is where artificial neural networks (ANNs) step in, offering a robust and adaptable solution.
Artificial Neural Networks, with their capacity to learn from examples and model intricate relationships, have emerged as a popular tool for time series prediction, feature extraction, and pattern recognition. However, ANNs have their limitations. They struggle with local features like discontinuities, abrupt changes, or other anomalies that are common in many datasets. To overcome these limitations, researchers have turned to wavelet neural networks (WNNs), an innovative approach that combines the strengths of neural networks with wavelet analysis.
Wavelet Neural Networks leverage nonlinear wavelet basis functions, localized in both time and frequency, to provide a more detailed and accurate representation of data. This unique approach allows WNNs to capture both global trends and local irregularities, making them particularly well-suited for complex time series prediction problems. Let’s dive into how WNNs work, their architecture, and how they're making waves in predictive analytics.
The Power of Wavelet Neural Networks: A Deep Dive
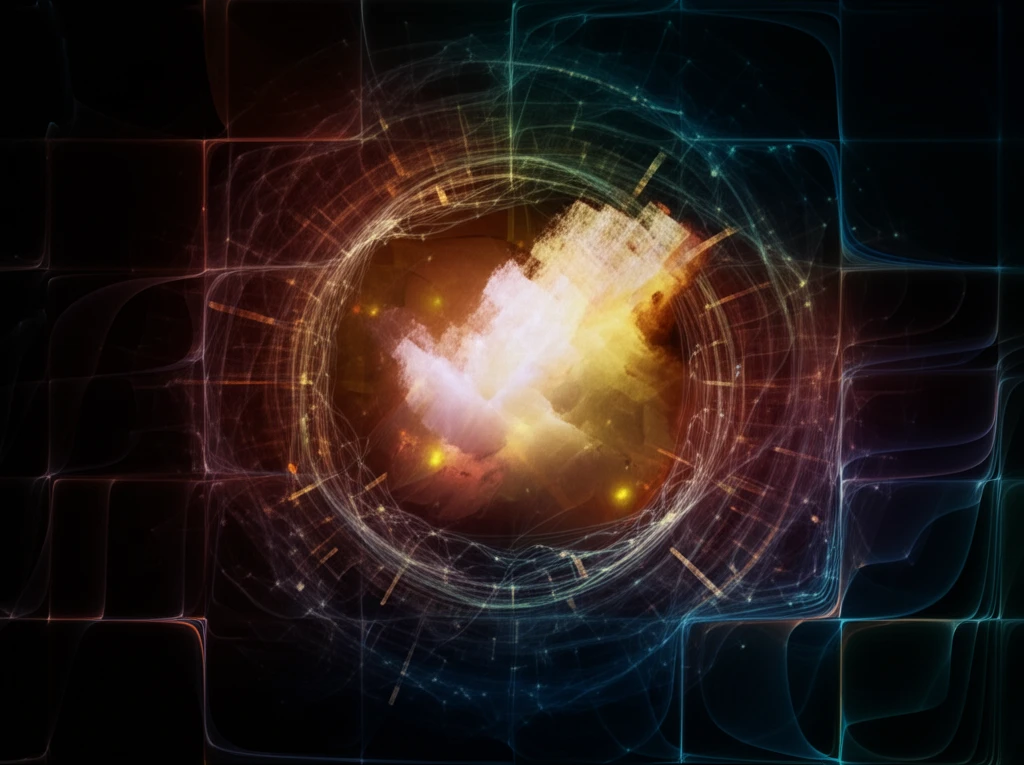
Wavelet Neural Networks (WNNs) represent a significant advancement in the field of neural networks, primarily due to their unique architecture and ability to handle complex data patterns. Unlike traditional ANNs that use sigmoid functions, WNNs employ wavelet functions, which are localized in both time and frequency domains. This localization allows WNNs to capture both high-frequency and low-frequency components of a signal, making them highly effective in time series analysis.
- Superior Local Feature Extraction: WNNs excel at capturing discontinuities, jumps, and other anomalies that traditional ANNs often miss.
- Adaptability: The adjustable parameters in WNN-Type2 architectures allow the network to adapt to varying data characteristics.
- Improved Accuracy: By combining neural networks with wavelet analysis, WNNs often achieve higher accuracy in time series prediction tasks.
The Future of Forecasting: WNNs and Beyond
Wavelet Neural Networks represent a significant leap forward in time series prediction, offering enhanced accuracy, adaptability, and stability compared to traditional methods. By dynamically clustering data and leveraging the unique properties of wavelet functions, WNNs can capture complex patterns and anomalies that are often missed by conventional ANNs. As data streams continue to grow in volume and complexity, WNNs promise to play an increasingly vital role in forecasting and decision-making across various domains, paving the way for more reliable and insightful predictions.