Unlock Smarter Investments: A Data-Driven Guide to Option Pricing
"Navigate the complexities of Asian and Lookback options with cutting-edge, AI-enhanced strategies."
In the dynamic world of finance, accurately pricing path-dependent derivatives like Asian and Lookback options has long been a challenge. These options, where the payoff depends on the underlying asset's price history, require complex calculations that often strain computational resources. Traditional methods often fall short when dealing with the intricacies of stochastic volatility, making precise pricing both time-consuming and elusive.
But what if there was a way to harness the power of data and artificial intelligence to revolutionize option pricing? Imagine slashing computational time while maintaining—or even improving—accuracy. This is the promise of a new, data-driven approach that's transforming how we understand and trade complex financial instruments. This innovative method extends previous work by tackling the challenges of sampling from time-integrated stochastic bridges. By utilizing artificial neural networks to "learn" the distribution of key random variables, this model achieves remarkable efficiency and robustness.
This article explores how this cutting-edge approach is redefining option pricing, making it faster, more accurate, and more accessible than ever before. Whether you're a seasoned financial professional or simply curious about the intersection of AI and finance, understanding these advancements is crucial for navigating the future of investment.
How Does This AI-Powered Option Pricing Work?
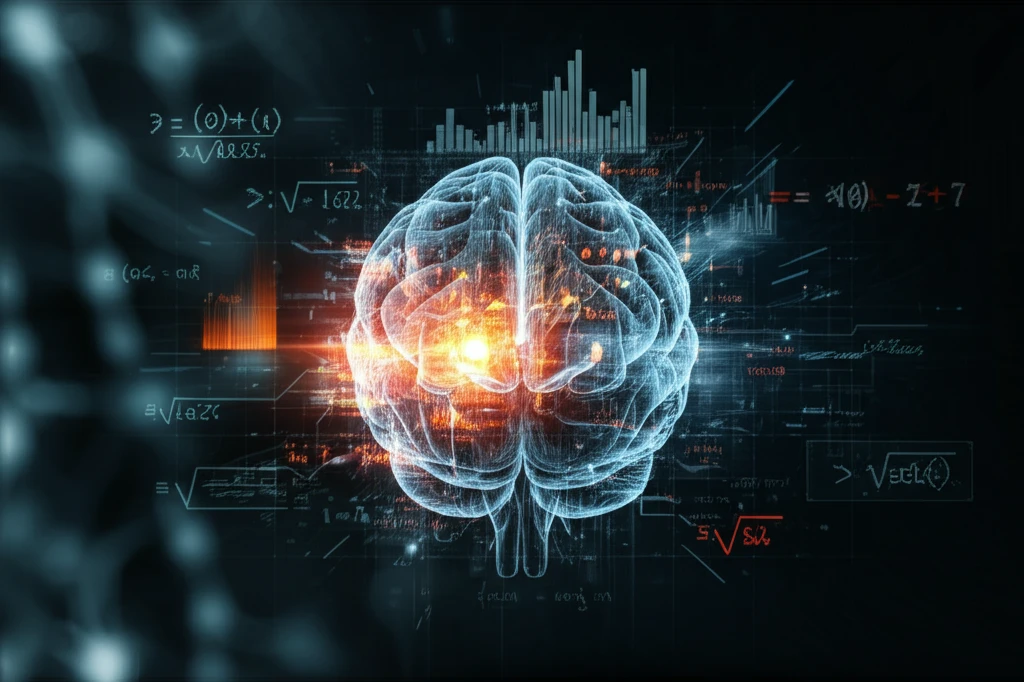
The core of this method lies in a clever combination of stochastic collocation (SC) and artificial neural networks (ANNs). Stochastic collocation is a technique used to approximate complex distributions by strategically selecting a set of points (collocation points) and fitting a simpler function to those points. This drastically reduces the computational burden compared to traditional Monte Carlo methods, which require simulating a vast number of possible scenarios.
- Stochastic Collocation (SC): Approximates complex distributions using strategic points and simpler functions.
- Artificial Neural Networks (ANNs): Learns relationships between option parameters and asset distributions.
- Inverse CDF Approximation: Enables efficient sampling and accurate option pricing.
The Future of Option Pricing is Data-Driven
This innovative approach signals a significant shift in the world of finance, where data and AI are increasingly playing a central role. By combining the power of stochastic collocation with the adaptability of neural networks, this method opens new possibilities for pricing complex financial instruments with unprecedented speed and accuracy. As AI continues to evolve, expect to see even more sophisticated data-driven techniques transforming the financial landscape, making it more efficient, accessible, and ultimately, more profitable for those who embrace the change.