Unlock Hidden Insights: How Dark Knowledge and AI Clustering Can Revolutionize Data Analysis
"Discover the power of dark knowledge in machine learning and how Nonnegative Matrix Factorization (NMF) enhances clustering for better data-driven decisions."
In an era dominated by data, the ability to extract meaningful insights from complex datasets is more critical than ever. Clustering approaches, which group similar objects together, have become a staple in various fields, from identifying customer segments to classifying genes. However, traditional clustering methods often struggle with robustness and stability, leading to the emergence of clustering ensemble (CE) algorithms. These algorithms combine the results of multiple basic clustering methods to achieve more accurate and reliable outcomes.
Traditional CE methods primarily rely on the labels produced by base learning algorithms. But what if these algorithms could offer more than just labels? What if they could provide additional information, such as parameter settings, covariance data, or probability distributions, that remains hidden or unused? This untapped reservoir of information is what researchers refer to as 'dark knowledge.'
A recent study from Southwest Jiaotong University explores how to integrate this often-ignored dark knowledge into the ensemble learning process. By applying Nonnegative Matrix Factorization (NMF) to a clustering ensemble model based on dark knowledge, the researchers have developed a novel approach that uncovers hidden patterns and improves clustering performance. This article dives into the details of this innovative method and its potential applications.
What is Dark Knowledge and How Can It Improve Clustering?
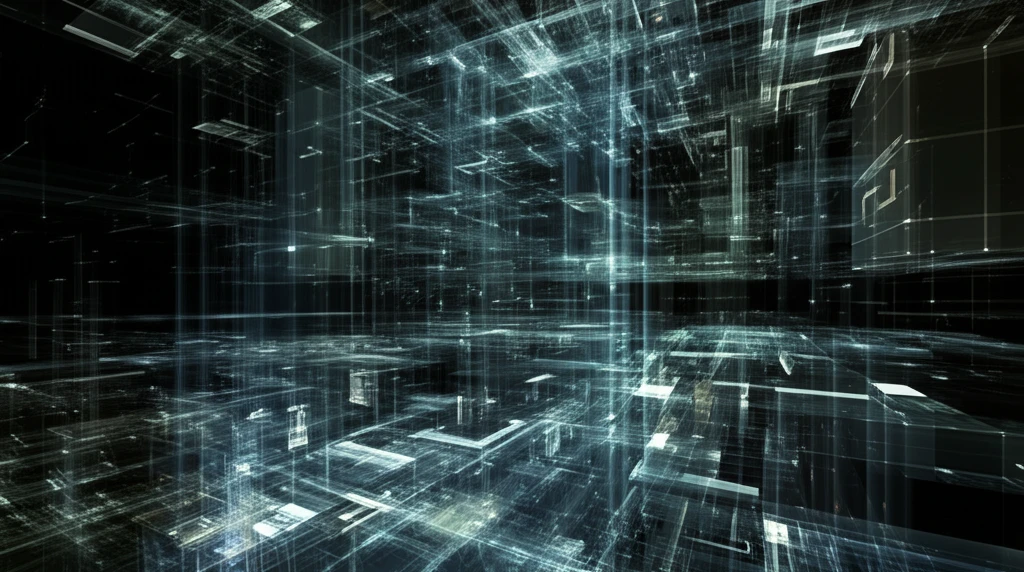
Dark knowledge, in the context of machine learning, refers to the valuable information that remains hidden or unused within complex models and large datasets. In traditional clustering ensembles, this includes parameters, covariance, or probability data generated by base learning algorithms. Ignoring this information can lead to suboptimal clustering performance and missed opportunities for deeper insights.
- Extracting Dark Knowledge: The process begins by running multiple base clustering algorithms with varied configurations to generate diverse clustering results.
- Applying NMF: NMF is then applied to the extracted dark knowledge to identify underlying structures and relationships.
- Integrating Results: The results from NMF are integrated to produce a final clustering that leverages both the explicit labels and the hidden information within the data.
The Future of Clustering: Embracing the Dark Side
The innovative NMFCE method represents a significant step forward in the field of clustering ensembles. By harnessing the power of dark knowledge, this approach unlocks hidden insights and improves the accuracy and robustness of clustering results. As data continues to grow in volume and complexity, techniques like NMFCE will become increasingly essential for extracting valuable information and making data-driven decisions.