Unlock Global Communication: How AI is Revolutionizing Language Translation
"Discover the latest advancements in neural machine translation and how they're breaking down language barriers, enhanced sub-word units, and synthetic data techniques that enhance translation accuracy"
In today's interconnected world, language barriers can significantly hinder effective communication. Recent advancements in artificial intelligence, particularly in the field of neural machine translation (NMT), are revolutionizing how we bridge these gaps. Neural machine translation systems have demonstrated state-of-the-art capabilities across numerous language pairs. This technology leverages deep learning to translate text, marking a substantial shift from older methods that depended on pre-defined rules and phrase-based approaches.
One of the key challenges in machine translation involves languages rich in morphology, where word forms can vary extensively. Data sparsity—the lack of sufficient training examples for all possible word variations—poses a significant hurdle. To combat this, researchers have turned to innovative techniques such as sub-word units. Introduced by Sennrich et al., this method breaks words into smaller, more manageable parts, allowing the system to better understand and translate rare and unknown words.
This approach helps retain the speed and robustness of word-level systems. Yet, because these algorithms often rely solely on character statistics from training data, they may not always align with the underlying morphological structure of words. The aim is to explore ways to refine word splitting strategies, thereby enhancing the accuracy and reliability of machine translation systems, especially for highly inflected languages.
Enhancing Translation Through Morphological Analysis and Sub-Word Units
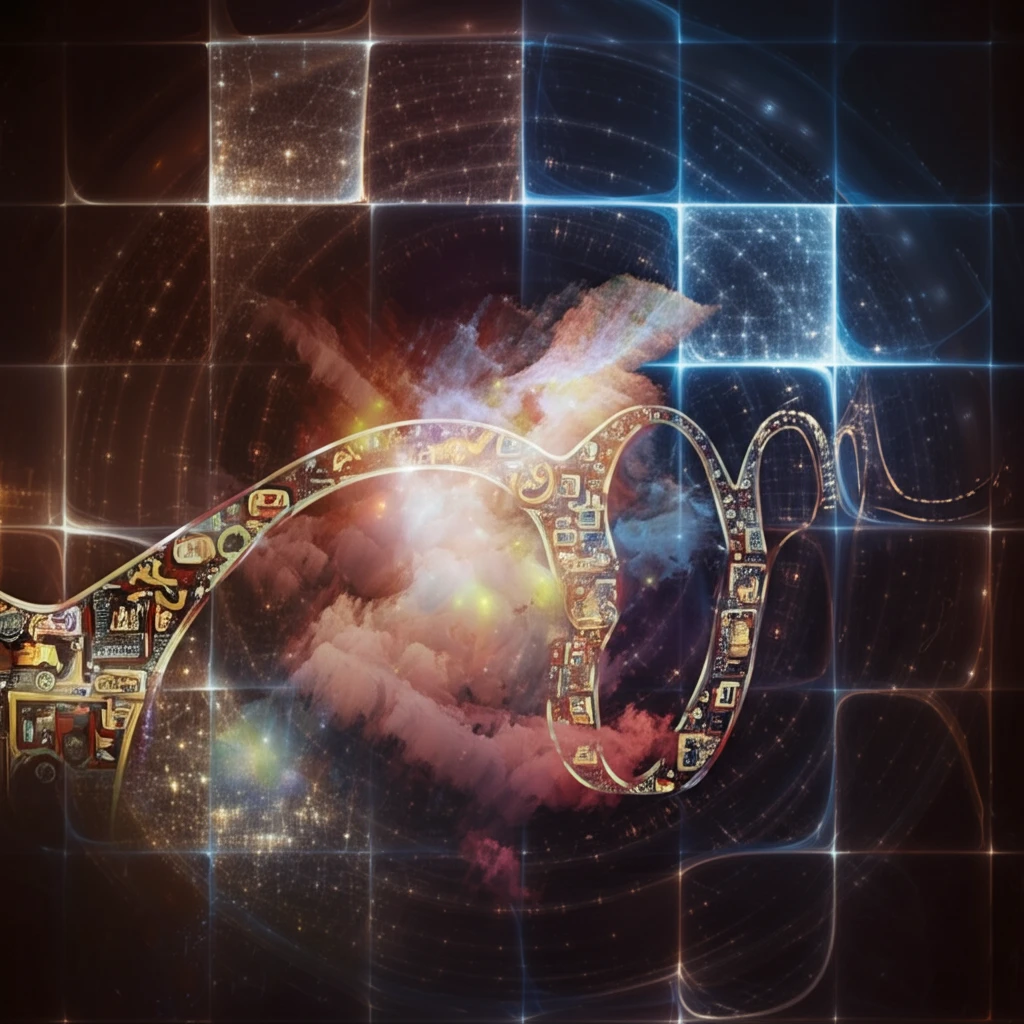
Traditional methods of machine translation often struggle with the inconsistencies in how words are split and translated, particularly in morphologically rich languages. Byte Pair Encoding (BPE), a common algorithm used in sub-word NMT, sometimes separates roots and affixes inconsistently because it operates agnostically to language structure. For example, different forms of the same word might be split in varying ways, leading to a lack of uniformity in translation.
- Compound Splitting: Breaking down compound words into their constituent parts.
- Prefix Separation: Identifying and separating common prefixes such as 'above-,' 'some-,' and 'every-' in English, or 'pie-,' 'uz-,' and 'ne-' in Latvian.
- Suffix Separation: Isolating suffixes to better understand the grammatical function and meaning of words.
- Morphological Analyzer: Splitting words based on morphological analysis increases consistency across different surface forms and words sharing the same roots. This ensures that translations are more accurate and contextually appropriate.
The Future of AI-Driven Language Translation
The ongoing evolution of AI in language translation promises a future where communication is seamless and universally accessible. By continuing to refine techniques for handling rare and unknown words, and by leveraging synthetic data to enhance training, we can expect even more robust and reliable translation systems. These advancements are crucial for fostering global collaboration, understanding, and connectivity in an increasingly interconnected world.