Unlock Competitive Insights: How Nested Pseudo Likelihood Estimation Revolutionizes Dynamic Game Analysis
"Discover the power of continuous-time modeling in dynamic discrete games and learn how the Nested Pseudo Likelihood (NPL) method offers a more accurate and efficient approach to economic analysis."
In the fast-paced world of economics, understanding how companies make decisions is key to predicting market trends and crafting effective policies. One powerful tool for analyzing these decisions is the dynamic discrete choice model. These models help us understand how businesses choose between different options, like entering or leaving a market, by considering not just the immediate payoffs but also the anticipated future consequences.
However, traditional dynamic models often use a discrete-time framework, which assumes that decisions are made at fixed intervals. This approach can be limiting because real-world decisions often occur at irregular times. To address this, economists have been developing continuous-time dynamic discrete choice models that allow for more flexible timing of decisions. These models offer a more realistic representation of economic activity, especially in complex scenarios like competitive markets.
This article dives into a cutting-edge method for estimating continuous-time dynamic discrete choice models: the Nested Pseudo Likelihood (NPL) estimator. We'll explore how this technique adapts insights from discrete-time models to provide more accurate and efficient analyses of market dynamics, strategic interactions, and the potential impacts of policy interventions.
What is Nested Pseudo Likelihood (NPL) Estimation and Why Does it Matter?
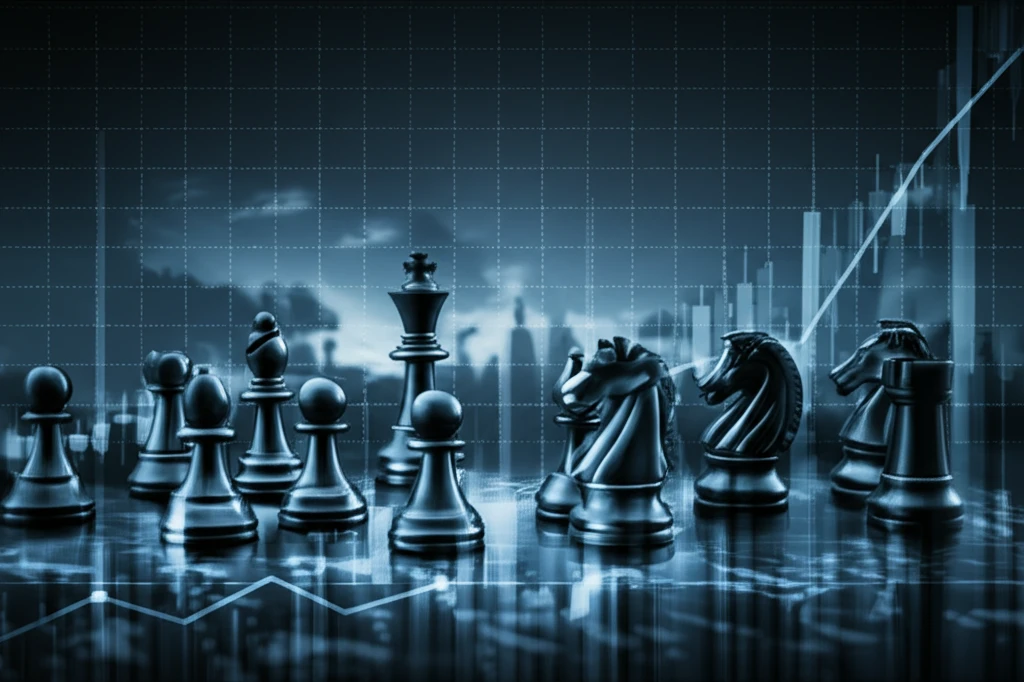
The Nested Pseudo Likelihood (NPL) method offers a sequential approach to estimating continuous-time dynamic discrete choice models. It cleverly adapts the NPL techniques developed by Aguirregabiria and Mira for discrete-time settings. The key advantage? NPL allows economists to analyze decision-making in scenarios where time is continuous and data is sampled either at specific moments (discretely) or continuously over time.
- Consistency and Normality: NPL estimators, under certain conditions, provide consistent and asymptotically normal estimates. This means that as the sample size grows, the estimator converges to the true value and its distribution becomes well-defined.
- Local Convergence: Guarantees that the estimator converges to a stable solution in the vicinity of the true parameter values.
- Zero Jacobian Property: In single-agent models, the NPL estimator exhibits a unique "zero Jacobian" property, which simplifies the estimation process and ensures local convergence.
Why This Matters for Your Business and Economic Insights
The Nested Pseudo Likelihood estimator marks a significant advancement in the analysis of dynamic discrete choice models. By providing a more accurate and efficient method for continuous-time analysis, NPL empowers researchers and businesses to gain deeper insights into competitive dynamics, strategic decision-making, and the potential consequences of policy interventions. Embrace these advanced techniques to stay ahead in the ever-evolving economic landscape.