Unlock AI Success: How Clear Instructions and Fair Pay Boost Data Annotation
"Discover the secrets to improving AI model accuracy through better data annotation practices – it's all about clear rules and rewarding your workforce."
Artificial intelligence is rapidly transforming industries, creating new job opportunities alongside displacing existing ones. One critical, often unseen, aspect of AI development is data annotation – the process of labeling images, text, and other data that AI models use to learn. The quality of this annotation directly impacts the accuracy and reliability of AI, making it a cornerstone of successful AI implementation.
Think of data annotation as teaching an AI. If the teaching materials are confusing or the teacher isn't properly motivated, the student won't learn effectively. This article delves into the economics of data annotation, focusing on how the design of task instructions (clear rules vs. vague standards) and monetary incentives influence data quality and overall project costs.
We'll explore groundbreaking research that reveals the surprising benefits of providing clear, rule-based instructions and fair compensation to data annotators. Discover how these factors not only improve the accuracy of AI models but also contribute to a more ethical and efficient AI development process. This isn't just about algorithms; it's about people and the future of work in the AI economy.
The Power of Clear Rules: Why Structure Matters in Data Annotation
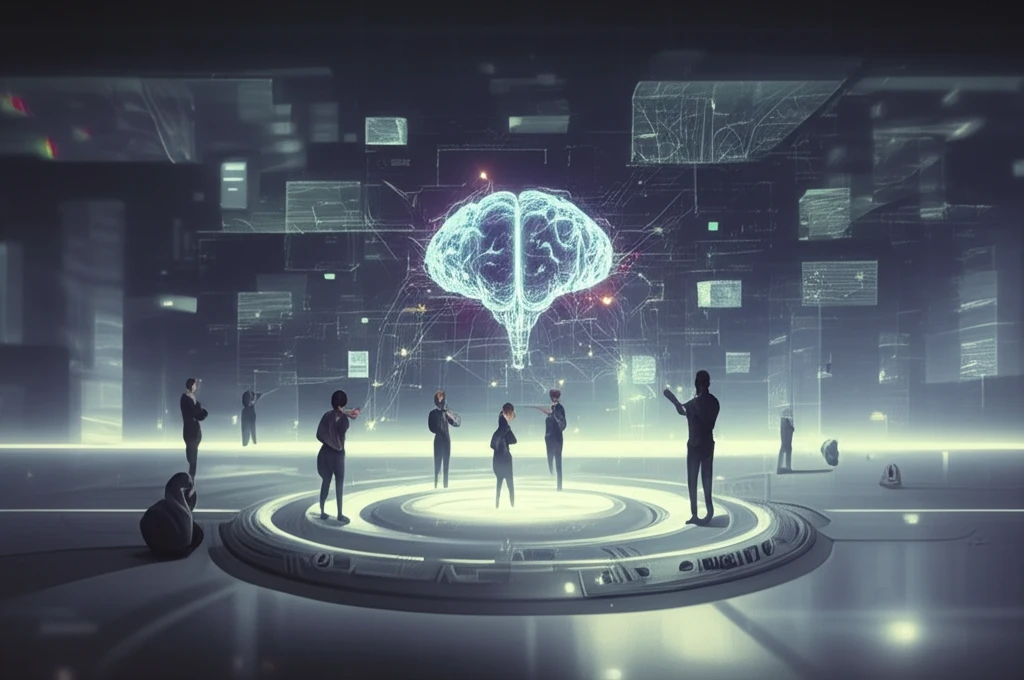
Imagine trying to assemble furniture with instructions that are vague and open to interpretation. The result is likely to be wobbly and unreliable. Data annotation is no different. Research has consistently demonstrated that clear, well-defined rules lead to higher accuracy rates compared to ambiguous standards.
- Reduced Ambiguity: Clear rules minimize confusion and ensure consistent application of guidelines.
- Improved Consistency: Rule-based instructions lead to more uniform annotation across different annotators.
- Higher Accuracy: Structured guidance translates directly into more accurate data labeling.
The Future of AI: Ethical Data and Human Well-being
The insights presented here offer a roadmap for building more accurate, reliable, and ethical AI systems. By prioritizing clear instructions and fair compensation for data annotators, organizations can unlock the full potential of AI while fostering a more equitable and sustainable AI development ecosystem. The future of AI depends not only on technological advancements but also on the well-being and effectiveness of the human workforce that powers it.