Spotting the Unseen: How AI is Revolutionizing Anomaly Detection
"Uncover hidden patterns and safeguard your data with cutting-edge anomaly detection techniques powered by incremental decision trees."
In today's data-rich world, the ability to quickly and accurately identify anomalies is more critical than ever. From detecting fraudulent transactions to spotting network intrusions and predicting equipment failures, anomaly detection plays a vital role in safeguarding our digital lives and optimizing operational efficiency. Traditional methods often struggle to keep pace with the increasing volume and complexity of data, creating a need for more sophisticated solutions.
Enter Artificial Intelligence (AI). AI-powered anomaly detection is rapidly transforming the field, offering advanced techniques that can learn complex patterns and identify subtle deviations from the norm. Among these innovations, incremental decision trees have emerged as a powerful tool for online outlier detection, providing real-time analysis and adaptation to changing data streams.
This article delves into the world of AI-driven anomaly detection, with a focus on the groundbreaking approach of using incremental decision trees. We'll explore how this method works, its advantages over traditional techniques, and its potential applications across various industries. Whether you're a data scientist, a cybersecurity professional, or simply curious about the future of AI, this article will provide valuable insights into this exciting and rapidly evolving field.
Why Traditional Anomaly Detection Falls Short
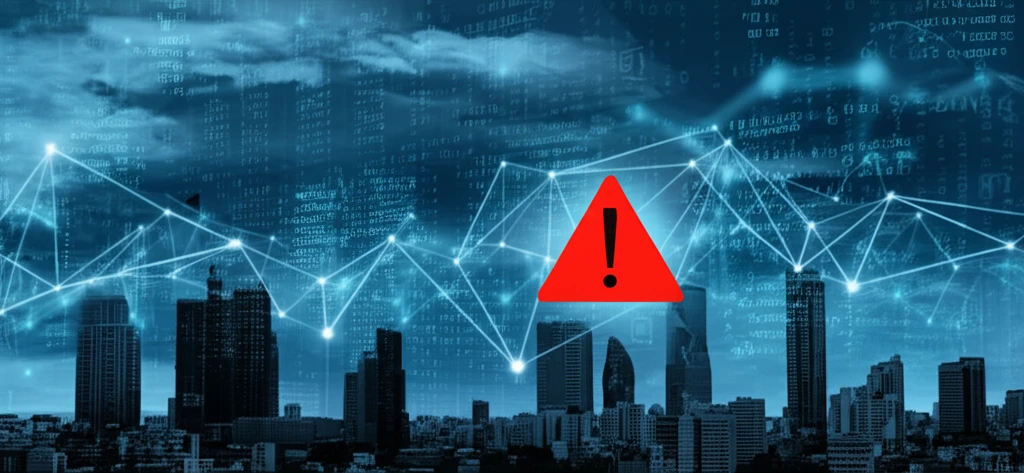
Traditional anomaly detection methods often rely on static thresholds and predefined rules, which can be inflexible and ineffective in dynamic environments. These approaches struggle to adapt to evolving data patterns and may generate numerous false positives or miss subtle anomalies.
- Lack of Adaptability: Traditional methods fail to adjust to changing data patterns, leading to decreased accuracy over time.
- High False Positive Rates: Static thresholds can flag normal behavior as anomalous, creating alert fatigue and wasted resources.
- Inability to Detect Subtle Anomalies: Simple rules may miss complex or hidden patterns that indicate malicious activity or system failures.
- Manual Configuration and Maintenance: Traditional systems often require manual tuning and updates, which can be time-consuming and costly.
The Future of Anomaly Detection
AI-powered anomaly detection is poised to revolutionize the way we protect our data and optimize our systems. As AI algorithms become more sophisticated and data volumes continue to grow, we can expect even more accurate and efficient anomaly detection solutions to emerge, paving the way for a safer and more productive future. By embracing these innovations, organizations can unlock the power of their data to proactively identify threats, prevent failures, and gain a competitive edge.