Predicting Traffic Flow: How a Hybrid Fuzzy Model Can Help You Avoid Congestion
"Combining Data and Fuzzy Logic for Smarter Traffic Predictions"
Urban traffic congestion is a growing problem, causing delays, frustration, and economic losses. Effective traffic flow prediction is essential for making informed decisions, optimizing traffic management, and providing travelers with timely information to avoid congested routes. Accurately forecasting traffic conditions can significantly improve urban mobility and reduce the negative impacts of traffic jams.
Traditional methods for traffic flow prediction often rely on statistical models or artificial intelligence techniques. While these approaches have shown promise, they may struggle to capture the complexities and uncertainties inherent in real-world traffic patterns. To address these limitations, researchers have been exploring hybrid models that combine the strengths of multiple approaches.
One such hybrid model, a data-driven fuzzy model, has emerged as a promising solution for short-term traffic flow prediction. This innovative approach leverages the power of data analysis and fuzzy logic to provide more accurate and reliable forecasts. By understanding how this model works, urban commuters and traffic planners can gain valuable insights into managing and navigating urban traffic.
What is a Hybrid Fuzzy Model for Traffic Flow Prediction?
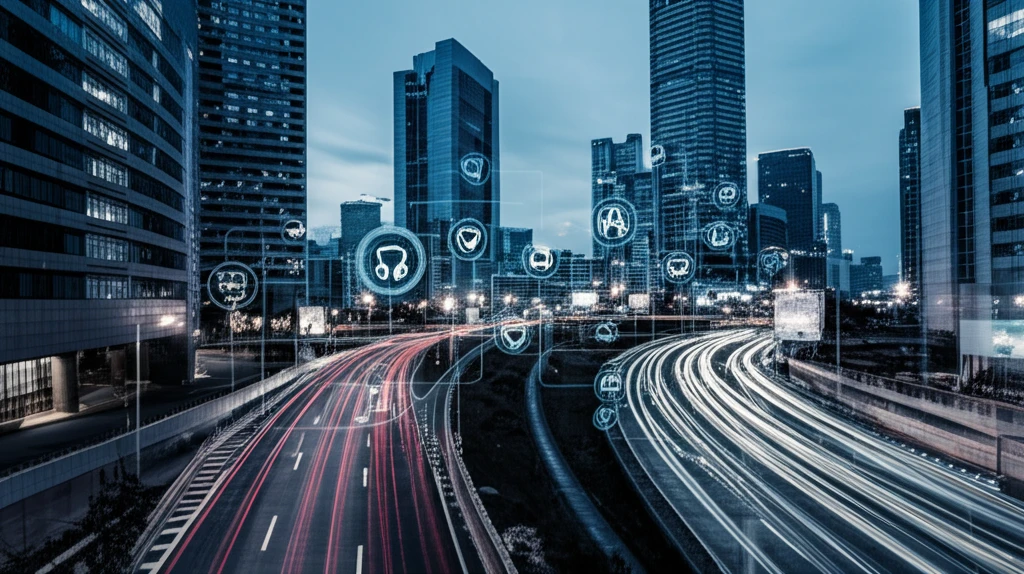
A hybrid fuzzy model for traffic flow prediction combines data-driven techniques with fuzzy logic to enhance prediction accuracy. This model extracts periodicity patterns from traffic flow data and constructs a Functionally Weighted Single-Input-Rule-Modules Connected Fuzzy Inference System (FWSIRM-FIS) to handle the residual data. By integrating periodicity patterns and FWSIRM-FIS outputs, the model generates final prediction results.
- Periodicity Extraction: The model first identifies and extracts recurring patterns in traffic flow data, such as daily or weekly cycles.
- FWSIRM-FIS Construction: A fuzzy inference system is built to model the residual data after removing the periodic components. This system uses single-input rule modules connected in a specific way to capture complex relationships.
- PACF Method for Optimal Inputs: The Partial Autocorrelation Function (PACF) method determines the optimal inputs for the FWSIRM-FIS model, ensuring that the most relevant variables are considered.
- Iterative Least Square Method: This method trains the parameters of the FWSIRM-FIS, optimizing the model's performance and accuracy.
- Integration of Results: The final prediction is generated by combining the periodicity pattern and the output from the FWSIRM-FIS model.
The Future of Traffic Prediction
The data-driven hybrid fuzzy model represents a significant advancement in traffic flow prediction. By combining the knowledge model with the residual data-driven FWSIRM-FIS model, this approach offers a more accurate and reliable way to forecast short-term traffic conditions. This can improve decision-making for traffic managers and help commuters plan their routes more effectively, ultimately leading to reduced congestion and improved urban mobility. As research continues, we can expect further innovations that integrate mathematical models with data-driven approaches to enhance the accuracy and applicability of traffic flow predictions.