Precision in Paediatrics: The Quest for Sharper Liver Scans
"New AI Advances Promise Safer, More Accurate Medical Imaging for Children"
The delicate nature of paediatric healthcare requires a constant balance between diagnostic accuracy and patient safety. When it comes to medical imaging, children are particularly vulnerable to the harmful effects of radiation. This is why combined PET-CT scanners, which offer lower radiation doses, are increasingly used. However, the resulting CT images often suffer from low contrast, making it challenging for doctors to accurately segment and analyze the liver.
Imagine trying to find a single puzzle piece in a box where all the pieces are the same color. That's the challenge doctors face when trying to segment a child's liver in a low-contrast CT scan. Accurate segmentation is crucial for diagnosis, treatment planning, and monitoring disease progression. Manual segmentation is time-consuming and prone to human error. This has spurred researchers to develop automated methods that can tackle the challenge effectively.
The field of liver segmentation has seen remarkable progress, with techniques ranging from probabilistic atlases to statistical shape models. Yet, the unique challenges posed by low-contrast paediatric images demand innovative solutions. A new study introduces an adaptive kernel-based Statistical Region Merging (SRM) algorithm, offering a promising step forward in the quest for precise and safe liver segmentation in children.
Adaptive Kernel-Based SRM: A Closer Look
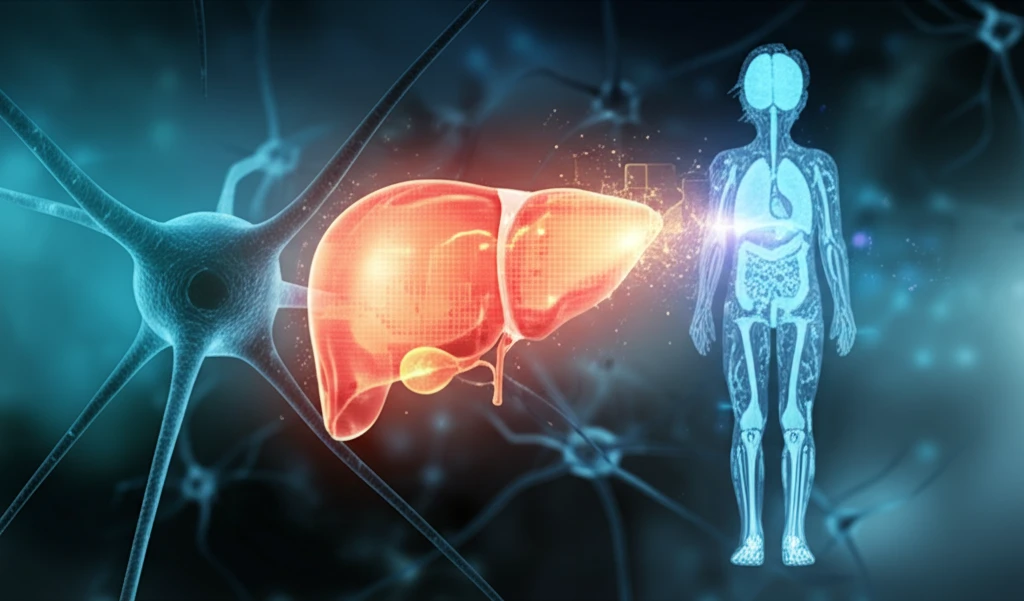
The core of this innovation lies in the adaptive kernel-based Statistical Region Merging (SRM) algorithm. It’s a mouthful, but the concept is ingenious. The algorithm is designed to address the issue of low contrast by merging pixels into statistically homogenous regions. The algorithm then leverages an adaptive kernel that sharpens image clarity to allow the automated systems to detect differences more readily. This enhancement is critical for accurately defining the liver's boundaries. The kernel adapts to the characteristics of each image, effectively smoothing out noise and enhancing relevant features.
- Improved Accuracy: Achieves a higher Dice index compared to traditional SRM methods.
- Enhanced Robustness: Successfully segments all CT images, even those with significant low contrast.
- Reduced Manual Intervention: Automates the segmentation process, saving time and resources.
- Personalized Adaptation: Adapts to the unique noise characteristics of each image.
The Future of Paediatric Liver Imaging
While these results are promising, the researchers acknowledge that further validation with a larger dataset is essential. This highlights the ongoing need for collaborative efforts to refine and expand the applications of AI in paediatric medical imaging. The journey toward safer, more accurate diagnostics for children is a continuous one, and innovations like the adaptive kernel-based SRM algorithm pave the way for a brighter future.