Human vs. Machine: Can Algorithms Truly Understand Context?
"Explore the limitations of AI in capturing the nuances of human judgment and discover when the human touch still matters."
Artificial intelligence is rapidly transforming industries, with algorithms now capable of performing tasks once exclusively handled by human experts. From medical diagnoses to financial forecasting, machines are making inroads into complex decision-making processes. This begs the question: Are algorithms truly equipped to replace human judgment entirely?
A key difference lies in how humans and algorithms approach evaluation. Algorithms typically rely on standardized data sets, applying the same criteria to every individual. Human evaluators, on the other hand, possess the ability to customize their approach, taking into account individual circumstances and contextual factors that may not be readily quantifiable. This "value of context" has sparked debate about the true potential and limits of AI-driven evaluation.
Recent research delves into this very issue, examining the strengths and weaknesses of both human and algorithmic evaluators. The findings suggest that while algorithms excel at processing vast amounts of data, they often struggle to replicate the nuanced understanding that humans bring to the table. Let's explore when and why context matters, and how it shapes our preferences between human insight and machine precision.
The Algorithmic Approach: Standardized, Swift, and Sometimes Shortsighted
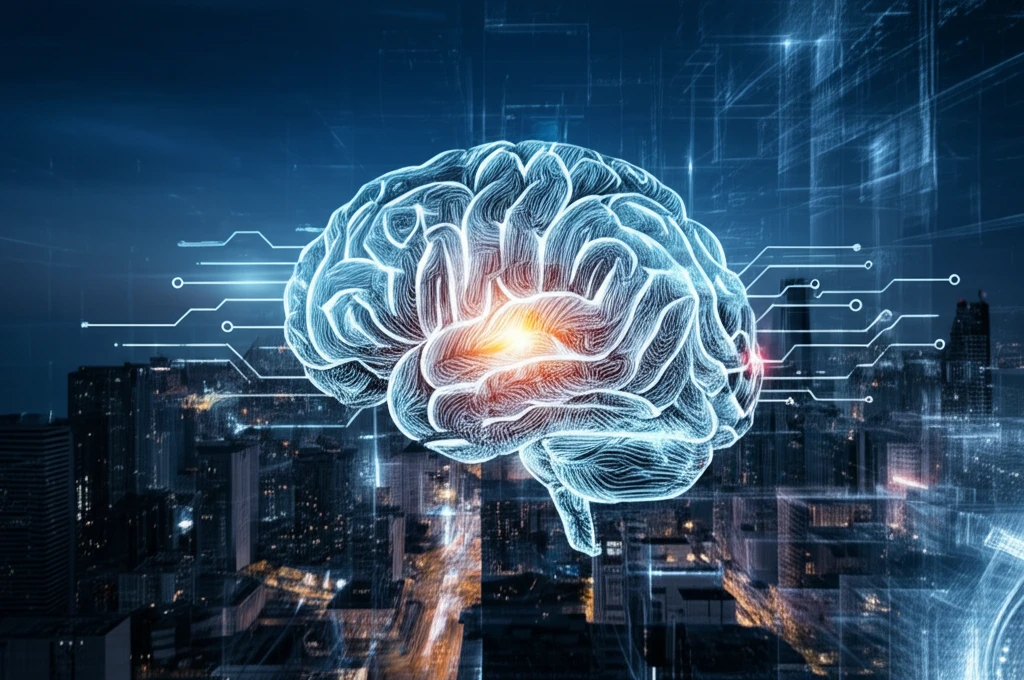
Algorithms shine when it comes to processing large, standardized datasets. They can identify patterns and correlations that might escape human notice, leading to swift and efficient evaluations. For instance, in medical imaging, AI can analyze thousands of scans to detect subtle anomalies indicative of disease. This capability can be invaluable in screening large populations and accelerating diagnoses.
- Lack of Flexibility: Algorithms are rigid, following pre-set rules.
- Data Dependency: Their accuracy is limited by the data they're trained on.
- Context Blindness: They often miss the "big picture" that humans naturally grasp.
Navigating the Future: Embracing a Balanced Approach
As AI continues to evolve, the key lies in finding the right balance between algorithmic efficiency and human understanding. Rather than viewing them as mutually exclusive, we should strive to integrate their respective strengths. This might involve using algorithms to sift through vast datasets and identify potential areas of concern, while relying on human experts to investigate the nuances of individual cases and make informed judgments. The future of evaluation, it seems, hinges on our ability to harness the power of AI without sacrificing the irreplaceable value of human context.