Federated Learning: Democratizing AI or Just Hype?
"Explore how federated learning is revolutionizing artificial intelligence by enabling collaborative model training across diverse data sources while keeping sensitive information safe."
Imagine training a powerful AI model without ever needing to gather all the data in one central place. That’s the promise of federated learning, a technique that allows machine learning models to learn from decentralized data sources, all while preserving the privacy of individuals and organizations. In today's world, data is more distributed than ever, and concerns about privacy are paramount. Federated learning offers a way to harness the power of AI without compromising on these critical considerations.
This approach is particularly relevant now as businesses and researchers grapple with massive datasets spread across various locations and devices. From hospitals holding patient records to smartphones collecting user behavior, the potential for AI is immense, but so are the challenges of data governance and security. Federated learning addresses these head-on, providing a framework for collaborative model training without the risks associated with traditional, centralized approaches.
But is federated learning truly a game-changer, or is it just another overhyped technology? In this article, we'll dive deep into the world of federated learning, exploring its core principles, real-world applications, and the tradeoffs involved in its implementation. We'll also examine how it's being used to tackle complex problems in various industries, and discuss whether it lives up to its promise of democratizing AI.
How Does Federated Learning Actually Work?
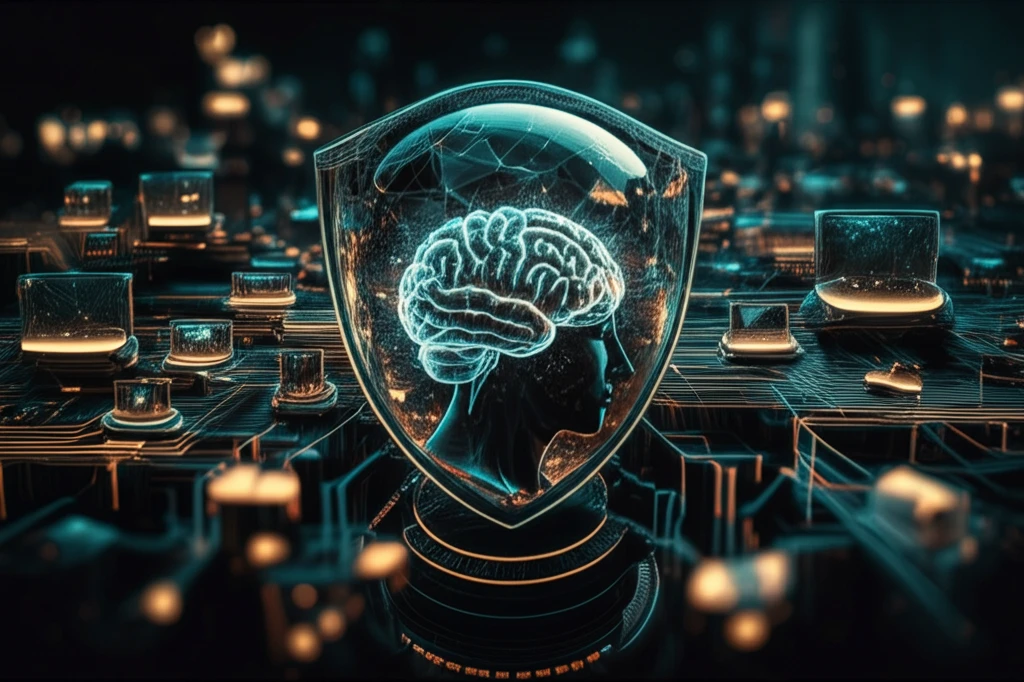
At its heart, federated learning is about bringing the AI model to the data, rather than the other way around. Instead of collecting all the raw data on a central server, a central model is sent out to various participating devices or organizations. Each participant then trains the model using their local data, and the updates (not the data itself) are sent back to the central server. These updates are then aggregated to improve the central model.
- Heterogeneous Data: Data across different sources can vary significantly in terms of format, quality, and distribution. This can make it difficult for the central model to learn effectively.
- Communication Costs: Sending model updates back and forth between the central server and participating devices can be expensive, especially when dealing with large models or slow network connections.
- Security Risks: Although the raw data is protected, the model updates themselves can potentially be vulnerable to attacks. Malicious participants could try to manipulate the updates to compromise the central model or infer sensitive information about other participants' data.
Is Federated Learning Worth the Hype?
Federated learning is still an evolving field, but it holds immense promise for the future of AI. By enabling collaborative model training without compromising data privacy, it has the potential to unlock new possibilities in various industries. While challenges remain, ongoing research and development efforts are paving the way for more robust and practical federated learning systems. As AI becomes increasingly integrated into our lives, federated learning could play a crucial role in ensuring that it is developed and deployed responsibly, ethically, and in a way that benefits everyone.