Drone Control Gets Smarter: How AI is Revolutionizing UAV Technology
"Explore the innovative fusion of artificial intelligence and adaptive control systems enhancing the agility and precision of quadrotor UAVs."
Artificial intelligence (AI) is rapidly changing the landscape of technology, and one of the most exciting applications is in the control of unmanned aerial vehicles (UAVs), commonly known as drones. Traditionally, drones have relied on pre-programmed instructions and basic control systems. However, the integration of AI, particularly neural networks, is enabling drones to perceive their environment, make intelligent decisions, and adapt to unforeseen circumstances.
Adaptive models and online learning, concepts that were once separate under control systems and machine learning, are now converging to create a new era of drone capabilities. This convergence allows for the development of sophisticated control systems that learn and evolve in real-time, improving the performance and reliability of UAVs.
This article explores the application of neural network-based model reference adaptive controllers on quadrotor UAVs. We will delve into the principles behind each design decision, ensuring that the knowledge gained can be applied to other practical applications. Furthermore, we'll look into practical considerations for approaching this exciting intersection of drones and AI, along with potential solutions.
Why Use Neural Networks for Drone Control?
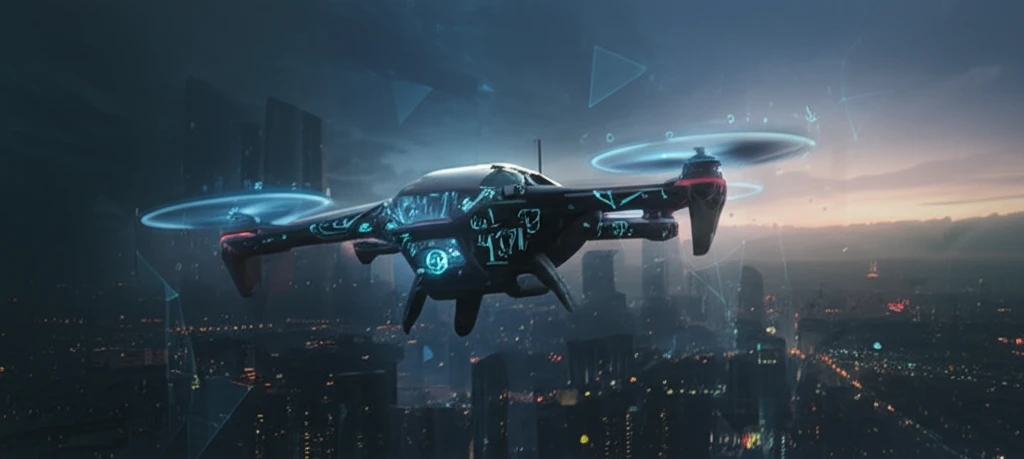
Traditional control systems often struggle when faced with unpredictable environments. Neural networks, with their ability to learn from data and adapt to changing conditions, offer a powerful solution. A key advantage of using neural networks in drone control is their 'black box' nature.
- Adaptability: Neural networks can adjust control strategies in real-time, maintaining stability and meeting objectives even as conditions change.
- Learning Capability: They improve their performance over time through experience, refining control actions based on previous outcomes.
- Versatility: Neural networks can be applied to various drone models and control parameters, offering a flexible solution for different UAV configurations.
The Future of AI-Powered Drones
The integration of neural networks and adaptive control systems is poised to revolutionize drone technology, enabling UAVs to perform more complex tasks in a wider range of environments. As AI algorithms continue to advance and processing power becomes more readily available, we can expect to see even more sophisticated drone applications emerge, transforming industries from agriculture to delivery services.