Decoding the Web: How AI Learns Your Clicks and Predicts Your Next Move
"Uncover how colored Petri nets model user behavior to personalize your online experience and transform web navigation."
In today's vast digital landscape, finding relevant information can feel like searching for a needle in a haystack. Search engines work diligently to address this challenge, but they often rely on generic algorithms that might not cater to your unique interests.
Personalized web experiences aim to solve this problem by tailoring content to your individual preferences. This approach seeks to increase the efficiency of a website, ensuring you quickly find what you need in an optimal state. By analyzing your behavior and data from other users, websites can unlock valuable insights to provide the services, products, and information you're most likely to be interested in.
This article delves into an innovative approach to web personalization using colored Petri nets, a formal modeling technique. This method aims to identify your current interests and recommend the most appropriate pages, creating a more intuitive and engaging online experience.
The Science of Suggestion: How User Behavior is Modeled
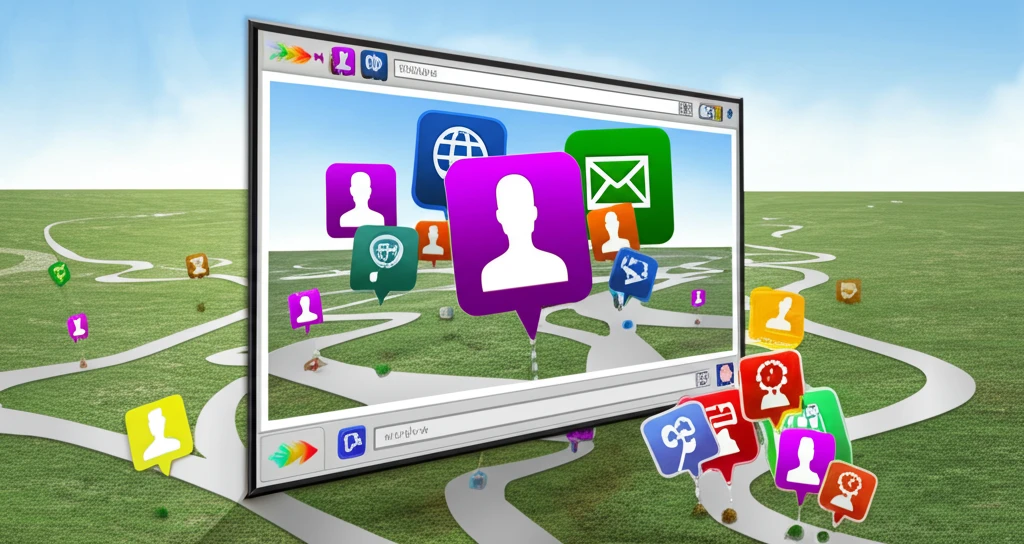
Web personalization systems strive to deliver what you need and like without you even having to ask. This involves providing relevant products, services, and information tailored to your specific preferences. To achieve this, these systems consider three key aspects of your web experience: the content presented, the layout of personal pages, and the overall structure of the website.
- Systems That Use Feedback: Analyzing your direct input and ratings.
- Interest Tracking Systems: Recording your stated interests and statistical information.
- Profile-Based Systems: Extracting interests from your browsing history.
The Future of Web Navigation: Personalization as the Standard
Web personalization is not just a convenience; it's becoming an essential component of online interaction. As AI and modeling techniques like colored Petri nets continue to evolve, the ability to predict and cater to individual user interests will only improve. This means a more intuitive, efficient, and enjoyable online experience for everyone, transforming how we navigate and interact with the digital world.