Decoding the Future: Can AI Predict Real-Time Electricity Prices?
"A new interpretable AI offers a promising approach to probabilistic forecasting of electricity market signals, potentially revolutionizing energy trading and grid management."
Imagine trying to predict the stock market, but instead of stocks, you're dealing with electricity prices that fluctuate wildly based on supply, demand, and even the weather. Accurately forecasting these real-time electricity market signals is crucial for energy companies, grid operators, and even consumers. The more precise the forecast, the better these entities can optimize bidding strategies, manage grid stability, and ultimately, keep the lights on.
Traditional forecasting methods often fall short when it comes to capturing the complexities of the electricity market. These markets are influenced by various factors, from locational marginal prices to interregional price spreads and demand-supply imbalances. The challenge lies in creating a forecasting model that is both accurate and interpretable, allowing stakeholders to understand the 'why' behind the predictions.
Now, a new approach is emerging that leverages the power of generative AI to tackle this forecasting challenge. Dubbed WIAE-GPF (Weak Innovation AutoEncoder-based Generative Probabilistic Forecasting), this innovative architecture aims to provide probabilistic forecasting with a level of interpretability previously unseen in black-box models.
What is WIAE-GPF and How Does It Work?
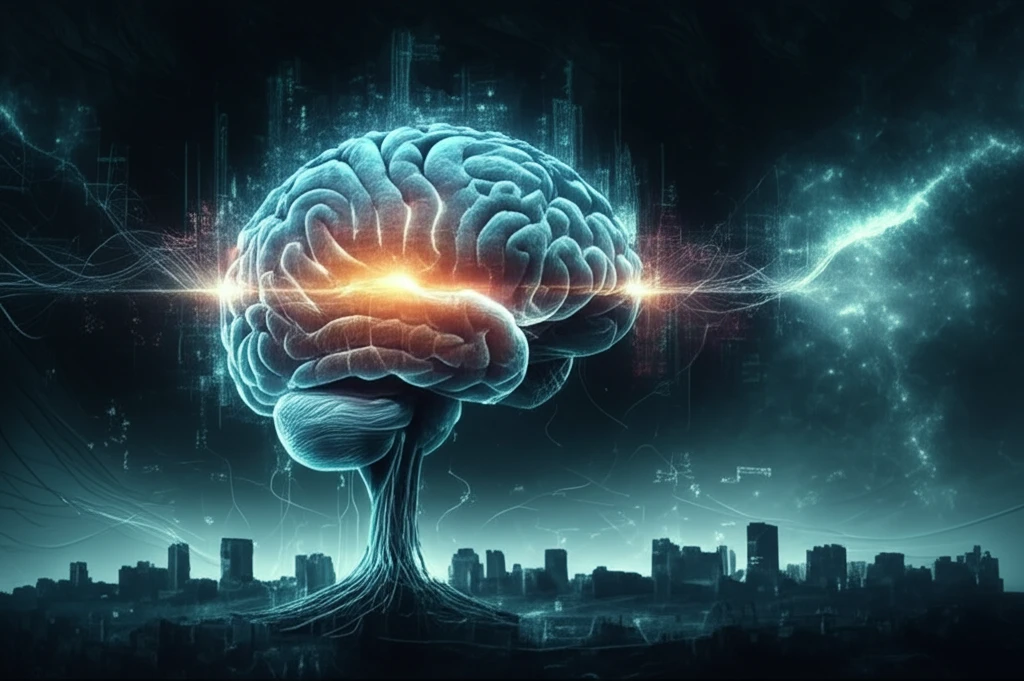
WIAE-GPF represents a significant advancement in electricity market forecasting because it provides a new approach to interpretability. Unlike traditional models that function as black boxes, WIAE-GPF offers insights into how it arrives at its forecasts, opening new paths for more informed decision-making.
- Generative AI Approach: Generates future samples of multivariate time series to predict electricity market signals.
- Weak Innovation AutoEncoder: Uses a unique architecture to capture the underlying patterns in time series data.
- Wiener-Kallianpur Innovation Representation: Offers interpretability by providing a representation for nonparametric time series.
- Structural Convergence Guarantees: Employs a novel learning algorithm, ensuring generated forecast samples match the ground truth conditional probability distribution under ideal training conditions.
The Future of Energy Forecasting is Here
WIAE-GPF offers a glimpse into the future of energy forecasting, where AI not only predicts outcomes but also provides valuable insights into the dynamics of the electricity market. As the energy sector evolves, innovations like WIAE-GPF will play a crucial role in ensuring grid stability, optimizing energy trading, and driving the transition to a more sustainable energy future.