Decoding Obesity: Can Deep Learning Unlock the Secrets in Our Genes?
"A new approach combines genetic studies with AI to predict obesity risk with unprecedented accuracy. Is this the future of personalized health?"
Obesity is a global health crisis, with rates soaring and impacting millions worldwide. The World Health Organization (WHO) recognizes obesity as a major mortality risk factor, contributing to approximately 2.8 million deaths each year. Beyond the human toll, obesity places a huge burden on healthcare systems. In the UK alone, the National Health Service (NHS) spent an estimated £5.1 billion on obesity-related issues in 2006-07.
While lifestyle factors such as diet and exercise play a significant role, genetics also have a huge influence on a person's susceptibility to obesity. Studies on twins and families show that up to 70% of the variation in body mass index (BMI) can be attributed to genetic differences. This means that some people are simply genetically predisposed to gain weight more easily than others.
Genome-wide association studies (GWAS) have emerged as a powerful tool for exploring the genetic basis of complex conditions like obesity. GWAS involve scanning the entire genome for single nucleotide polymorphisms (SNPs), which are variations in a single DNA building block. By analyzing the SNPs of thousands of people, scientists can identify genetic markers associated with an increased risk of obesity.
How Deep Learning Cracks the Genetic Code of Obesity
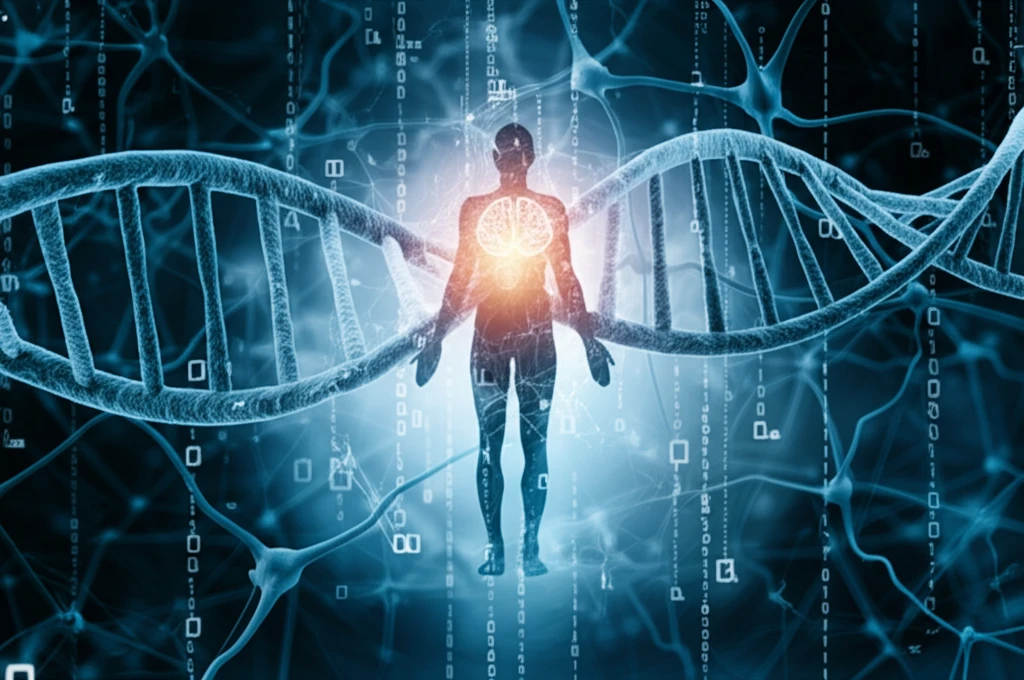
Traditional GWAS methods typically analyze each SNP independently, overlooking the complex interactions between genes. Deep learning, a type of artificial intelligence, offers a new way to analyze this data. Deep learning models can identify intricate patterns and relationships within vast datasets, potentially uncovering the complex interplay of genes that contribute to obesity.
- Data Collection: The study used data from the database of Genotypes and Phenotypes (dbGaP), including participants from the MyCode Community Health Initiative.
- Quality Control: Rigorous quality control measures were applied to the data, including filtering out individuals with inconsistent sex information, removing related or duplicate samples, and excluding SNPs with low minor allele frequency or high missing rates.
- Association Analysis: Statistical association testing was conducted between individual SNPs and obesity using logistic regression, an additive model was employed.
- Deep Learning Implementation: A multi-layer feedforward neural network was implemented, trained using labelled training samples from case-control genetic data. The network's performance was evaluated using metrics like sensitivity, specificity, AUC, Gini coefficient, log loss, and MSE.
The Future of Obesity Prediction and Prevention
These findings suggest that single SNP analysis fails to capture the cumulative effect of less significant variants and their overall contribution to the outcome. The application of GWAS in case control setups have resulted in a plethora of significant genetic variants associated with complex disease phenotypes. However, the predictive capacity of these genetic markers is weak since this approach is based on single-locus analysis, omitting the existence of interactions between loci.