Decoding Malware: How AI Simplifies Threat Analysis for Everyone
"Automated malware analysis is here, making cybersecurity more accessible and efficient for analysts of all skill levels."
In today's digital landscape, malware poses a persistent and evolving threat. The challenge for cybersecurity professionals lies not only in detecting these malicious programs but also in understanding their behavior and impact quickly and accurately. Traditionally, malware analysis has been a time-consuming and complex task, requiring specialized expertise and resources. Security analysts often grapple with massive amounts of data generated by dynamic analysis tools, such as sandboxes, making it difficult to extract meaningful insights efficiently.
Recognizing this challenge, researchers have been exploring ways to automate and streamline the malware analysis process. A promising approach involves leveraging artificial intelligence (AI) to generate human-readable reports that summarize the key findings from sandbox logs and other sources. This technology aims to bridge the gap between raw technical data and actionable intelligence, empowering security analysts to make informed decisions and respond effectively to threats.
One such AI-driven system, known as AMAR-Generator, represents a significant step forward in automated malware analysis. By employing techniques like template matching, API behavior mapping, and malicious behavior databases, AMAR-Generator can produce concise, easy-to-understand reports that describe the malicious activities of malware programs. This innovative approach promises to democratize malware analysis, making it more accessible to a wider range of cybersecurity professionals.
The Power of Automated Analysis
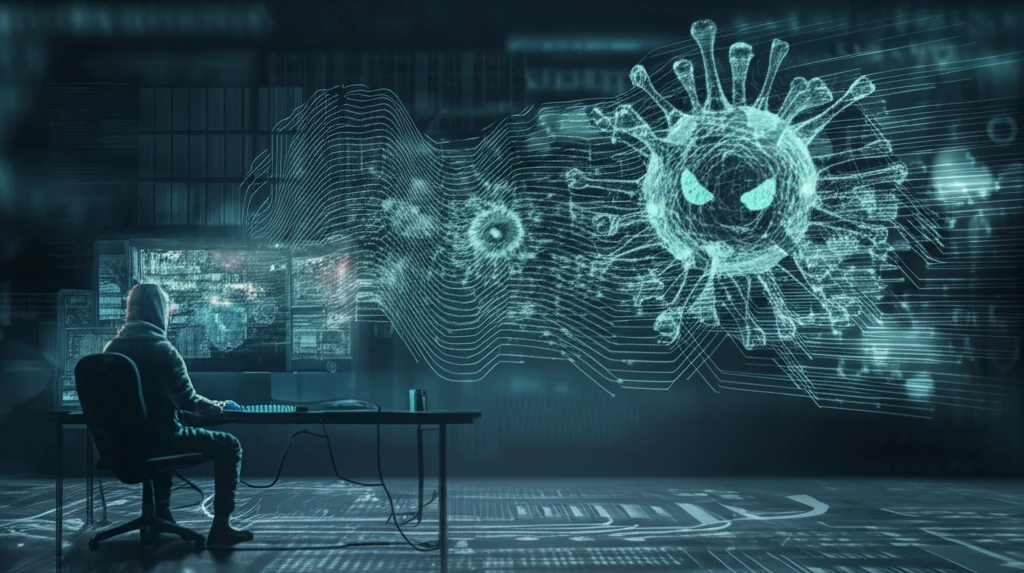
The core strength of AMAR-Generator lies in its ability to interpret and synthesize information from diverse sources. The system leverages vendor reports, which are typically written in natural language, and connects them with the detailed technical data found in sandbox logs. This process involves:
- API Behavior Mapping: API calls and value names from sandbox logs are extracted using an API Behavior Map.
- Behavior Correlation: The malware behavior database confirms whether API call values from sandbox logs are malicious.
- Report Generation: Concise, human-readable reports are produced based on matches in the malware behavior database, using descriptions from vendor reports.
Looking Ahead
While AMAR-Generator represents a significant advancement, there are ongoing efforts to enhance its capabilities. Future research will focus on expanding the types of malicious behaviors the system can detect, improving the accuracy of interpretation, and refining the report generation process. The ultimate goal is to create a comprehensive and user-friendly tool that empowers cybersecurity professionals to stay ahead of evolving malware threats. As AI continues to mature, automated malware analysis will become an increasingly indispensable component of effective cybersecurity strategies.