Decoding Location: Which Agglomeration Estimator Fits Your Needs?
"Navigate the complexities of measuring industrial location with this guide, designed to help researchers choose the right estimator for accurate results."
In the dynamic field of urban economics, industrial agglomeration—the clustering of businesses in specific locations—remains a central topic. This phenomenon drives economic growth and shapes the character of cities, as highlighted in surveys by Rosenthal and Strange (2003), McCann and Folta (2009), and Glaeser (2010). These studies underscore that the concentration of industries fosters the exchange of ideas, labor, and resources, boosting overall productivity and innovation. However, accurately measuring this concentration presents a significant challenge. Choosing the right estimator is crucial for sound analysis, yet the increasing array of options can be daunting.
The need for precise measurement has spurred the development of numerous estimators within urban economics and quantitative geography. These range from simple spatial inequality indices, like the Gini coefficient, to more complex, theoretically grounded measures such as the Ellison-Glaeser index (1997) and point-based measures like those by Marcon and Puech (2003), and Duranton and Overman (2005). Most of these metrics focus on localization, which gauges the extent to which specific industries concentrate spatially relative to the overall concentration of all industries. The core challenge lies in selecting the most appropriate estimator for a given study.
Complicating this choice is the surprisingly low correlation observed between different estimators. For instance, research by Billings and Johnson (2014) and Ellison et al. (2010) reveals correlations below 0.5 between the Ellison-Glaeser (EG) and Duranton-Overman (DO) indices. These indices often yield different results when assessing the determinants of agglomeration. These discrepancies stem from the fact that each estimator captures distinct elements of spatial relationships. While the EG index emphasizes specialization in measuring industry concentration, the DO index focuses on the scale of localization. Given these differences, researchers must carefully weigh the trade-offs between computational simplicity and addressing potential issues like the Modifiable Areal Unit Problem (MAUP).
Navigating the Statistical Minefield: Power and Properties of Agglomeration Estimators
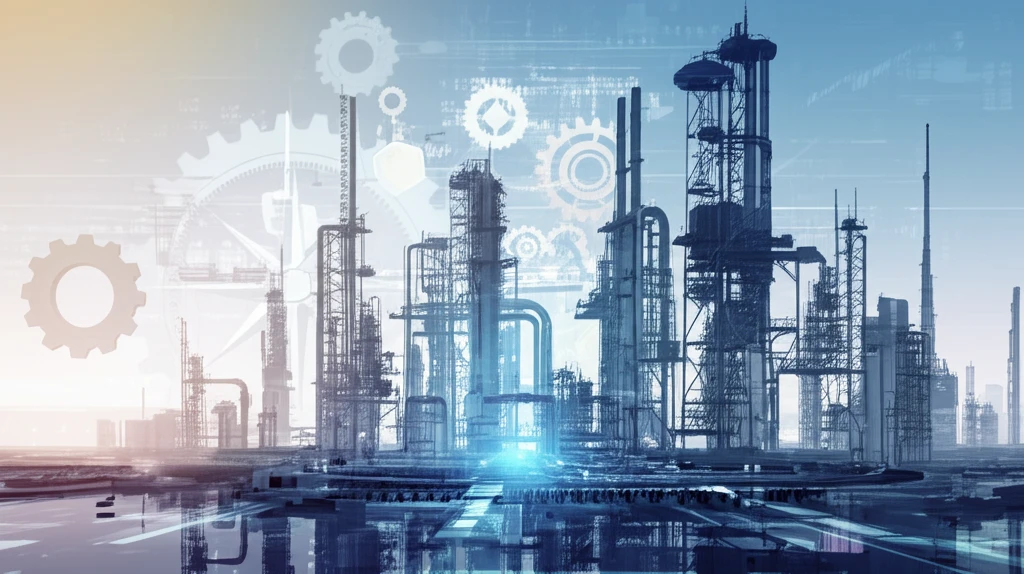
Beyond the fundamental differences in how estimators quantify agglomeration, it’s critical to consider their statistical properties. Conclusions about agglomeration can vary significantly based on an estimator’s statistical power. This article provides a formal assessment of the commonly used Gini coefficient, and the EG and DO indices, evaluating their performance in quantifying industrial agglomeration through a series of simulations using a known data generating process (DGP).
- Finite Sample Bias: Discrete estimators like the Gini index and the EG index exhibit substantial finite sample bias. This means their accuracy can be compromised when dealing with smaller datasets.
- EG Index Caution: Direct comparisons involving the EG index should be made cautiously. The EG index measures spatial dissimilarity, often generating larger values in industries with significant specialization in areas of low commercial density.
- Polycentric Areas: In polycentric study areas (regions with multiple business centers), the statistical properties of estimators show smaller differences. However, continuous estimators generally perform better than discrete ones.
Choosing the Right Tool for the Job
In summary, commonly used estimators of industrial agglomeration vary in how they quantify spatial distributions. Simulation results applied to real-world data confirm concerns regarding small sample sizes and highlight the benefits of continuous measures of space. Moving forward, new methods in machine learning could empower researchers to estimate specific spatial attributes of individual industries. These approaches promise richer insights into spatial relationships than existing summary indices frequently incorporated into empirical research. Ultimately, the key lies in understanding the strengths and limitations of each estimator and selecting the one that best fits the specific research context.