Decoding Fuzzy Clustering: How Deviation-Sparse Methods Enhance Image Analysis
"Explore how deviation-sparse fuzzy c-means clustering improves image segmentation by handling noise and outliers more effectively."
In the realm of image analysis and pattern recognition, clustering stands as a cornerstone technique. Its ability to categorize similar objects into distinct groups has found applications across diverse fields, from medical imaging to remote sensing. Among the various clustering methods available, fuzzy c-means (FCM) has garnered considerable attention for its simplicity and effectiveness.
However, FCM is not without its limitations. Real-world data often contains noise, outliers, and other artifacts that can distort clustering results. Traditional FCM, sensitive to these imperfections, may produce inaccurate or unreliable segmentations. This challenge has spurred the development of numerous modified FCM algorithms aimed at enhancing robustness and accuracy.
This article delves into an innovative approach called Deviation-Sparse Fuzzy C-Means (DSFCM), a method designed to address the shortcomings of traditional FCM by incorporating sparsity and neighbor information constraints. We’ll explore how DSFCM refines clustering through theoretical data estimation and deviation analysis, offering a powerful tool for image processing and analysis.
What Makes Deviation-Sparse Fuzzy C-Means (DSFCM) Different?
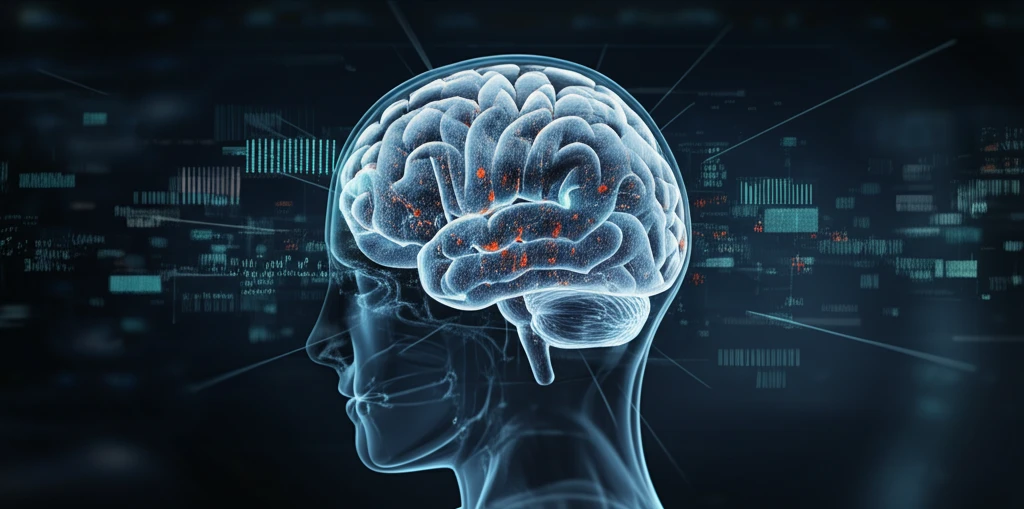
The core innovation behind DSFCM lies in its utilization of theoretical data values, estimated from measured values, within the clustering process. Unlike traditional FCM, which directly uses raw data, DSFCM seeks to refine the clustering by leveraging these theoretical values. By imposing sparsity on the deviations between measured and theoretical values, DSFCM gains the ability to identify and mitigate the impact of noise and outliers.
- Theoretical Value Utilization: Incorporating estimated theoretical values for more accurate clustering.
- Sparsity Implementation: Applying sparsity to identify and handle noise and outliers effectively.
- Neighbor Information Constraint: Enhancing reliability through contextual analysis in spatially correlated data.
The Future of Clustering in Image Analysis
The introduction of Deviation-Sparse Fuzzy C-Means represents a significant step forward in the field of image analysis. By addressing the inherent limitations of traditional FCM and incorporating innovative techniques for handling noise and outliers, DSFCM offers a more reliable and accurate approach to clustering. As technology continues to advance and data becomes increasingly complex, methods like DSFCM will play a crucial role in unlocking valuable insights from visual data. The ongoing research and development in this area promise even more sophisticated and effective clustering solutions, driving advancements in various applications from medical diagnostics to environmental monitoring.