Decoding Doctor's Notes: How AI is Revolutionizing Medical Concept Extraction
"Unlocking Insights from Unstructured Clinical Text with Automated Methods"
Imagine trying to understand complex medical reports filled with jargon and confusing terminology. Medical concept extraction (MCE) aims to solve this problem by automatically identifying and categorizing key information within clinical texts. The i2b2 challenge in 2010 highlighted the importance of this field, focusing on extracting concepts like medical problems, treatments, and tests. Now, innovative approaches are leveraging AI to streamline this process, making healthcare data more accessible and understandable.
Traditional methods for medical concept extraction often rely on rule-based systems. These systems use predefined rules to recognize specific words and phrases, mapping them to standardized medical vocabularies like the Unified Medical Language System (UMLS). While effective to some extent, rule-based approaches can be limited by their rigidity and inability to adapt to the variations in clinical language. This is where the power of AI comes in, offering more flexible and adaptable solutions.
This article explores how AI and machine learning are revolutionizing medical concept extraction. We'll delve into the challenges of extracting information from unstructured clinical text, examine the benefits of AI-driven approaches, and discuss the future implications for healthcare. Whether you're a healthcare professional, a patient, or simply interested in the intersection of AI and medicine, this guide will provide valuable insights into this rapidly evolving field.
Why is Medical Concept Extraction So Difficult?
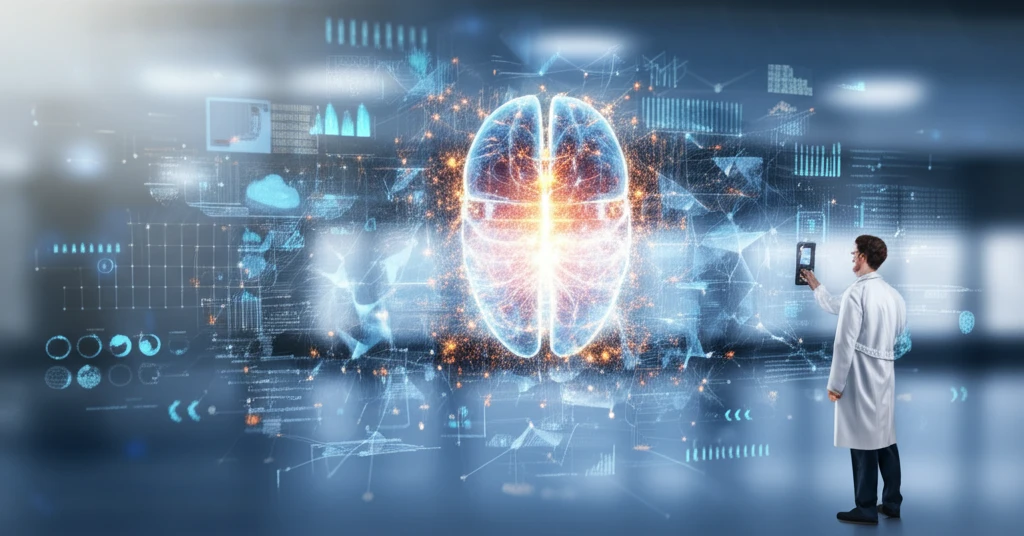
Clinical text is notoriously complex. Doctor's notes, discharge summaries, and other medical documents are often filled with abbreviations, technical terms, and inconsistent formatting. Unlike well-structured data, this unstructured text presents significant challenges for automated analysis. Consider these hurdles:
- Variations in terminology: Doctors may use different terms to describe the same condition or treatment.
- Inconsistent formatting: Clinical notes can vary widely in structure and organization, making it difficult to apply standardized rules.
- The sheer volume of data: The amount of clinical text generated daily is enormous, making manual extraction impractical.
The Future of Medical Concept Extraction: AI-Powered Insights
AI-driven medical concept extraction holds immense potential for transforming healthcare. By automating the extraction of key information from clinical text, AI can improve the accuracy and efficiency of diagnosis, treatment, and research. As AI algorithms become more sophisticated and are trained on larger datasets, we can expect even more impressive advances in the field. This will empower healthcare professionals, improve patient outcomes, and accelerate the pace of medical discovery.