Decoding Climate Change: Can We Predict the Future with Data?
"Unlocking the Secrets of Climate Causality: A Deep Dive into High-Dimensional Analysis for a Sustainable Tomorrow"
For decades, the concept of Granger causality—an approach initially developed to explore causal relationships in economics—has steadily grown into a respected tool for climate scientists. Tests using Granger causality now offer a refined approach to understanding climate dynamics, proving more adept than traditional methods like lagged linear regression. This evolution has led to its broad application across diverse fields within climate science.
However, a significant challenge remains: how to effectively manage the complexity of climate data. Climate models often involve numerous variables, from radiative forcings to global temperatures, each potentially influencing the other in intricate ways. Traditional Granger causality tests, designed for smaller datasets, struggle with this high dimensionality, risking statistical inaccuracies and spurious findings. This limitation necessitates innovative approaches that can handle extensive datasets without compromising the reliability of results.
Recent research introduces advanced statistical techniques to overcome these challenges, allowing for the examination of complex causal chains within climate systems. These methods promise a more nuanced understanding of the interplay between various climate factors, paving the way for more accurate predictions and informed climate policies.
What is High-Dimensional Granger Causality?
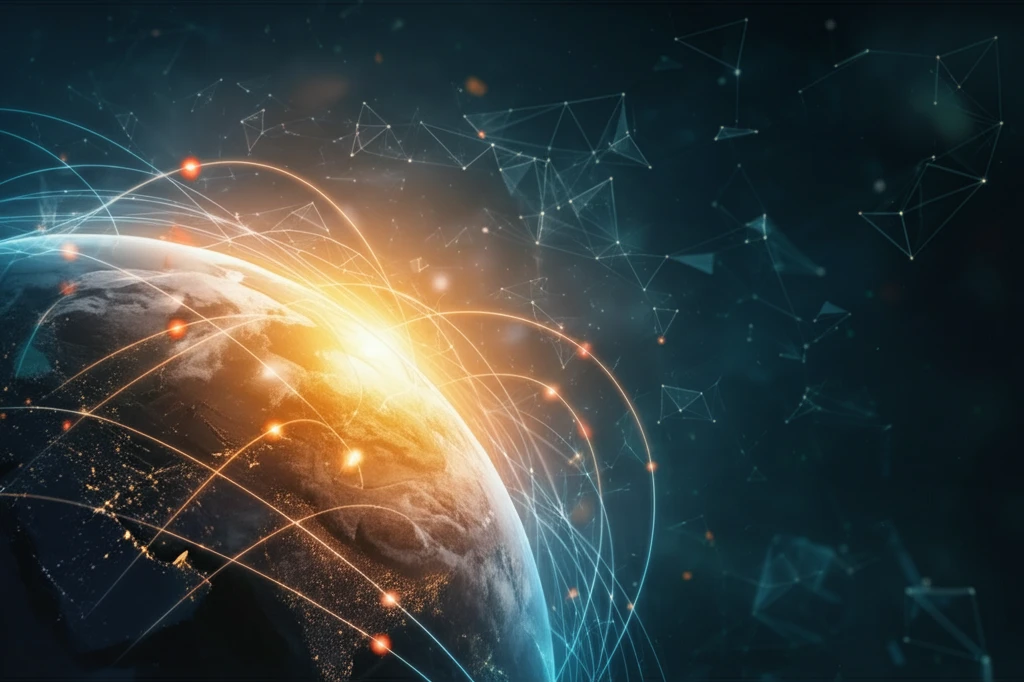
At its core, Granger causality helps determine if one time series can forecast another. In simpler terms, if knowing the past values of variable X improves the prediction of variable Y, then X is said to 'Granger cause' Y. However, this determination is relative. It depends heavily on the information set used—what other variables are considered simultaneously. In climate science, where numerous factors interact, this becomes particularly complex.
- Sparsity Assumption: This assumes that many of the potential relationships between variables are negligible. By focusing on the most significant connections, the complexity of the model is reduced.
- Dimensionality Reduction: Techniques like Lasso regression help to automatically select the most relevant variables, discarding the less influential ones.
- Lag Augmentation: To account for stochastic trends, redundant lags are added to the model, providing an automatic differencing mechanism.
The Future of Climate Prediction
High-dimensional Granger causality and related techniques represent a significant leap forward in our ability to understand and predict climate change. By embracing these advanced analytical tools, researchers can develop more accurate climate models, inform effective climate policies, and ultimately, better prepare for the challenges of a changing world. As climate data continues to grow in volume and complexity, these methods will become increasingly vital in the ongoing effort to secure a sustainable future.