Cerebral Palsy & Activity: Can AI Help Kids Move More?
"New tech offers hope for more accurate, personalized tracking of physical activity in children with cerebral palsy."
Cerebral palsy (CP), the most common physical disability in children, often brings challenges to physical activity. For many kids with CP, staying active can be tough, but it’s incredibly important for their overall health and well-being. That’s where new technology, like machine learning, comes into play, offering innovative ways to track and encourage physical activity.
Traditionally, measuring physical activity in children, including those with CP, has relied on methods like questionnaires or wearable sensors. However, these approaches often fall short. Questionnaires can be subjective and rely on memory, while standard wearable sensors might not accurately capture the nuances of movement in children with CP, potentially underestimating their activity levels.
But what if we could use smart technology to understand exactly how kids with CP move and find ways to help them be more active? Researchers are now exploring machine learning (ML) algorithms to more accurately identify the types and intensity of physical activity in children with CP. This approach could lead to personalized interventions and a better understanding of how to promote healthier, more active lifestyles.
Machine Learning for Accurate Activity Tracking: How Does It Work?
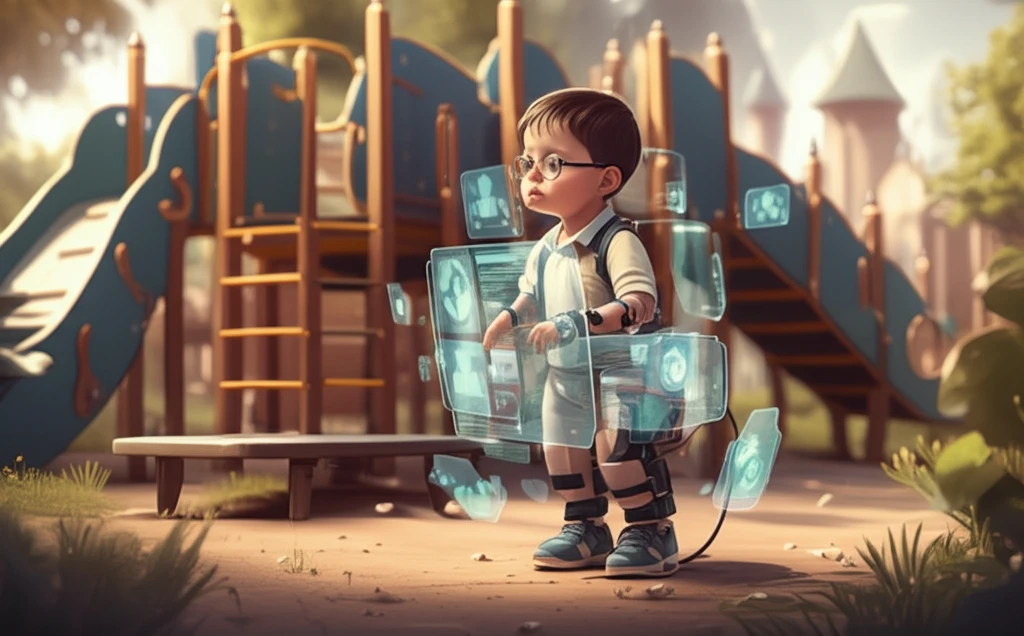
Machine learning steps in to bridge the gap by analyzing data from wearable sensors to accurately classify different types of physical activity. This is especially crucial for children with CP, whose movements may not fit standard activity profiles.
- Data Collection: Researchers used ActiGraph GT3X+ accelerometers to gather movement data from children with CP during structured activities.
- Feature Extraction: The raw data was processed to extract key features, like the intensity and frequency of movements.
- Algorithm Training: Machine learning algorithms like Random Forest (RF), Support Vector Machine (SVM), and Binary Decision Tree (BDT) were trained to recognize different activity types.
- Cross-Validation: The models were tested rigorously to ensure they accurately classified activities across different children.
The Future of Activity Tracking: What Does This Mean for Kids with CP?
The development of machine learning models for activity recognition opens up exciting possibilities for children with CP. By accurately tracking physical activity, these models can help clinicians and families better understand a child’s movement patterns and tailor interventions to promote more active lifestyles. This personalized approach can lead to improved motor skills, increased participation in daily activities, and enhanced overall well-being for children with CP.