Breathe Easier: How Advanced Air Quality Modeling Can Help Us Win the Fight Against Pollution
"A Deep Dive into 3D-Var and Optimal Interpolation Techniques for Aerosol Assimilation Over the U.S."
In our increasingly industrialized world, air pollution continues to pose a significant threat to public health and environmental sustainability. Fine particulate matter, particularly PM2.5, is a major concern due to its ability to penetrate deep into the lungs and bloodstream, causing respiratory and cardiovascular problems. Accurate air quality forecasting is, therefore, essential for implementing effective pollution control measures and protecting vulnerable populations.
For years, organizations like the National Oceanic and Atmospheric Administration (NOAA) have been working tirelessly to improve air quality predictions. The U.S. National Air Quality Forecasting Capability (NAQFC) utilizes complex models to forecast daily ozone and PM2.5 levels. However, these models are not without their limitations, and ongoing research focuses on refining their accuracy and reliability.
One promising area of advancement lies in data assimilation techniques – methods that blend information from both models and observations to create a more accurate representation of the current state of the atmosphere. This article delves into a fascinating case study comparing two such techniques: 3D-Var (three-dimensional variational) and Optimal Interpolation (OI) for aerosol assimilation over the contiguous United States. Understanding these methods is crucial for appreciating the next generation of air quality forecasting.
3D-Var vs. Optimal Interpolation: What’s the Difference and Why Does It Matter?
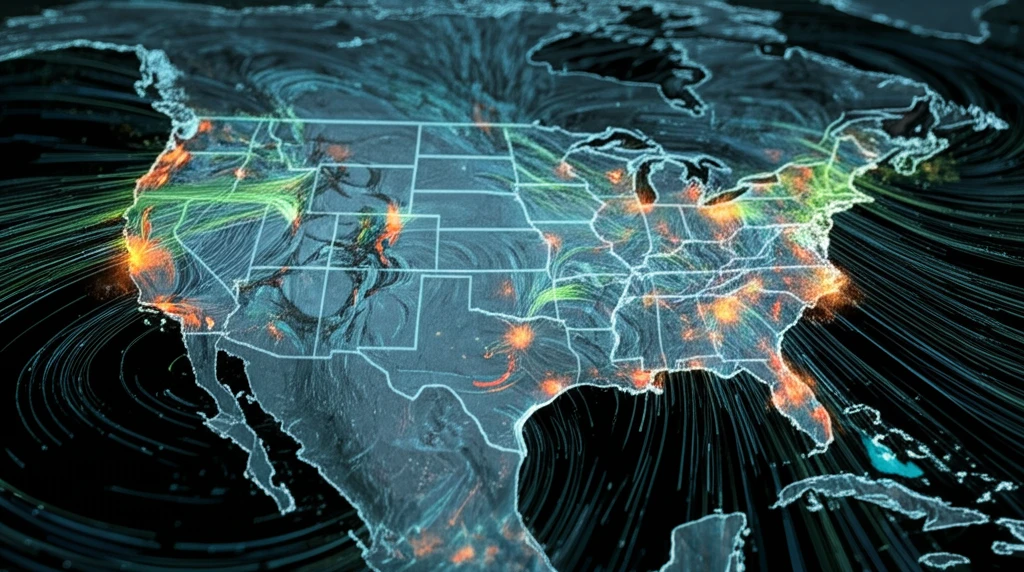
The study focuses on using the Gridpoint Statistical Interpolation (GSI) 3D-Var assimilation tool, originally developed by the National Centers for Environmental Prediction (NCEP), to enhance surface PM2.5 predictions across the United States. This method was compared against the optimal interpolation (OI) method. Both techniques aim to improve the initial conditions of air quality models by incorporating real-world observations.
- Surface PM2.5 vs. AOD Assimilation: GSI experiments showed that assimilating surface PM2.5 data led to more significant increases in surface PM2.5 concentrations compared to assimilating MODIS AOD data. Conversely, MODIS AOD assimilation had a greater impact on surface aerosols at 18 UTC compared to surface PM2.5 assimilation using the OI method.
- Spatial Distribution of Increments: Increments resulting from the OI assimilation are spread across 11x11 horizontal grid cells (12km horizontal resolution). The spatial distribution of GSI increments is controlled by its background error covariances and horizontal/vertical length scales, allowing for a more adaptable and potentially precise correction.
- Impact on Model Biases and Correlation: The study found that both GSI and OI generally helped reduce prediction biases and improve the correlation between model predictions and observations. GSI produces smoother results and yields overall better correlation coefficient and root mean squared error (RMSE).
Looking Ahead: The Future of Air Quality Prediction
This study demonstrates the potential of advanced data assimilation techniques like GSI and OI to improve air quality forecasts. While each method has its strengths and weaknesses, the findings suggest that GSI, with its sophisticated handling of error covariances and length scales, may offer a slight edge in producing smoother and more accurate results. Future research should focus on further refining these methods, incorporating additional data sources, and exploring the use of even more advanced techniques like four-dimensional variational (4D-Var) assimilation to achieve even greater accuracy in air quality predictions.