Brain Tumor Breakthrough: AI-Powered Analysis for Accurate Diagnosis
"Revolutionizing Glioma Grading with 18F-FET-PET and Perfusion MRI Texture Features"
Gliomas, which constitute a significant 80% of all malignant brain tumors, present a considerable challenge in oncology. Accurate classification of these tumors is critical for surgeons and oncologists to formulate effective treatment plans. This is where advanced imaging techniques and artificial intelligence come into play, promising a new era of precision in diagnostics.
Perfusion-weighted MRI (PWI) stands out as an advanced magnetic resonance imaging technique, offering quantifiable hemodynamic parameters that include relative cerebral blood volume (rCBV), cerebral blood flow (rCBF), and mean transit time (MTT). Positron emission tomography (PET) with 18F-FET further aids in delineating cerebral gliomas and differentiating between high- and low-grade tumors. The fusion of these technologies holds potential for significantly improving diagnostic accuracy.
This analysis delves into a study evaluating the use of 18F-FET-PET and perfusion-weighted MRI texture features. By adopting a principal component analysis (PCA), the study aims to refine the classification of high- and low-grade gliomas. PCA helps to reduce the number of features needed, addressing the common challenge of limited patient data in this specialized area of research.
Decoding the Study: How AI Analyzes Brain Tumor Images
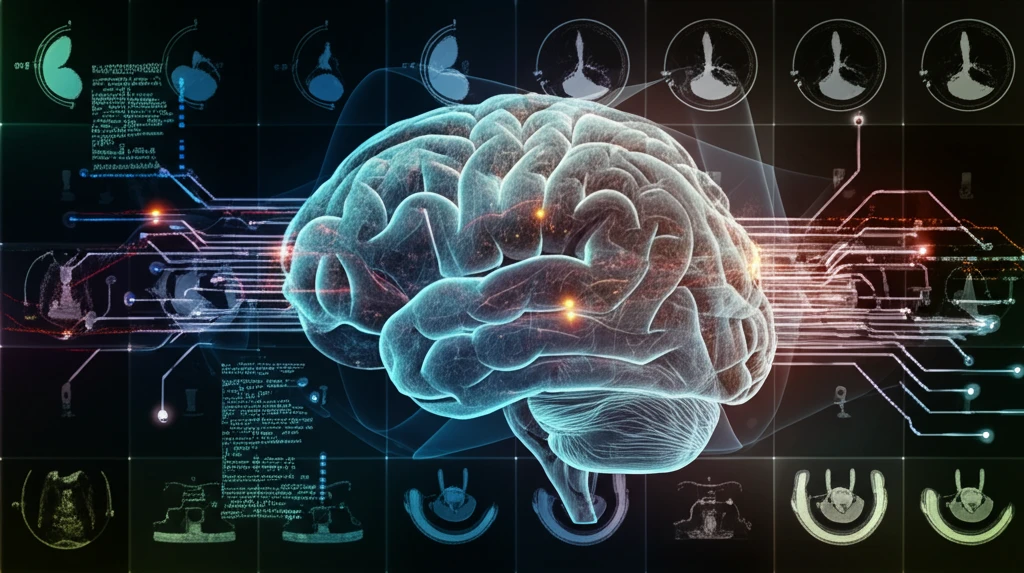
The study, conducted between 2012 and 2016, involved 27 patients diagnosed with brain tumors—18 with high-grade gliomas and 9 with low-grade gliomas. These patients underwent both FET-PET and perfusion MRI imaging. The MRI imaging was performed using a Siemens 3T Tim Trio Scanner, part of a hybrid 3TMR-BrainPET setup. Dynamic susceptibility-weighted contrast-enhanced T2-MRI acquisition was performed during the first pass of a contrast agent, using echo-planar imaging (EPI).
- Image Co-registration: Aligning PET and MRI images for accurate comparison.
- VOI Creation: Delineating tumor regions based on FET-PET uptake.
- Normalization: Standardizing perfusion maps using PMOD software.
- Texture Feature Extraction: Analyzing gray-level patterns within VOIs using various matrices.
The Future of Brain Tumor Diagnostics
This study illustrates how texture feature analysis, combined with PCA, can significantly reduce the number of features required for 18F-FET PET and MRI data analysis. By reducing the feature set to one-fourth of the original size, PCA streamlines the analytical process and enhances the focus on the most critical data. This approach not only makes the analysis more manageable but also sets the stage for more accurate and efficient diagnostic models. As data collection expands and analytical techniques advance, the potential for creating predictive models capable of classifying gliomas with greater precision becomes increasingly viable, heralding a new era in personalized cancer treatment.