Beyond the Bottom Line: Understanding Poverty Trends in Australia
"A new study challenges traditional methods of poverty measurement, revealing how nonresponse bias and data limitations can skew our understanding of economic well-being in Australian households."
For years, policymakers and researchers have relied on standard survey data to track poverty and inform economic strategies. However, these methods often overlook critical factors like incomplete datasets and complex survey designs, potentially leading to inaccurate conclusions about the true state of economic well-being. A recent study is changing the narrative by introducing an innovative framework that addresses these limitations, offering a more nuanced understanding of poverty trends, specifically focusing on Australian households.
Traditional approaches to stochastic dominance testing, a common method for comparing income distributions, typically assume complete datasets and rely on simple random sampling or time-series data. In reality, socioeconomic surveys like the Household, Income and Labour Dynamics in Australia (HILDA) Survey are complex, with significant nonresponse rates and intricate designs involving stages, clustering, and stratification. These complexities can skew results, making it difficult to draw reliable conclusions about poverty trends.
Enter a new methodology that integrates partial identification techniques with design-based inference, providing a unifying approach to stochastic dominance testing under survey nonresponse. This method not only accommodates a wide range of nonresponse assumptions but also offers a transparent way to assess the sensitivity of poverty comparisons, ensuring that policy decisions are based on the most accurate and comprehensive data available.
Why Traditional Poverty Measures Fall Short?
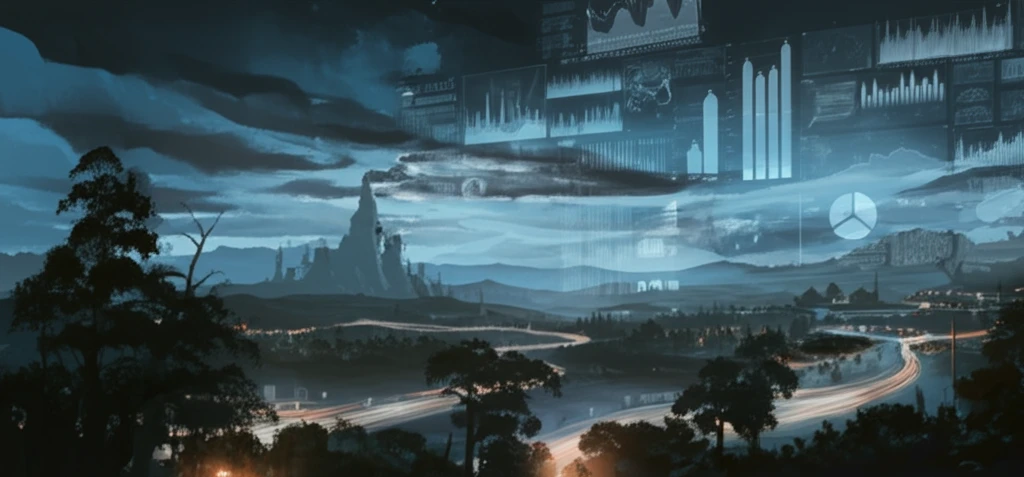
Traditional methods often assume complete datasets or make overly simplistic assumptions about nonresponse, which can lead to skewed results. For instance, surveys often underrepresent high-income individuals, meaning that standard analyses might not accurately capture the economic experiences of the wealthiest, potentially biasing poverty assessments.
- Incomplete data: Most methods assume complete datasets, leading to bias when respondents are missing.
- Simple random sampling: Reliance on random sampling ignores complex survey designs.
- Nonresponse bias: Assumptions about nonresponse are point-identifying or assume specific models, which may not hold true.
- Design complexities: Ignoring survey design aspects can distort standard error estimations and test outcomes.
A Clearer Lens on Poverty
By integrating partial identification, design-based inference, and sensitivity analysis, this new framework provides a more accurate and transparent way to compare poverty levels over time. The result is a more nuanced, data-driven approach to understanding economic hardship in Australia, offering valuable insights for policymakers and researchers alike.