AI's Next Frontier: How Singularity-Aware Learning Could Revolutionize Decision-Making
"Forget perfect data: A new approach to reinforcement learning tackles real-world messiness and opens doors for AI in complex, unpredictable environments."
Imagine teaching a robot to navigate a bustling city street. Existing artificial intelligence (AI) methods would require the robot to experience every possible scenario – every pothole, pedestrian, and unpredictable traffic pattern – before it can make informed decisions. This reliance on complete information is a major roadblock, limiting AI's potential in dynamic, real-world situations where data is often incomplete, biased, or just plain messy.
But what if AI could learn to make smart choices even when faced with the unknown? That's the promise of a groundbreaking approach called Singularity-Aware Reinforcement Learning (STEEL). Unlike traditional methods that demand perfect data, STEEL embraces the 'singularities' – those gaps and inconsistencies that make real-world data so challenging. This innovative technique is poised to unlock new possibilities for AI in fields ranging from healthcare to finance.
This article breaks down the core concepts of STEEL, explores its potential impact, and examines why this new approach could be the key to unlocking AI's next level of intelligence and adaptability. We'll explore how STEEL addresses a fundamental problem in AI and what that could mean for the future of automated decision-making.
What is Singularity-Aware Reinforcement Learning (STEEL)?
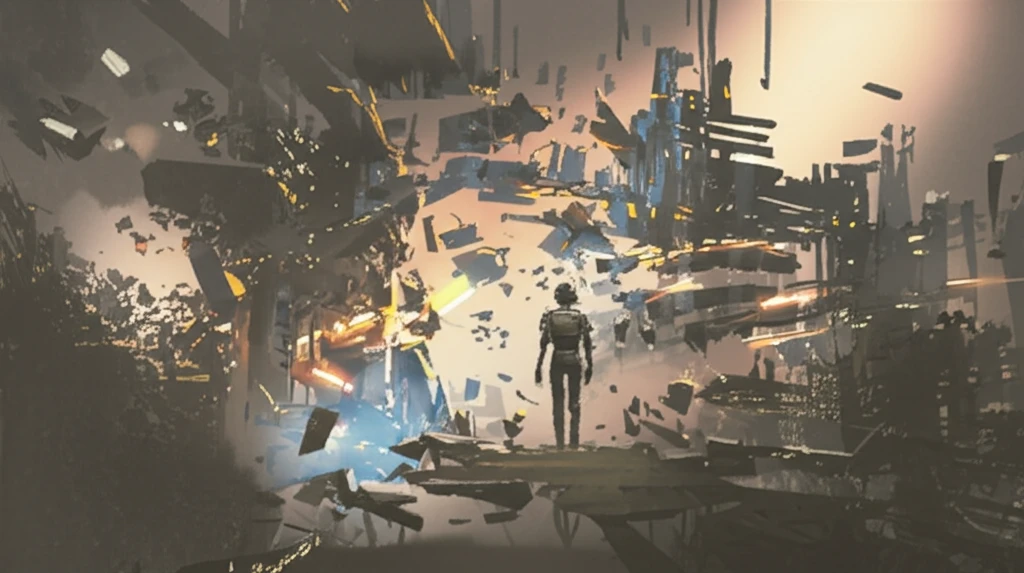
At its heart, STEEL is a type of reinforcement learning (RL) designed to function effectively even when the data used for training is incomplete. Reinforcement learning is a method where an AI agent learns to make decisions by trial and error, receiving rewards or penalties for its actions. Think of it like teaching a dog tricks – positive reinforcement (treats) encourages desired behaviors.
- Incomplete Data: Real-world datasets rarely capture every possible scenario.
- Distributional Mismatch: The data used for training might not accurately reflect the situations the AI will encounter in the real world.
- Non-Overlapping Regions: Situations where the AI encounters entirely new experiences not represented in the training data.
STEEL: A More Intelligent Future?
Singularity-Aware Reinforcement Learning represents a significant step forward in AI's ability to tackle complex, real-world problems. By embracing uncertainty and learning to make informed decisions even with incomplete data, STEEL opens the door to a new era of AI applications that are more robust, adaptable, and ultimately, more intelligent. As AI continues to evolve, techniques like STEEL will be essential for unlocking its full potential and creating systems that can truly thrive in the messy, unpredictable world around us.