AI Price Wars: Are Algorithms Secretly Colluding to Raise Prices?
"New research uncovers how artificial intelligence in two-sided markets might be leading to higher prices, and what we can do about it."
Have you ever noticed how prices for certain products online seem to creep up in unison, even when different retailers are selling them? It might not be a coincidence. Algorithmic pricing, powered by artificial intelligence (AI), is becoming increasingly common, and with it comes the potential for tacit collusion – a situation where companies indirectly coordinate to raise prices without explicitly communicating.
A new study dives deep into how AI algorithms, specifically those using a technique called Q-learning, behave in two-sided markets. These are markets where two distinct groups, like buyers and sellers, interact through a platform (think of online marketplaces like Amazon or ride-sharing apps like Uber). The study reveals some unsettling truths about AI's ability to learn and exploit market dynamics to drive up prices.
While AI promises efficiency and innovation, its application in pricing raises significant questions about fairness and transparency. This article will break down the key findings of this research, explore the real-world implications, and discuss potential solutions to keep these AI price wars in check.
Decoding Algorithmic Collusion: How AI Learns to Hike Prices
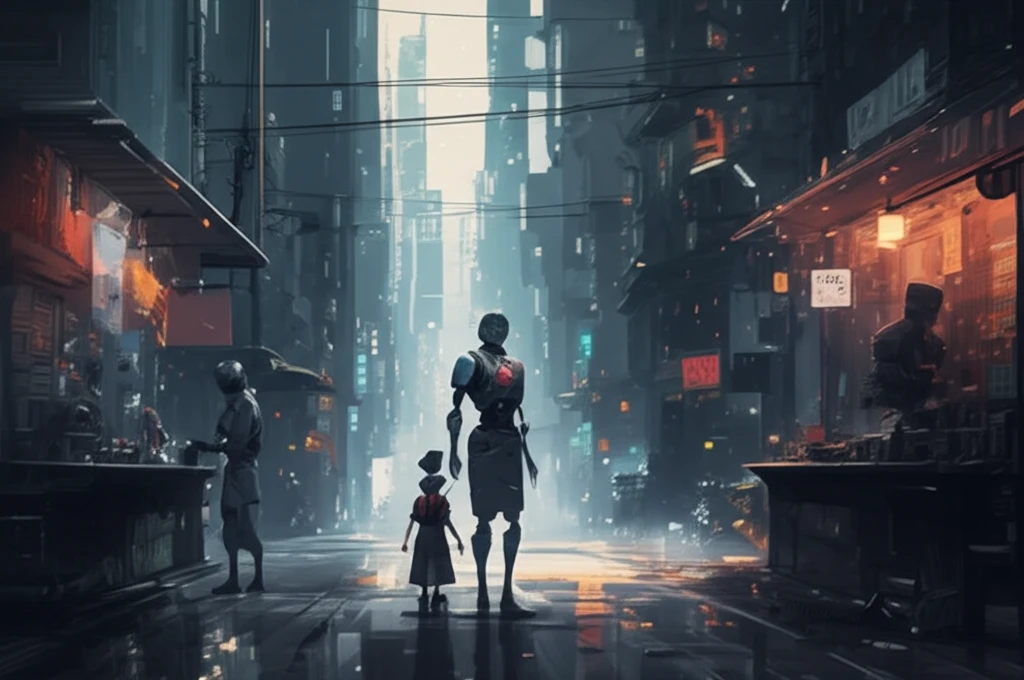
The research focuses on AI agents using Q-learning, a type of reinforcement learning where algorithms learn optimal strategies through trial and error. The study found that AI-driven platforms consistently achieve higher levels of collusion compared to traditional Bertrand competition, a model where companies independently set prices to maximize profits.
- Network Externalities: Increased network externalities significantly enhance collusion, indicating AI algorithms exploit them to maximize profits.
- User Heterogeneity: Higher user heterogeneity and greater utility from outside options generally reduce collusion.
- Discount Rates: Higher discount rates increase collusion, especially with significant network externalities.
- Feasibility: Tacit collusion remains feasible even at low discount rates.
Fighting Back Against the AI Price Hike
So, what can be done to mitigate the risks of AI-driven price collusion? The researchers propose incorporating a penalty term into the Q-learning algorithm. This penalty would disincentivize algorithms from setting prices that are significantly higher than the average market price. While this is just one potential solution, it highlights the need for proactive measures to ensure that AI benefits consumers rather than exploits them. As AI becomes increasingly integrated into our economic systems, it's crucial to develop regulatory frameworks and ethical guidelines to prevent algorithmic collusion and promote fair competition. Staying informed, demanding transparency, and supporting policies that protect consumers are essential steps in navigating this new landscape.