AI Overdrive: How Algorithms Are Rewriting the Rules of Decision-Making & What It Means for You
"Uncover the hidden biases in algorithmic recommendations and learn how to navigate the future of human-AI collaboration for smarter choices."
Imagine you're a judge facing a difficult decision about whether to grant bail to a defendant. You receive a risk assessment from an algorithm suggesting the defendant is high-risk. How does this information affect your decision? Traditionally, we might assume it simply provides an objective data point to consider. However, recent research suggests that algorithmic recommendations can do more than just inform—they can subtly alter our preferences.
A groundbreaking study from Stanford University sheds light on this phenomenon, revealing how algorithmic assistance can create "recommendation-dependent preferences." This means that the way an algorithm presents information can unintentionally bias decision-makers, leading them to over-rely on the AI's suggestions, even when it contradicts their own judgment.
This article explores the fascinating world of algorithmic influence, drawing insights from the Stanford study and other cutting-edge research. We'll uncover how algorithms are reshaping human decision-making, discuss the potential pitfalls of recommendation-dependent preferences, and explore strategies for navigating the age of AI with greater awareness and control. Whether you're a business leader, a healthcare professional, or simply someone curious about the impact of AI, this is your guide to making smarter choices in an increasingly algorithmic world.
The Algorithm as a Silent Persuader: How Recommendations Change Your Mind
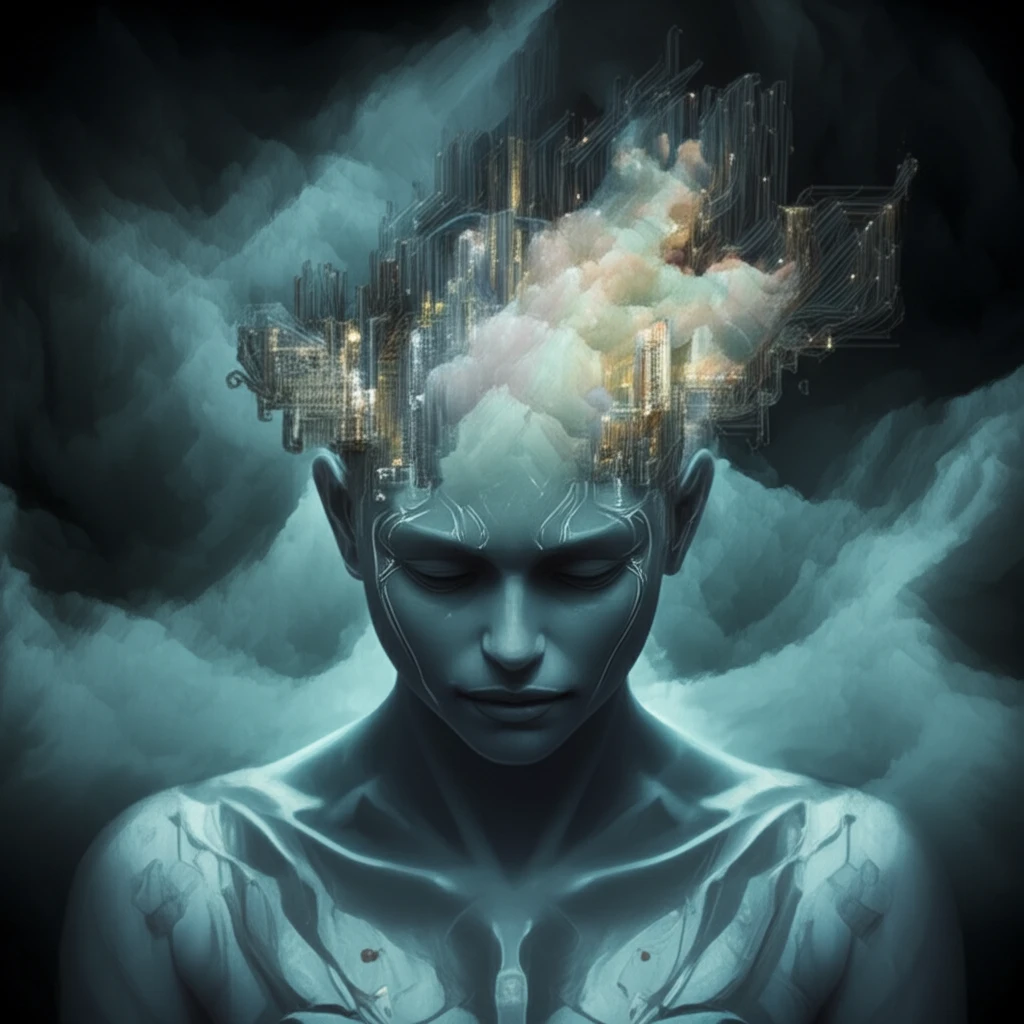
The Stanford study introduces a principal-agent model to explain how algorithmic recommendations can subtly shift our preferences. In this model, the "principal" designs the algorithm, while the "agent" is the human decision-maker. The agent has to choose between a "safe" and a "risky" decision, based on their own private information and a recommendation from the algorithm. The key insight? The algorithm's recommendation doesn't just provide information; it also acts as a reference point, influencing how the agent perceives the potential outcomes.
- Institutional Factors: Imagine a judge who is hesitant to go against a recommendation to jail a defendant out of fear of public or professional backlash.
- Behavioral Science: In medical field, a doctor could be hesitant to not prescribe a test that shows up as 'Recommended'.
- Loss Aversion: People weigh potential losses more heavily than equivalent gains, so if an algorithm suggests a course of action, deviations feel riskier than they might be.
Steering Clear of Algorithmic Bias: Taking Control of Your Choices
The rise of AI in decision-making is inevitable. However, by understanding how algorithms can subtly influence our preferences, we can take steps to mitigate their potential biases. Here are a few key strategies for navigating the age of AI with greater awareness and control: Recognize the Potential for Bias, Seek Diverse Perspectives, Focus on the "Why" Behind Recommendations, Prioritize Transparency and Accountability, Embrace Human Oversight and promote continuous evaluation