AI Decision-Making Under Scrutiny: Unveiling Critical Corrections and Ensuring Accuracy
"Dive into the essential updates in AI decision modeling, addressing critical revisions for reliable and ethical applications."
In the rapidly evolving landscape of Artificial Intelligence (AI), the accuracy and reliability of decision-making models are paramount. AI systems are increasingly integrated into critical sectors such as healthcare, finance, and transportation, where their decisions can have profound consequences. Therefore, any inaccuracies or errors in these models must be promptly identified and corrected to ensure ethical and effective deployment.
The original publication, 'Modeling Decisions for Artificial Intelligence,' aimed to provide a comprehensive framework for AI decision-making. However, like any pioneering work, it was subject to revisions and refinements as the field advanced. The authors identified certain areas, particularly within the chapters on 'Graded Logic Aggregation' and 'Implicative Weights as Importance Quantifiers in Evaluation Criteria,' that required correction to enhance the model's precision and applicability.
This article delves into the essential corrections made to 'Modeling Decisions for Artificial Intelligence,' highlighting the significance of these revisions in ensuring the accuracy and reliability of AI decision models. We will explore the specific areas that were addressed, the rationale behind the corrections, and the implications for AI practitioners and researchers. Understanding these updates is crucial for anyone working in AI, as it directly impacts the validity and trustworthiness of AI-driven decisions.
Why Accurate AI Models are Essential for Reliable Decision-Making
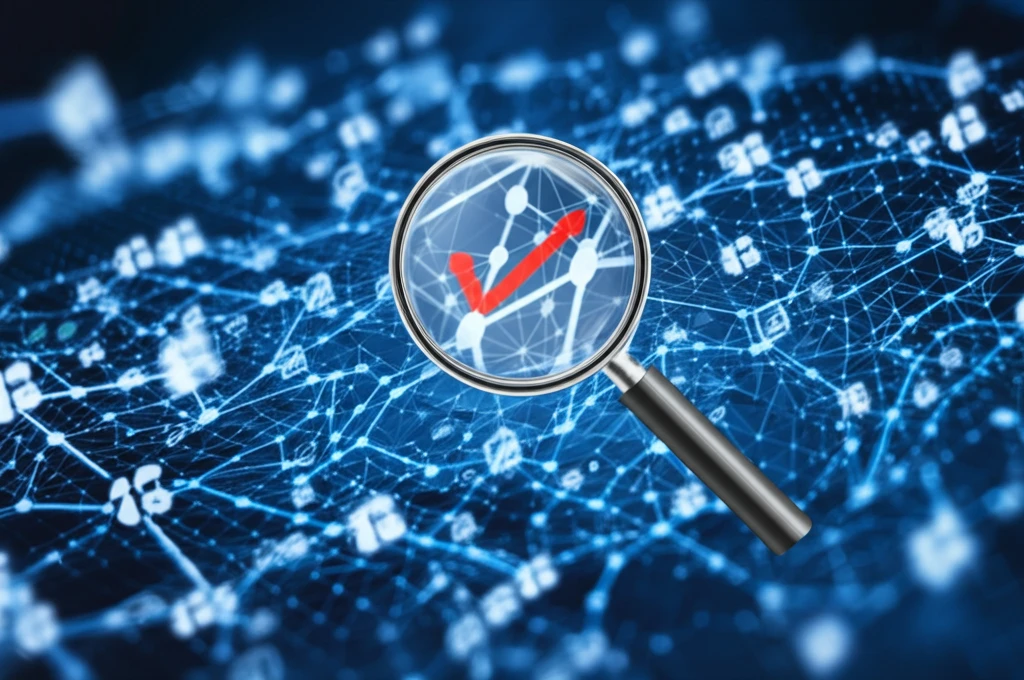
AI decision-making models are designed to mimic human cognitive processes, enabling machines to analyze data, identify patterns, and make informed decisions. However, unlike human decision-makers, AI systems rely entirely on the data and algorithms they are trained on. If the underlying models contain errors, the resulting decisions can be flawed, leading to undesirable outcomes.
- Healthcare: Incorrect diagnoses or treatment recommendations.
- Finance: Flawed risk assessments or investment decisions.
- Transportation: Erroneous routing or autonomous driving errors.
- Criminal Justice: Biased predictions or unfair sentencing.
- Human Resources: Unfair hiring processes or discrimination.
The Path Forward: Continuous Improvement in AI Decision-Making
The corrections to 'Modeling Decisions for Artificial Intelligence' underscore the importance of ongoing refinement and validation in the field of AI. As AI systems become more complex and pervasive, it is crucial to prioritize accuracy, reliability, and ethical considerations. By addressing errors and biases in AI models, we can ensure that these technologies are used responsibly and for the benefit of society. The journey towards reliable AI decision-making is a continuous one, requiring collaboration, vigilance, and a commitment to excellence.