Unveiling the Clarity: Deep Learning Revolutionizes Fringe Pattern Denoising
"A breakthrough approach to image processing promises sharper insights in optical measurement, now within everyone's reach."
In an era where image clarity is paramount, especially in scientific and industrial applications, the ability to remove noise from fringe patterns is crucial. Fringe patterns are visual representations of data in fields like optical metrology, where precision is key. However, these patterns are often marred by noise, which obscures the underlying information and complicates analysis. Traditional methods of denoising, while effective to some extent, often fall short when dealing with complex or large datasets.
Now, a groundbreaking solution has emerged, harnessing the power of deep learning to revolutionize fringe pattern denoising. This innovative approach promises not only to enhance the clarity of these critical images but also to streamline the entire process, making it more accessible and efficient for researchers and industry professionals alike. As deep learning continues to make waves across various sectors, its application to optical measurement marks a significant leap forward.
This article explores the intricacies of this deep learning-based denoising technique, its advantages over conventional methods, and its potential impact on fields ranging from manufacturing to medical imaging. Join us as we delve into how this technology works, why it matters, and what the future holds for this exciting application of artificial intelligence.
The Deep Learning Difference
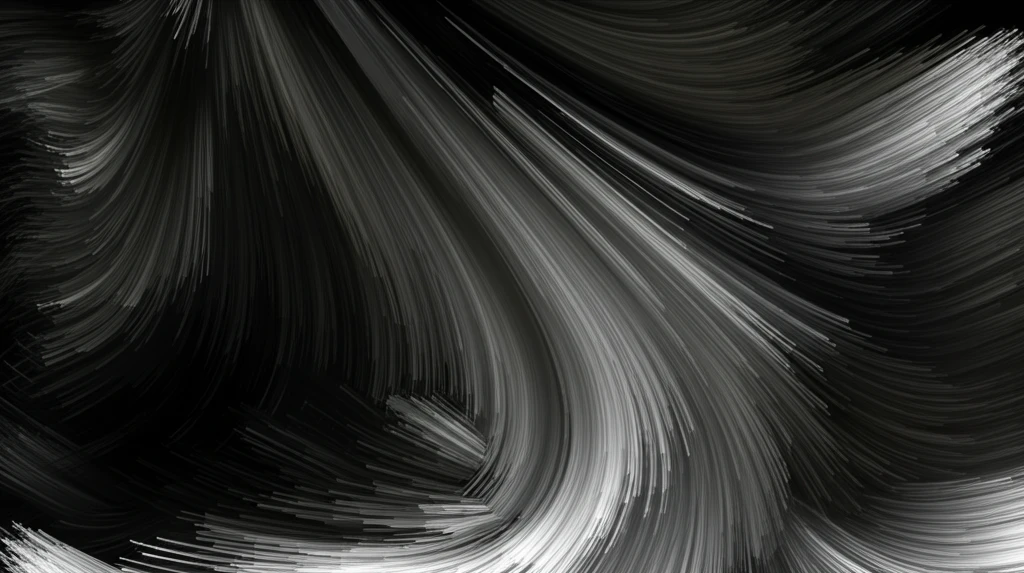
Deep learning, a subset of machine learning, excels at identifying intricate patterns in data. This capability makes it exceptionally well-suited for denoising fringe patterns, where noise can manifest in various complex forms. Unlike traditional algorithms that require manual tuning and specific assumptions about the noise, deep learning models can learn directly from the data, adapting to different types of noise and pattern variations.
- Superior Noise Reduction: DCNNs can handle complex and variable noise more effectively than traditional methods.
- Increased Efficiency: Once trained, the DCNN can denoise fringe patterns much faster than iterative algorithms.
- Automated Process: The DCNN learns from data, reducing the need for manual parameter tuning.
- Broad Applicability: The trained DCNN can be applied to various types of fringe patterns, making it a versatile tool.
The Future of Clarity
The application of deep learning to fringe pattern denoising represents a significant advancement in optical measurement. By providing a more accurate, efficient, and automated means of noise reduction, this technology opens up new possibilities for researchers and industry professionals. As deep learning models continue to evolve and datasets grow, we can expect even greater improvements in the clarity and quality of fringe patterns, paving the way for new discoveries and innovations across various fields.