Unmasking Hidden Biases: How to Get Real Treatment Effect Estimates From Subgroups
"Dive into the world of clinical trials and discover the secrets to achieving a balanced and unbiased view of treatment effects across different patient subgroups."
In the ever-evolving landscape of medical research and clinical trials, pinpointing treatment effects within specific subgroups has become increasingly vital. From oncology to diabetes, understanding how treatments affect different patient populations can lead to more personalized and effective healthcare strategies. However, this pursuit is fraught with challenges, one of the most significant being selection bias.
Selection bias occurs when the focus is disproportionately on the most extreme or favorable results from a subset of a larger group. This can lead to exaggerated claims about treatment efficacy and a skewed perception of the true benefits. The issue, often dubbed a “reproducibility crisis,” undermines the reliability of research findings and can mislead both healthcare professionals and patients.
To combat this, innovative statistical methodologies are needed. This article delves into the critical methods for adjusting treatment effect estimates in clinical trials, ensuring a balanced and unbiased view across various patient subgroups. By constructing simultaneous confidence intervals and comparing them with shrinkage estimates from Bayesian hierarchical models, researchers can achieve a more realistic assessment of treatment outcomes.
The Core of the Problem: Selection Bias Explained
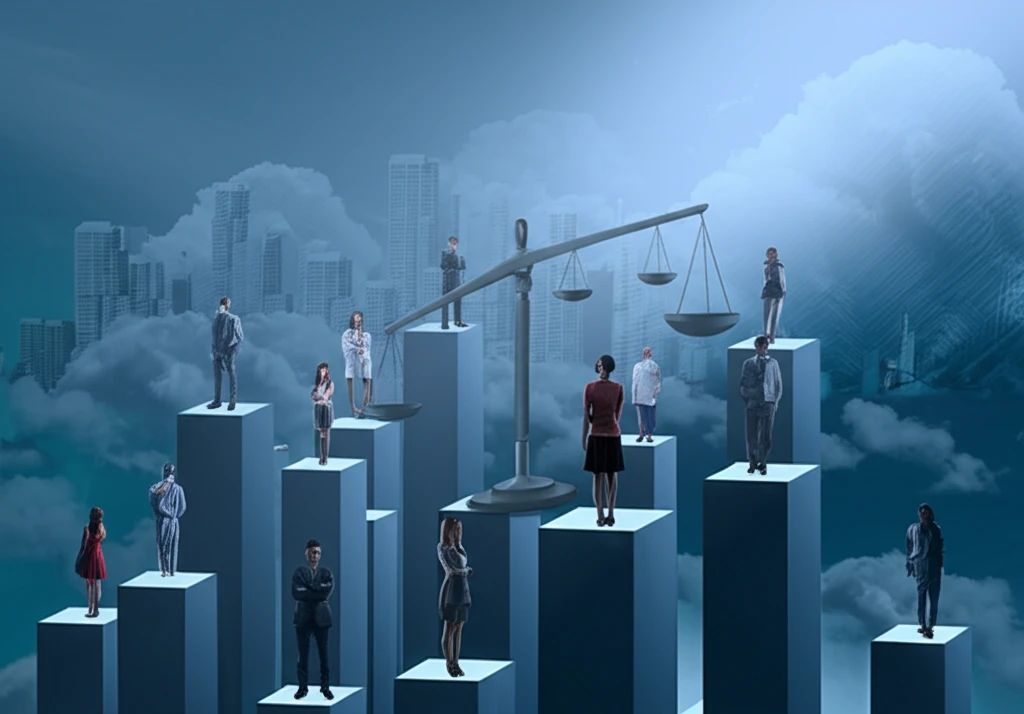
Selection bias arises when the process of choosing which results to highlight or report is not random but influenced by the magnitude or direction of the effect. Imagine a clinical trial examining the impact of a new drug across multiple genetic profiles. If researchers selectively emphasize the results from the subgroup showing the most significant positive response, the overall efficacy of the drug might be overstated. This distortion can have serious implications, leading to inappropriate treatment decisions and resource allocation.
- Frequentist Methods: Pioneering work in this area focuses on unbiased point estimation for selected means, offering a way to correct for the overemphasis on extreme values.
- Interval Estimation: These methods consider interval estimation of selected effects, providing a range within which the true effect is likely to lie, rather than a single, potentially biased, point estimate.
- Genomic Applications: Tailored approaches address the unique challenges of genomics, where the number of subgroups can be vast, and only a small subset may be truly relevant.
Navigating the Path Forward
In conclusion, while subgroup analysis offers the promise of personalized medicine, it also brings the risk of selection bias. By understanding and applying the methods discussed, researchers and healthcare professionals can better navigate the complexities of clinical trial data, ensuring that decisions are based on reliable and unbiased information. Embracing these strategies leads to more informed choices, benefiting both individual patients and the broader healthcare landscape.