Unlocking Diffusion: How New Math Can Smooth Out Tech and Nature's Toughest Problems
"Innovative 'Quasi-Toeplitz Splitting' Methods Offer a Leap Forward in Modeling Unsteady Diffusion Across Diverse Fields"
Imagine trying to predict how pollutants spread in the air, how a new drug will distribute through the body, or how heat dissipates in a high-tech device. All these scenarios, seemingly disparate, are governed by a common phenomenon: diffusion. Understanding and accurately modeling diffusion processes is crucial in numerous fields, but it becomes incredibly challenging when dealing with complex environments and variable conditions.
Traditional diffusion models often fall short when applied to heterogeneous or memory-dependent systems. This is where fractional diffusion models come into play, offering a more nuanced and accurate description. However, these advanced models require sophisticated mathematical tools to solve, pushing the boundaries of computational power and algorithmic efficiency.
Now, a new breakthrough offers a promising path forward. Researchers have developed a class of 'Quasi-Toeplitz splitting iteration methods' designed to tackle unsteady space-fractional diffusion equations. This innovation promises to significantly reduce the computational burden while maintaining accuracy, potentially revolutionizing simulations across various scientific and engineering domains.
The Power of Quasi-Toeplitz Splitting: Simplifying Complexity
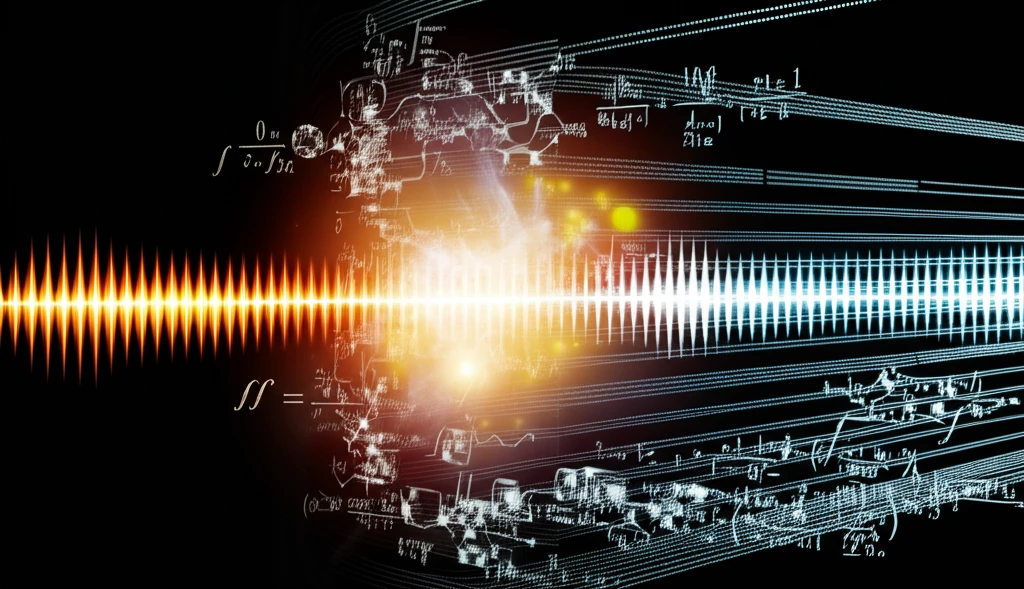
At the heart of this advancement lies a clever mathematical trick: exploiting the structural characteristics of the coefficient matrix in the equations. This approach drastically reduces computational costs, scaling at O(n log n) with 'n' degrees of freedom. This efficiency stems from using a 'circulant matrix' as a preconditioner, streamlining calculations without sacrificing precision.
- Enhanced Accuracy: Fractional diffusion models provide a more realistic representation of diffusion in complex systems.
- Computational Efficiency: The new method significantly reduces the computational cost.
- Broad Applicability: The technique can be applied to a wide range of problems across various disciplines.
- Potential for Innovation: The findings could pave the way for new discoveries and technologies.
The Future of Diffusion Modeling: A New Era of Precision
This research marks a significant step forward in our ability to model and understand complex diffusion processes. By combining mathematical innovation with computational efficiency, these new methods empower scientists and engineers to tackle real-world problems with greater accuracy and speed. From optimizing drug delivery to predicting environmental changes, the implications of this breakthrough are far-reaching and promise to shape the future of countless industries.