Unlock the Power of Moving Averages: A Beginner's Guide to Exponentially Weighted Models
"Discover how exponentially weighted moving models (EWMM) can revolutionize your data analysis and forecasting techniques."
In today's data-rich environment, the ability to extract meaningful insights from time-varying data is more critical than ever. Traditional methods often struggle to adapt to the dynamic nature of real-world data, where recent observations may hold more relevance than older ones. This is where exponentially weighted moving models (EWMM) come into play, offering a powerful and flexible approach to time series analysis.
At its core, an EWMM is a statistical method designed to fit a new data model for each time period, placing greater emphasis on recent data points while gradually diminishing the influence of past observations. This is achieved through an exponentially fading loss function, ensuring that the model remains responsive to changes in the underlying data patterns. The exponentially weighted moving average (EWMA), a widely used technique for estimating the mean using a square loss function, is a specific instance of EWMM.
This article delves into the world of EWMMs, exploring their underlying principles, advantages, and practical applications. Whether you're a seasoned data scientist or just starting your journey in data analysis, understanding EWMMs can significantly enhance your ability to model and forecast time series data effectively.
What Makes Exponentially Weighted Moving Models (EWMM) Different?
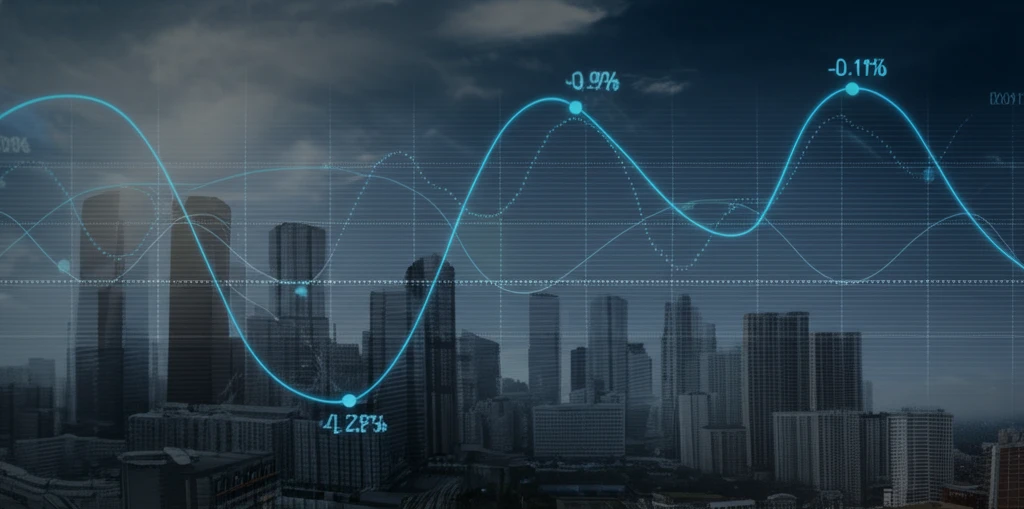
Unlike traditional moving average techniques that treat all data points within a fixed window equally, EWMM assigns weights that decrease exponentially as data points become older. This exponential weighting scheme offers several key advantages:
- Adaptability: EWMMs are highly responsive to changes in the underlying data, quickly adjusting to new trends and patterns.
- Flexibility: EWMMs can be applied to a wide range of data models and loss functions, making them versatile for various applications.
- Efficiency: For quadratic loss functions, EWMMs can be computed recursively, requiring minimal storage and computational effort.
Embracing the Future with EWMM
Exponentially weighted moving models offer a powerful and flexible approach to time series analysis, providing a means to adapt to changing data patterns and make informed decisions in dynamic environments. As data continues to grow in volume and complexity, mastering techniques like EWMM will become increasingly valuable for anyone seeking to extract meaningful insights from time-varying data.