Unlock the Power of Derivatives: How a New Mathematical Tool Could Reshape Probability and Statistics
"Discover a revolutionary 'natural derivative' that bridges the gap between continuous and discrete mathematics, offering new insights into binomial measures and beyond."
For years, mathematicians and statisticians have grappled with a fundamental challenge: the divide between continuous and discrete spaces. Many powerful tools in functional analysis work seamlessly in the realm of real numbers, but become far more complex when dealing with integers. One key reason for this disparity lies in the concept of a derivative. In continuous spaces, the derivative is a well-defined and ubiquitous tool. However, in discrete spaces, the notion of a derivative becomes less clear. How do you measure the rate of change when you can only move in whole number steps?
Traditionally, mathematicians have relied on finite difference operators to approximate derivatives in discrete settings. These operators come in two main flavors: left differences and right differences. The left difference looks backward, calculating the change between a point and the point immediately before it. The right difference looks forward, calculating the change between a point and the point immediately after it. While useful, these approaches have limitations, especially when trying to mirror the elegant properties of continuous derivatives.
Now, a groundbreaking paper introduces a new player in this field: a 'natural derivative' specifically designed for functions defined on the discrete interval [0, n]. This innovative operator, denoted as Vn, cleverly combines left and right finite differences, creating a hybrid approach that captures the essence of differentiation in a discrete world. This isn't just an academic exercise; this new derivative promises to unlock new insights into probability measures, spectral analysis, and even the optimization of complex systems.
What Makes This 'Natural Derivative' So Special?
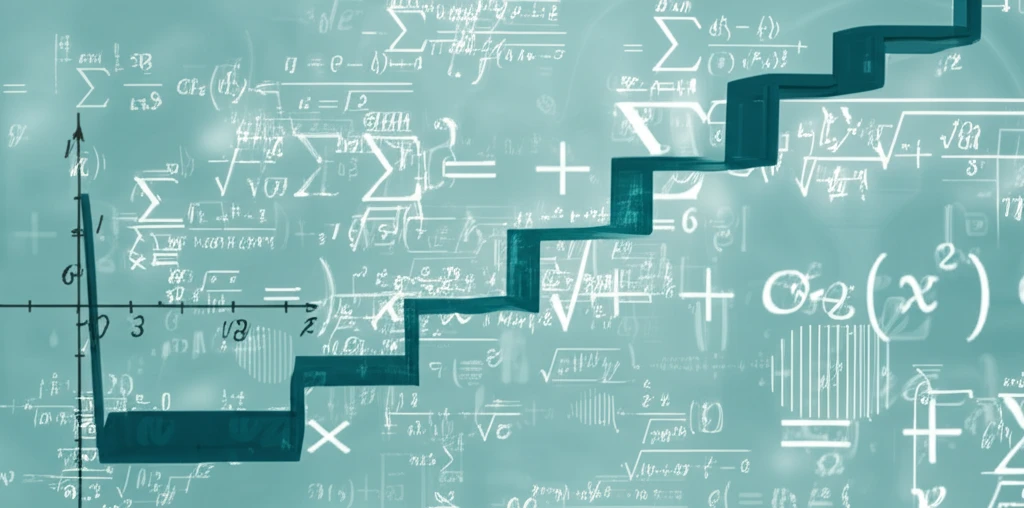
The key innovation lies in the way Vn blends left and right differences. Instead of relying solely on one direction, it takes a weighted average of both. This weighting is crucial, and it's defined in a very particular way: Vn is defined as a linear combination of left and right finite differences. This carefully constructed combination allows Vn to act as a bridge between the discrete and continuous, inheriting desirable properties from both worlds.
- A Smooth Transition: Vn facilitates a smoother translation of point masses, offering a more nuanced way to shift probability distributions across the discrete space.
- Poincaré Inequality: Vn plays a central role in establishing a new Poincaré inequality specifically tailored for binomial weights. This inequality provides powerful bounds on the variance of functions, offering insights into the concentration of probability measures.
- Spectral Analysis: The operator Vn possesses a remarkable connection to Krawtchouk polynomials, a family of orthogonal polynomials that arise frequently in probability and combinatorics. These polynomials act as eigenfunctions of Vn, allowing mathematicians to analyze the spectrum of the operator and gain deeper insights into its properties.
- Optimal Transport: The development of Vn may provide a means of tackling optimal transport challenges by developing effective computational techniques.
The Future of Discrete Calculus
The introduction of the 'natural derivative' Vn marks a significant step forward in our understanding of discrete mathematics and its connection to probability and statistics. While the initial paper lays a solid foundation, many questions remain. What are the full implications of this new operator? Can it be generalized to other discrete spaces? How can it be used to solve real-world problems in areas like machine learning, data analysis, and network science? Only time will tell, but one thing is clear: the future of discrete calculus looks brighter than ever before.