Unlock Super-Resolution: How AI Image Enhancement Sharpens Your World
"Dive into the fascinating realm of AI-driven image enhancement and discover how super-resolution technology transforms blurry images into crystal-clear masterpieces."
In an increasingly visual world, the quality of our images matters more than ever. Whether it's capturing precious memories, showcasing products online, or creating compelling marketing materials, clarity and detail are essential. But what happens when your images are blurry, pixelated, or simply lack the sharpness you desire? That's where the magic of AI image enhancement and super-resolution comes into play.
Imagine transforming a low-resolution snapshot into a vibrant, high-definition masterpiece. Or enhancing the details of a surveillance video to reveal crucial information. Thanks to advancements in artificial intelligence, these once-impossible feats are now a reality. Super-resolution technology, powered by sophisticated algorithms, is revolutionizing the way we perceive and interact with images in the digital age.
This article delves into the fascinating world of AI image enhancement and super-resolution, exploring the science behind these technologies, their diverse applications, and the potential they hold for the future. We'll uncover how AI algorithms analyze and reconstruct images, adding detail where none existed before, and examine the techniques used to overcome common image quality challenges like blur, noise, and aliasing.
The Science of Sharpness: AI Image Enhancement Explained
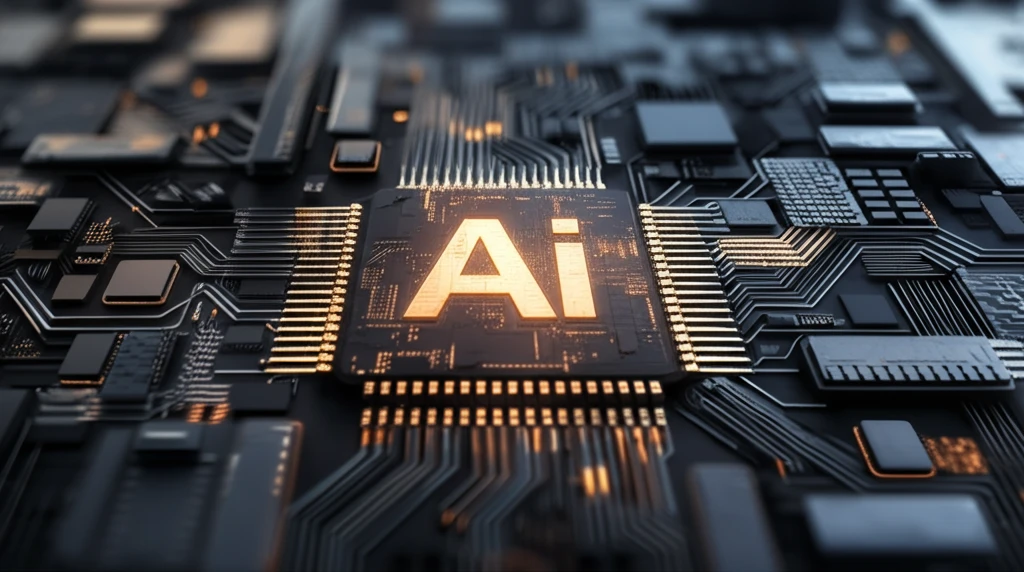
At its core, AI image enhancement leverages the power of machine learning to 'learn' the characteristics of high-quality images. By training on vast datasets of images, AI algorithms can identify patterns, textures, and structures that define sharpness and detail. These algorithms then use this knowledge to intelligently reconstruct low-resolution images, effectively 'hallucinating' the missing details to create a higher-resolution output.
- Internal Learning: Uses information already in the low-resolution image to find similar patterns at different scales.
- Cross-Scale Self-Similarity: Recognizes that small parts of natural images often repeat at different sizes.
- Adaptive Filtering: Selects the best filters to use based on the image's content, balancing detail and reducing unwanted artifacts.
The Future of Image Perfection: AI's Expanding Role
As AI technology continues to evolve, we can expect even more impressive advancements in image enhancement and super-resolution. Future algorithms will likely be more sophisticated, capable of handling a wider range of image types and overcoming current limitations. We may also see the integration of AI image enhancement into more consumer devices and software applications, making it easier than ever to transform ordinary images into extraordinary visuals.