Unlock Predictive Power: How Ensemble Machine Learning Can Boost Your Business Insights
"Combine multiple machine learning methods for better forecasts and reduced risk in data analysis."
In the world of data analytics, making accurate predictions is crucial. Businesses are constantly seeking ways to use data to forecast trends, understand customer behavior, and make informed decisions. However, with so many machine learning models available, it's hard to know which one to use or how to combine them effectively.
That's where ensemble machine learning comes in. Ensemble methods combine the predictions of multiple models to achieve higher accuracy and reduce the risk of overfitting, which is when a model performs well on training data but poorly on new, unseen data. This approach not only improves the reliability of predictions but also provides a more robust way to handle complex datasets.
This article explores the power of ensemble machine learning and how it can be applied across various industries to gain better insights and make smarter decisions. We'll break down the key concepts, discuss practical applications, and provide actionable strategies for leveraging ensemble methods in your own data analysis projects.
Why One Model Isn't Enough: The Challenge of Predictive Modeling
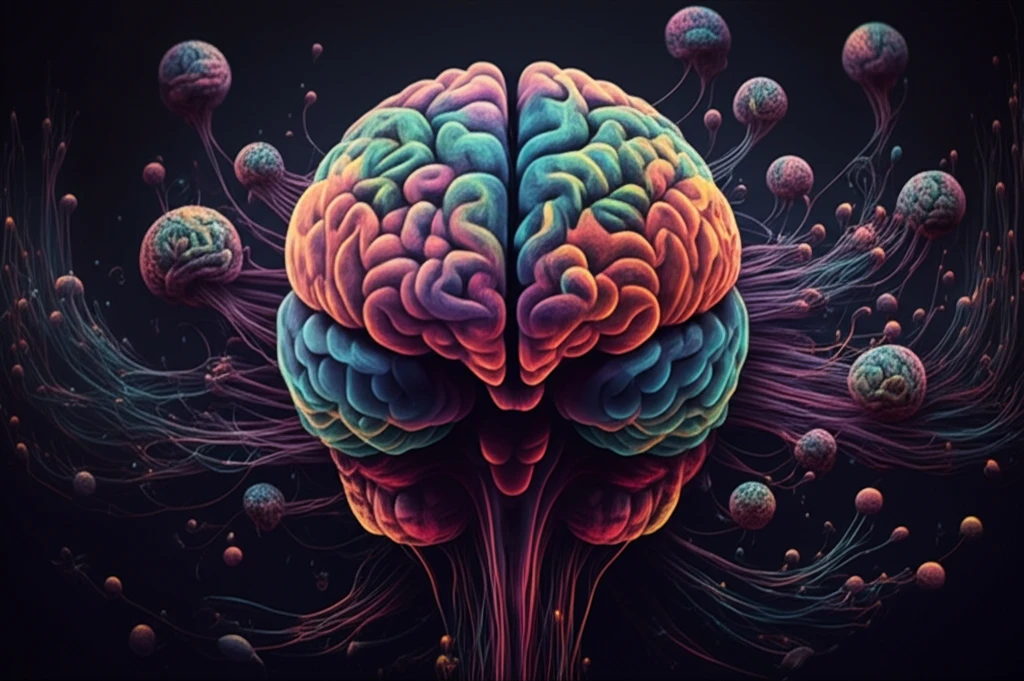
Predictive modeling is plagued by two persistent problems. First, with numerous available algorithms, determining the most suitable one for a specific problem can be daunting. Each algorithm has its strengths and weaknesses, and what works well in one scenario might fail in another. Comparative analyses often yield conflicting conclusions, adding to the confusion.
- Algorithm Selection: The sheer number of algorithms makes it hard to pick the best one.
- Overfitting: Models can learn training data too well, hurting their ability to predict new data.
- Tuning Parameters: Different settings can significantly change how well a model works.
The Future of Prediction: Embracing Ensemble Methods
Ensemble machine learning offers a pragmatic and effective way to improve predictive accuracy and reduce the risks associated with relying on single algorithms. By combining multiple models, businesses can gain more reliable insights, make smarter decisions, and stay ahead in an increasingly data-driven world. Embracing ensemble methods isn't just about using advanced technology; it's about adopting a more robust and adaptable approach to data analysis that can handle the complexities of real-world problems.